Application of Sequential Design of Experiments (SDoE) to an MEA-based CO₂ Capture Process at TCM
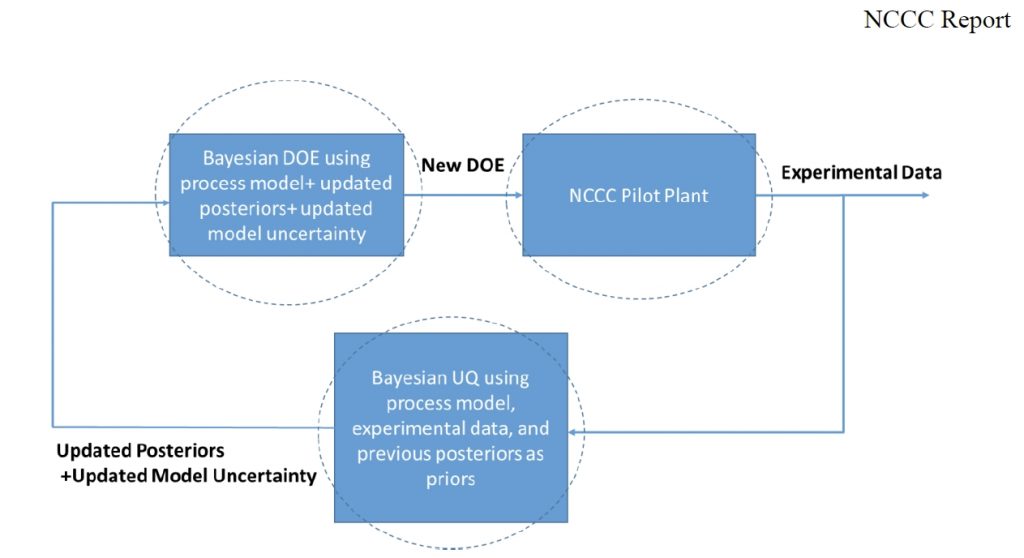
The U.S. Department of Energy’s Carbon Capture Simulation for Industry Impact (CCSI²) is a collaboration among national laboratories, academic institutions, and industrial partners focused on applying computational tools to accelerate and de-risk the development and commercialization of CO2 capture technology.
This suite of tools (CCSI Toolset: https://github.com/CCSI-Toolset) was developed under the CCSI² predecessor program, the Carbon Capture Simulation Initiative (CCSI).VendorCarbon Capture Simulation for Industry Impact (CCSI2)PartnersU.S. Department of Energy, TCMTest at TCMJune-July, 2018
Vendor:
Carbon Capture Simulation for Industry Impact (CCSI2)
Partners:
U.S. Department of Energy, TCM
Test at TCM:
June- July, 2018
The CCSI tools applied in the work with TCM are from a modelling framework for solvent-based CO2 capture applications which quantify the effect of parametric uncertainty on user-defined performance metrics. The goal of the CCSI² work at TCM is to apply an aqueous MEA model integrated with this Uncertainty Quantification (UQ) framework to develop high impact test campaign results which maximize learning with each test run. CCSI² has developed a sequential design of experiments (SDoE) methodology which leverages UQ through machine learning to intelligently target TCM test runs to systematically improve model precision.
In this approach, a subset of the underlying parameters is characterized by probability distributions, representing uncertainty in the parameter values. Parameter distributions and their effects are propagated through the process model, resulting in uncertainty distributions of key output variables of interest. The SDoE approach is flexible enough to adapt to different study criteria of interest. For the TCM campaign, the focus was on selecting test conditions for which the estimated output uncertainty is large in order to improve knowledge in these regions. At the same time, SDoE spreads test points throughout the input space to ensure full characterization of performance throughout all regions of interest. Data are incorporated into a Bayesian inference framework which updates the model parameter distributions as information is gained throughout the campaign.
The output uncertainty for the input space of interest is updated simultaneously with the model parameter distributions, thus resulting in an iterative process which systematically refines the models and improves the test plan as the campaign progresses. The CCSI2 team has implemented this methodology while collecting data at TCM, resulting in an average (across the entire input space) reduction in estimated CO2 capture rate uncertainty of about 60%; capture predictions improved from +/- ~10% to +/- ~4% across the operating range studied. In addition to the SDoE procedure, additional plant data have been gathered to support optimization of an economic objective function under uncertainty