Development of CO2 capture process cost baseline for 555 MWe NGCC power plant using standard MEA solution (2022)
Koteswara Rao Puttaa*, Daniel Saldanab, Matthew Campbella, Muhammad Ismail Shaha
aTechnology Centre Mongstad, 5954 Mongstad, Norway
bAspen Technology, Inc, 01730 Bedford, Massachusetts, USA
Abstract
Carbon capture, utilization and storage (CCUS) is essential to achieve Net-zero emissions targets. The IEA sustainable development scenarios also emphasize the importance of CCUS. Post-combustion CO2 capture using amine solvents is the most mature technology among several options available and amine-based CO2 capture projects have been demonstrated at industrial scale. Several new vendors and technology developers are working on multiple innovative and advanced CO2 capture concepts. Industrial clients, project developers targeting the CO2 capture projects in their facilities require reliable and updated costing information using non-proprietary solvents to develop investment strategies, portfolios and evaluate the commercial project bids for CO2 capture.
The CO2 project cost estimation depends on several factors like solvent used, amount of flue gas treated, accuracy of the simulation tool/model used for designing the CO2 capture plant, quality and size of experimental pilot data used for model validation, accurate representation of the capture facility while keeping the columns hydraulics suitable for practical operation, consideration of space requirements for column internals, design of plate heat exchangers and other packaged items like filter package and reclaiming units. Domain knowledge and practical operational experience are also crucial to perform the study. Selection of appropriate material of construction also plays a key role in accuracy of cost estimation. Technology Centre Mongstad’s 10 years of operational knowledge and experience together with AspenTech’s expert team worked together to perform a reliable and accurate costing exercise by considering all essential elements of CO2 capture process and project. The key finding from the current costing baseline study are columns material costs found to account for 63% of total CO2 capture process equipment material costs and absorber alone accounts for 45% of these total equipment material costs. The total capital expenditure for capturing 90% CO2 from 555 MWe Natural Gas Combined Cycle (NGCC) power plant using aq. 30 wt% MEA solvent is estimated to be around 326.6 Million USD. Annual total operating costs are estimated to be 47 Million USD. Assuming 25 years of plant life, the cost of CO2 capture is calculated to be 47 USD/ton.
1. Introduction
The CO2 Technology Centre Mongstad (TCM) is located next to the Equinor Mongstad refinery in Norway. TCM DA is a joint venture owned by Gassnova representing the Norwegian state, Equinor, Shell, and TotalEnergies. TCM is the largest post-combustion CO2 capture (PCC) test centers in the world. This facility has been in the operation from 2012 and is often called the “amine plant”.
TCM amine plant was built to run on two different types of flue gases, namely, natural gas-fired combined heat and power (CHP) plant i.e., CHP flue gas is from a natural gas fired CCGT plant or the Equinor Mongstad refinery residue fluid catalytic cracker (RFCC). Over the last 10 years, several commercial vendor proprietary solvents as well as non-proprietary solvents have been tested at TCM amine facility. TCM amine plant is well equipped with around 4,000 online sensors to gather critical information in addition to more than 100 offline manual sampling points. TCM has more than 20,000 hours non-proprietary operational data using aqueous monoethanolamine and CESAR 1 (AMP/PZ mixture) [1–7]. Fig. 1 shows the process flow diagram for CO2 capture process at TCM.
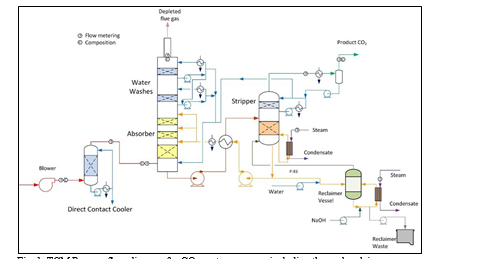
A comprehensive baseline for performance and cost estimation of CO2 capture processes is crucial to assess, identify and support widespread deployment of CO2 capture projects. Successful CCUS (Carbon Capture Utilization and Storage) projects will be essential for achieving world Net-Zero emissions targets by 2040-2050. To have a fair assessment of new technology developments and avoid using commercial technology as baseline, TCM has developed a cost baseline using a non-proprietary solvent, i.e., aqueous 30 wt% Monoethanolamine (aq. MEA) solution. The assessment of performance and cost were determined independently and does not represent the views of any technology vendor. The baseline input conditions for this model and costing exercise were based on National Energy Technology Laboratory (NETL) baseline study case 14 [8] with CO2 capture.
The present work discusses about the systematic methodology or steps followed to develop a technoeconomic analysis (TEA) for CO2 capture technology using AspenTech products like Aspen Plus, Aspen EDR, Aspen Capital Cost Estimator (ACCE). A proper costing baseline development must contain five critical steps, given below:
Step 1: Develop and validate an accurate rate-based process model with operational data from pilot plant Step 2: Run steady state simulation with aqueous 30 wt% MEA solvent targeting 90% CO2 capture from the industrial plant of interest and capacity
Step 3: Perform detailed sizing of major process equipment such as columns (direct contact cooler, absorber, regenerator, water-wash sections) and heat exchangers, pumps, blower/compressors …etc.
Step 4: Perform estimation of costs using vendor quotes and/or relevant costing software tools by considering all relevant elements
Step 5: Economic/financial analysis and presentation of results and sensitivity study
These steps are described in detail in the following sections 2 and 3.
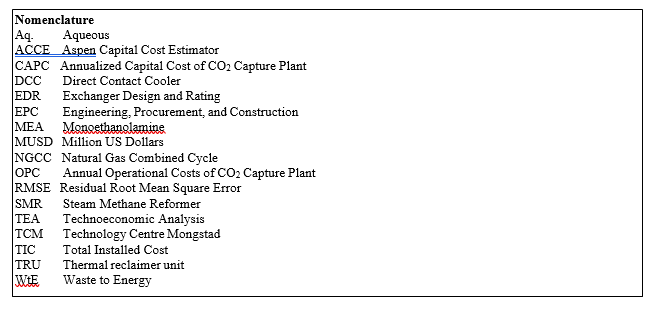
2. Methodology
As mentioned above, a proper technoeconomic analysis for CO2 capture technology using alkanolamines requires five essential steps explained below.
In the present work, CO2 capture using aqueous MEA solution has been considered and AspenTech software tools are used to perform different steps involved in the TEA. In this section, Steps 1- 4 will be described in detail and step 5 will be presented in section 3.
2.2 Step 1 – Development and validation of process model
In order to develop the process model, it is important to understand the chemicals used, chemistry involved and phenomena occurring in the process. Thermodynamics plays key role in CO2 absorption process design and simulation as the absorption with chemical solvents requires thermodynamic data, especially phase equilibria: vapor-liquid equilibrium (VLE) and speciation in the solution. Aqueous amine systems used for CO2 capture are non-ideal systems which can be thermodynamically modelled utilizing activity coefficients models for the liquid phase such as Electrolyte NRTL (e-NRTL), Extended-UNIQUAC or similar. Gas phase is modelled by utilizing equation of state such as Redlich-Kwong (RK) or Soave-Redlich-Kwong (SRK). Aspen Plus V11 software is used to develop the model and validate.
In the current study, MEA is the main chemical used as solvent to capture the CO2 from flue gas. In the present study, e-NRTL RK method has been chosen for thermodynamic modelling. The chemistry/ chemical equilibrium involved in the MEA-CO2-H2O system are given by the reactions (1)-(5) below [9,10].
Ionization of water:
2H2O ↔ OH− + H3O+ (1)
Dissociation of carbon dioxide:
2H2O + CO2 ↔ H3O+ + HCO3− (2)
Dissociation of bicarbonate:
H2O + HCO3− ↔ H3O+ + CO32− (3)
Dissociation of protonated amine:
MEAH+ + H2O ↔ H3O+ + MEA (4)
Carbamate reversion to bicarbonate:
𝑀𝐸𝐴𝐶𝑂𝑂− + 𝐻2𝑂 ↔ 𝑀𝐸𝐴 + 𝐻𝐶𝑂3− (5)
In the literature, simulation or design of columns is performed by either using equilibrium-based approach or rate- based modelling approach. For CO2 capture applications with amine solvents, rate-based modelling approach gives a more realistic and predictive model compared to equilibrium-based approach. Rate-based modelling approach has been used for all columns in the CO2 capture.
In addition to the reactions (1)-(5) considered in equilibrium thermodynamic model above, kinetic reactions are also important to accurately design and simulate the process, especially absorber column. Two reactions have been considered to be kinetically controlling, given below [9,11,10]:
𝐶𝑂2 + 𝑂𝐻− ↔ 𝐻𝐶𝑂3− (6)
𝐶𝑂2 + 𝑀𝐸𝐴 + 𝐻2𝑂 ↔ 𝑀𝐸𝐴𝐶𝑂𝑂− + 𝐻3𝑂+ (7)
Power law expressions are used for the rate-controlled reactions (reactions (6)- (7)) on activities basis. The power law expression (8) is used for reaction rate:
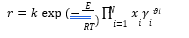
Where 𝑟 represents reaction rate; 𝑘 is pre-exponential factor; 𝐸 is activation energy; 𝑅 is Universal gas constant; 𝑥𝑖 is mole fraction of component i; 𝛾𝑖 is activity coefficient of component i; 𝜗𝑖 is the stoichiometric coefficient of component i in the reaction equation and T is temperature. Kinetic reaction parameters are regressed using literature kinetic data.
After the development of the e-NRTL RK thermodynamic model for MEA-CO2-H2O system and kinetic parameters refinement to match literature data, this model is validated with TCM testing pilot scale data. This validation has been performed by implementing TCM amine plant in Aspen Plus and collecting the steady state operational data over wide range of conditions. The operating conditions window used for the model validation is given in Table 1. The criteria for selection of steady state conditions employed in model validation are as follows:
- Overall plant mass balance is 100 +/- 2%
- CO2 mass balance 98 +/- 2%
- Stable operation at least 2 – 6 hours (steady state operation)
- Availability of liquid lab analysis results for CO2 and amine concentrations
Table 1 TCM Aspen Plus model validation conditions range for CO2 capture using aq. MEA solution
Operating parameter | units | Range |
Flue gas flowrate | Sm3/hr | 34,000 – 68,000 |
Flue gas CO2 concentration | vol % | 3.6 – 14 |
Flue gas temperature | oC | 28 – 45 |
MEA concentration | wt % | 28 – 40 |
Lean amine loading | mol/mol | 0.1 – 0.3 |
Lean amine temperature | oC | 35 – 50 |
CO2 capture rate | % | 70 – 99 |
Absorber packing height | m | 12,18, 24 |
Water wash sections | # Sections | 1, 2 |
Stripper in operation | – | CHP, RFCC† |
† The difference between CHP and RFCC strippers is in the diameter. CHP stripper ID=1.25 m and RFCC stripper ID=2.2 m. Both strippers are The model validation results are shown in Fig. 2. As it can be seen from the Fig. 2, the model predicts the pilot scale data with good accuracy both for CO2 capture as well as specific reboiler duty (SRD). The outliers or higher deviation in the SRD corresponds to cases where maldistribution and foaming were occurring in the stripper during operation.
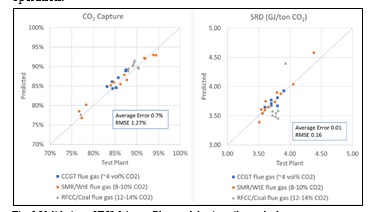
After extensive validation with TCM scale pilot data and proven model predictability over wide range of operation window, the TCM Aspen plus model is used for simulating and designing real industrial scale plants.
2.2 Step 2 – Simulation of CO2 capture from NGCC power plant
The present study is to capture CO2 from 555 MWe Natural Gas Combined Cycle (NGCC) power plant. Aqueous 30 wt% MEA solution is used as solvent for capturing CO2. Steady state simulation with aqueous 30 wt% MEA solvent targeting 90% CO2 capture from 555 MWe‡ Natural Gas Combined Cycle (NGCC) power plant. This was based on the case 14 from NETL baseline reference [8] performed with MEA solvent. The flue gas conditions are given in Table 2.
The validated model described in section 2.1 using Aspen plus is used to simulate the design case. The process description for CO2 capture is given in the following paragraphs.
Table 2 555 MWe NGCC plant flue gas conditions for CO2 capture
Name | Value | Units |
Flow rate | 113,831 (3,230,636) | kgmol/hr (kg/hr) |
T | 143 | °C |
P | 0.1 | MPa, abs |
Annual operation | 8000 | hours |
Capture rate | 90 | % |
Annual CO2 capture | 1,475, 200 | ton/year |
Composition | Mole fraction | |
Ar | 0.0089 | |
CO2 | 0.0404 | |
H2O | 0.0867 | |
N2 | 0.7432 | |
O2 | 0.1209 | |
NOX | 155 | ton/year |
Flue gas from the NGCC power plant is introduced in direct contact cooler (DCC) to cool it to the required absorber inlet temperature. In the DCC cold water is feed at the top and hot flue gas (143°C) is introduced at the bottom of the DCC column. The flue gas moves counter-current to the cold water over a packed section and gets cooled to the desired temperature and leaves to the absorber via a flue gas blower. The flue gas blower supplies enough pressure to the flue gas to overcome the pressure drop in the absorber. In the DCC, when the incoming flue gas is cooled by contacting with the cold water, water vapor in the flue gas gets condensed. The excess water condensed from flue gas will be bled and the remaining water will be cooled in the DCC water cooler (DCCHEX) and sent back to the DCC top. The flue gas cooling process implementation in Aspen Plus is shown in Fig. 3.
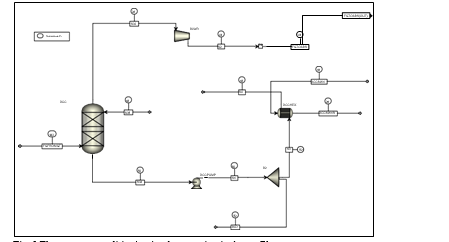
The pre-conditioned flue gas from DCC is fed to the absorber bottom where it meets counter-currently the lean amine fed at the top of absorption section. CO2 is captured by amine via an exothermic reaction forming a carbamate as MEA is primary amine. The lean amine becomes richer and richer as it flows down the absorber column and leaves the absorber bottom via rich amine pump to the stripper via rich/lean cross plate heat exchanger. The flue gas becomes depleted in CO2 on its way upward in the absorber column, due to exothermic reactions in the absorber, the flue gas and amine solvent temperature increases. The NGCC flue gas leaving the absorption section is normally saturated with water and containing volatile components like solvent amine and other amine degradation products. To reduce loss of amine and reduce emission of volatile components such that the plant can be operated within regulated emission permit, the flue gas from the absorption section needs to be cooled and conditioned water washes sections above of the absorber section before sending it to the atmosphere via flue gas stack. In the water wash sections, the flue gas is cooled by counter current flow of cold water, which partially condenses water and some of the volatile components. The condensed stream is fed back either to the bottom of the absorber or can be fed with lean amine feed to the absorber to keep the plant in water balance and reduce the amine loss.
In the stripper the CO2-rich amine solution flows downwards during which CO2 is stripped off from the rich solution by supplying heat in a reboiler with low pressure steam. The CO2-rich gas stream containing CO2, steam, and solvent vapor from the top of the stripper is partially condensed in the overhead condenser and separated from the gas stream in the gas-liquid separator. The condensed liquid is sent to the stripper using reflux pump. The top section of the stripper is a wash/rectification section designed to limit the amount of solvent vapor entering the stripper overhead system.
The stripped solvent from the bottom of the stripper, i.e., CO2-lean solution from the bottom of the stripper is cooled-down in the lean/rich cross heat exchanger after heat exchange against the rich solvent; further cooled in the lean amine cooler to achieve the desired temperature for the optimal operation of the absorber. However, solid particles from the feed flue gas could get into the solvent and cause foaming during operation. A split stream of about 5-10% of the circulating solvent is passed through a filtration unit consisting of a mechanical pre-filter, activated carbon bed and post-filter, to remove solid particles and substances that could increase foaming in the solvent. Aspen plus V12 implementation of CO2 capture process is shown in Fig. 4.
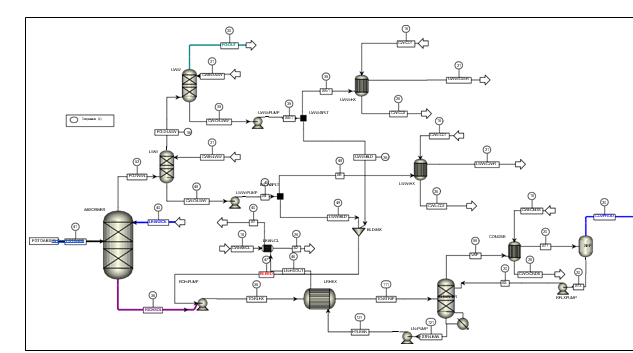
Amine based solvents will degrade over time, the rate of degradation is depending on the solvent molecular stability, flue gas contaminants more specifically NO2, SO2 and O2, operating conditions and plant design (hot inventory). The level and composition of corrosion and solvent degradation products also affect the degradation rate. Capture, HSE and energy performance of the solvent will deteriorate over time unless the solvent is kept in good hygiene to reduce the degradation. One way of keeping the solvent is reclaiming, where a slip stream (normally a slip from hot lean amine stream downstream lean amine pump) is reclaimed in a thermal reclaimer unit (TRU). Reclaiming is performed at higher temperatures than stripper (130-150°C for MEA). Caustic soda (NaOH) is added to the recycle stream to neutralize the acids and liberate MEA. The amount of caustic to inject can be calculated based on the amounts of organic acids (formic, glycolic, oxalic, and acetic), nitrate, nitrite, and sulfate in the solvent. Due to high temperature, water and major portion of the non-degraded amine are evaporated and fed back to the stripper via top of the TRU. The bottom of the TRU contains heavy molecules, some solvent amine and most of the degradation products. These bottoms products are more viscous than solvent amine and are pumped out and disposed as hazardous waste depending on the composition. The reclaiming section schematic and implementation in Aspen Plus is shown in Fig. 5.
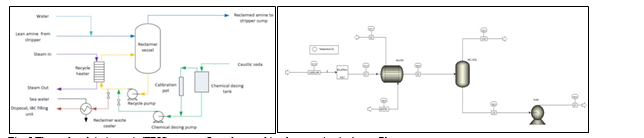
2.3 Step 3 – Equipment sizing
After simulation the CO2 capture unit, the next step is to perform sizing of all essential equipment. This sizing information will be utilized in the next cost estimation step. This section provides some key information and tools
used to perform sizing of different equipment.
- Columns: As Aspen Plus rate-based model is used to perform the simulation in Step 2 above, diameter and packing height is calculated from the Aspen Plus for the selected packing by maintaining good hydrodynamics and meeting the design requirements. In the costing, as the tangent-to-tangent information needs to be provided to get accurate/reliable price for the columns, the spacing required for the internals (e.g., demisters, liquid collectors and distributors, packing supports, sump … etc.) in the columns was calculated based on TCM amine plant columns.
- Plate heat exchangers: The process information from the Aspen Plus simulation is transferred to the Aspen Exchanger Design and Rating (EDR) V12 software and exchanger sizing was performed using the EDR tool to meet the required heat transfer duties and achieve corresponding temperatures for the process/utility streams. The plate types in the plate heat exchangers are selected based on the TCM internal datasheets from the amine plant.
- Reboiler: Kettle type reboilers were designed in the EDR software using the solvent and steam information from the Aspen Plus simulation.
- Separators/vessels: Gas -liquid Separators, reclaimer vessel sizing was performed using Aspen Plus sizing feature.
- Storage tanks: Storage tanks sizes for solvent storage, Caustic storage, reclaimer waste storage was estimated from volume of liquids/solvents from process simulation as well as process operating experience from TCM.
- Cooling towers: Cooling duties and cooling water flowrate were taken from the Aspen Plus simulation performed in step 2. Using this information and cooling towers standards, cooling tower units sizing was considered by discussing with experts working in the industry.
- Filtration unit: Activated carbon filter package sizing was performed assuming that a slip stream of lean amine solvent, i.e., 10% of lean solvent will be sent to filter package.
In addition to the AspenTech softwares, TCM internal tool for reclaimer and TCM internal database have been used in the present study.
2.4 Step 4 – Cost Estimation
This section provides information and methodology followed to estimate the costing using Aspen Capital Cost Estimator (ACCE) V12 software. Step 4 has been performed together with experts from AspenTech. The cost estimations in this work represent the costs that are relative to new plants without considering specific project requirements for design, standards or site integration
AspenTech’s Economic Evaluation solution is based on a core design, estimating, scheduling, and expert systems technology. It automatically develops preliminary design-based economic results – early from minimal scope, and refined designs and economics later in the project. This unique technology provides:
- Key answers quickly
- Dramatic reductions in evaluation time and resources
- The best, most economical process and plant design for funding/bidding decisions and project evaluation.
Aspen economic evaluation systems (including Aspen Capital Cost Estimator and Aspen Process Economic Analyzer) are in daily use. Aspen Capital Cost Estimator uses the equipment models contained in the Evaluation Engine – a knowledge base of design, cost, and scheduling data, methods, and models – to generate preliminary equipment designs and simulate vendor-costing procedures to develop detailed Engineering-Procurement- Construction (EPC) estimates. Volumetric models generate a costed, quantity takeoff for the bulk materials without using factors or user input. The volumetric models also produce the quantities of pipe, valves, concrete, steel, and instruments identified by the associated equipment or area. Components of each line of pipe and instrument loop are quantified and costed, enabling the user to view and adjust construction tasks. The Aspen Capital Cost Estimator Work Item Models produce the required man-hours by craft and task needed to install Aspen Capital Cost Estimator- generated bulks, as well as the equipment Aspen Capital Cost Estimator designed, by simulating detailed design construction tasks. Finally, the Engineering Models in Aspen Capital Cost Estimator produce man-hours by discipline and engineering work product.
Cost indices in the economic evaluation suite and Aspen Capital Cost Estimator include engineering disciplines, wage rates, material costs, shop and field labor rates, construction equipment rental rates, etc. These indices do not derive from public sources, and they may not accurately reflect how they affect a specific project. To evaluate this, the user should run benchmark projects and develop own adjustments.
2.4.1 Aspen Capital Cost Estimator Project General Workflow
- Create project scenario and define properties like country base, units of measure, and currency.
- Define design basis (general mechanical design rules), wage rates and productivities, code of account definition and allocation, material and man-hour indexing, equipment rental, and project execution schedule settings.
- Define the Power Distribution system (if desired).
- Define the Process Control system (if desired) and link to areas and substations.
- Add contractors and redefine responsibilities (if desired) and link to areas and substations.
- Run an item evaluation to produce direct costs for an individual component or run a project evaluation to produce design and cost results needed to prepare project reports.
- View and/or print reports.
2.4.2 Generating the economic evaluation for this project
The economic evaluation project with the equipment list can be generated in simulation either within the simulation itself or by importing the simulation file into ASPEN CAPITAL COST ESTIMATOR. The economic evaluation can be started from within the simulation, by setting the economics active in the economic evaluation tab, as shown in Fig. 6.
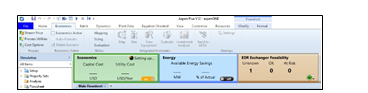
The simulation will generate a preliminary estimate based on default values; however, these values should be reviewed by the user. The first thing to review will be the mapping process.
Simulations and estimates differ in the sense that a theoretical unit operation is not the same as pieces of equipment set on a plant. For example, a column unit operation may converge for heat and mass balance, but when estimating it, an actual distillation process needs the column vessel, the reboilers, the condensers and accumulators as well as the pumps to circulate the condensate. Furthermore, mapping is important because while a heat exchanger may be represented by a simple unit operation, for estimation it will be very important to define if the type is an air cooler, shell and tube exchanger or others. Lastly, the mapping exercise will also have an impact on the bulks cost calculated by the economic evaluation engine for example a separator in a simulation may represent a process vessel or a storage tank and these will have greatly different impact in the piping and instrumentation associated to each of them as shown in Fig.7.
As the Fig.7 illustrates below, a horizontal process vessel needs more piping and instrumentation items than a general storage tank would. This has an impact in the final estimated project cost.
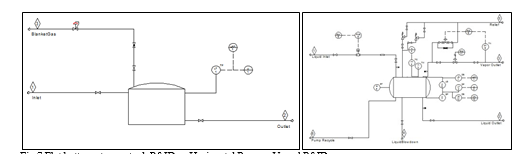
Once the mapping exercise is done the, the economic evaluation technology integrated in the simulation will perform a sizing of the equipment list generated in the previous list. This sizing considers the mass and energy balances calculated in the simulation and sizes the equipment following standards defined in the design bases under “cost options”. Design Basis defines the general mechanical design rules for the entire project. AspenTech’s Economic Evaluation uses built in, industry-standard design procedures for the preparation of mechanical designs. The standards used include ASME (American Standards), BS5500 (British Standards), JIS (Japanese Standards), DIN (German Standards), or EN 13445 (European Standards).
Finally given the equipment list and design generated in the economic evaluation, a preliminary cost analysis will be generated for the equipment listed in the simulation which includes the material cost as well as the installed cost of the equipment list, it will also include a preliminary operational cost given the utilities defined in the simulation (ACCE has default utilities defined in case a user does not define them manually) and generates an “IZP” file that is readable by Aspen Capital Cost Estimator, AspenTech’s detailed estimating tool.
It is important to mention that an estimate generated from the simulation and the cost results displayed in the simulation are just a preliminary cost. For an asset’s total install cost (TIC) to be completely estimated, it still needs definitions of structures such as Pipe Racks, the Piping that will interconnect multiple areas, Control Centers, Power Distributions, any and all skids for the dosage packages etc. User input is required for labor wage rates, productivities, escalation indexes, utilities cost definitions, licensor and other indirect costs, contingency costs, etc. For this, previously generated IZP file is imported into Aspen Capital Cost Estimator and add more details to the existing estimate to create a higher quality estimate that can be backed up with better data.
Open the IZP file in Aspen Capital Cost Estimator and review the Project Basis. So far, default values have been used, however have more control within the Aspen Capital Cost Estimator User Interface to adjust and modify the cost and design basis to meet the needs.
In the Equipment Specs form under Design Basis, design information specific to the project needs to be entered, such as:
- Design allowances
- Rental equipment option for lifting
- Shop fabrication specifications
- Design cut off temperature
Notice default values are light blue colored while user entered values will be black as shown in Fig. 8.
Continuing under Project basis view, user will find General Piping Specs form. Enter here information such as Pipe fabrication preferences, tracing tube material, X-ray analysis percentage, insulation jacket material, etc.
As moving on to the next form Civil/Steel Specs will be displayed. This form will let the user enter information about plant site such as soil type, loading density, wind velocity, pile types and sizes. All this data is used to calculate the amount of civil work needed to setup the equipment, for example how much foundation the columns need as well as structural steel, all key parts of the direct cost estimated.
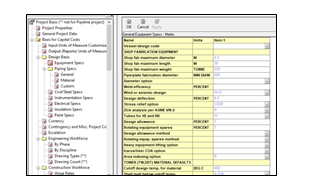
Continuing, next to the Instrumentation Specs Form. This form allows the user to enter information about the instrumentation configuration needed in the project, such as P&ID design, Instrumentation type and system, Junction Box distances and cable size option. Entering this information will affect the quantity of material needed for instrumentation, the type of material used and will directly affect the Instrumentation code of account direct cost. The most important modification done for this project will be the type of instrumentation, as the default Pipe and Instrumentation design (P&IDs) is changed from Standard Instrumentation to Fully Instrumented (FULL). One important point to consider here using the domain knowledge, even the fully instrumented option still doesn’t have all the required instrumentation for few equipment, such as absorber column which contains both absorption and water wash sections in the same column. User needs to add additional instrumentation required manually. This option is available in ACCE.
Wage Rates form is available under the Construction Workforce folder. This form shown in Fig. 9 allows to enter data for wage rates, productivities, shifts per workday, overtime wage rates. This information is used to calculate the total labor hours needed to install all equipment and bulks, reflected in the direct costs.
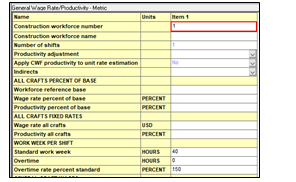
To add Indirect cost such as Developer Cost, Plant Integration and Start Up as a percentage of the EPC cost, user can add them to the “Indirect Prorate-able” table, in this table users are able to define costs as percentage basis of the EPC cost and can also organize said costs as direct costs by defining its “code of account” a code that allows for reporting purposes where should the cost be displayed. An example of how this table looks can be seen in Fig. 10.
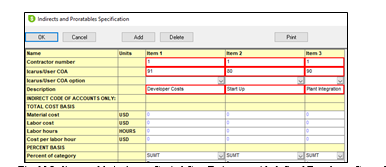
Moving into Project basis, there are other aspects of an EPC estimate that have been not considered so far. The Aspen Plus simulation link with Economic Evaluation gave us the major pieces of equipment present in the project, however items such as pipe racks, interconnecting piping with the outside battery limits, motor control centers, and even services such as dosage packages and cooling towers are not present in a normal simulation but are important to be considered when evaluating the capital cost of a project. Items can be added manually from the Aspen Capital Cost Estimator library of components as shown in Fig. 11.
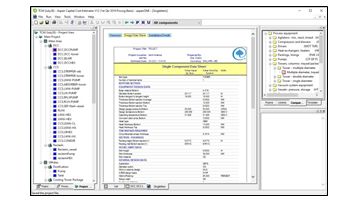
For items like the dosage packages, user can add them as vessels and pumps that are mounted on skids. ACCE allows us to add these skids and reuse them in future projects as new library items.
Once user has completed the scope of the direct costs entered in Project View and the Indirect Costs entered in Project Basis, user can evaluate the estimates to generate reports to give the CAPEX of the project.
To analyze the OPEX of the project is important to correctly define the Investment Parameters form for the asset. The Economic Evaluation soluition provides a standard form with data, but defining correctly the Economic Life of the Project, the number of years and how many weeks per year, will be important for this analysis. User should also define how many hours will the asset will be operating per year as shown in Fig. 12.
It is important to set-up operating unit costs, that allows to define operator and supervision costs that will be needed year over year to operate the asset/plant. Raw material cost is also necessary for this analysis, in this case, defining the cos of the MEA will be key. Lastly defining the utilities costs for steam, cooling water, electricity and intrumentation air will allow the software incorporate these costs into the Investment Analysis and provide a breakdown of the costs year over year of the asset.
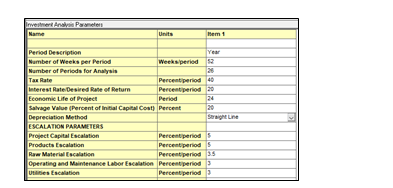
3. Results and Discussion
After finishing the step 4, the user needs to evaluate the project in ACCE to get costing report for the project. The user has the possibility to select interactive excel based report. ACCE will generate extensive report with 99 different types of summaries related to the project. In this section (step 5), the main focus will be on the results generated/estimated from the economic analysis to get insights like capital and operating costs and cost of CO2 capture per ton. The cost basis used for economic analysis in ACCE V12 is 2019 Q1. This is considered as base year for TCM costing estimations.
The Capital cost summary with various details, estimated by ACCE is shown in Fig. 13 below. The total capital cost (referred to as Project Total Costs in ACCE and Fig. 13) for capturing 90% CO2 from 555 MWe Natural Gas Combined Cycle (NGCC) power plant using aq. 30 wt% MEA solvent, estimated by ACCE is around 326.6 MUSD. The equipment cost (~105 MUSD) corresponds to 32% of Project Total Costs.
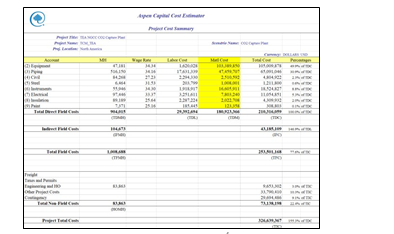
The direct and indirect field costs summary is shown in Fig. 14. Direct field costs contribute to 64% of total capital costs. Direct field costs include equipment, piping and instrumentation, civil and steel structures, insulation, and paint required. Indirect field costs include scaffolding, consumables like small tools and consumable materials, construction rental equipment costs, startup and commission, field supervision …etc. Non-field costs include engineering costs (basic, detailed engineering and materials procurement, contract fees, general, and administrative overheads, and contingency …etc.)
§ According to the current market data, the cost for plate heat exchangers is overestimated in ACCE V12. This can be modified by recalibrating the plate heat exchangers costs in ACCE using vendor quotes. This calibration was performed by using costing data from CO2 capture projects, calculating the over-estimation factor and indexing the data accordingly, matching market data.
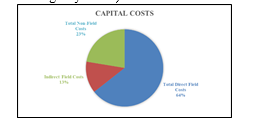
The materials cost summary of CO2 capture plant is shown in Fig. 15. Columns material costs account for 63% of total equipment material costs and absorber accounts for 45% of total equipment material costs.
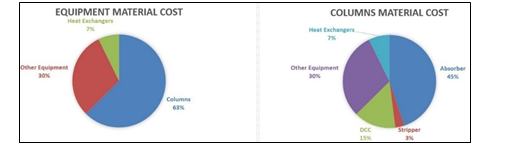
In order to understand and verify the accuracy of the cost estimates from the present work, a comparison has been made with NETL baseline study cost estimates [8]. In the NETL baseline study, flue gas pre-conditioning is not considered. Therefore, a comparison is made after excluding the flue gas pre-conditioning section (all associated equipment) which represent a reduction of 18% of the total equipment costs. The CAPEX for equipment (total field costs) estimated by ACCE is around 172.8 MUSD and prorating the NETL baseline costs using the chemical engineering plant cost index (CEPCI) estimated to be around 162.1 MUSD. This translates into 6.5% increase in costs, which is an acceptable deviation, as shown in Table 3.
Table 3 TCM Aspen and NETL baseline cost estimates comparison
Name | TCM | NETL |
Cost base | 2019 Q1 | 2007 June |
Capital costs excluding flue-gas pre-conditioning (direct), MUSD | 172.8 | 140.0 |
Prorated costs to TCM base year, MUSD | – | 162.1 |
Difference (%) | – | 6.5 |
Operating costs for the CO2 capture plant will include costs for manpower, utilities (cooling water, steam, process water), power and solvent makeup, other chemicals and waste handling costs. It is assumed that on annual basis, cooling water make-up will be 10% to compensate for bleed. Solvent costs will require an estimate of solvent lost due to degradation, evaporation losses as well as solvent lost in the waste during reclaiming. TCM internal tool for reclaiming has been used to estimate the annual total solvent makeup required. Solvent and reclaimer waste handling costs are taken from TCM internal quotes.
The total annual operating costs are estimated to be 47 MUSD. With MEA cost of 2000 USD/ton, the solvent makeup costs account for 15% of the total operating costs and these costs share increases to 20% with 3000 USD/ton MEA price. Solvent degradation is very crucial when it comes to optimizing the CO2 capture projects operating costs.
Cost of CO2 capture per ton:
Cost of CO2 capture per ton is calculated using the following equation (9) [12].

Where CAPCCCS represents annualized capital investment costs and OPCCCS represents annual operational costs of CO2 capture plant and TONCO2 represents tons of CO2 removed in a year.
With 25 years of plant life and 5% discount rate, the cost of CO2 capture per ton is calculated to be 47 USD and the cost increases to 50 USD with 3000 USD/ton MEA solvent price, i.e., 6.4 % increase.
4. Conclusions
An extensive study has been conducted by Technology Centre Mongstad together with AspenTech to develop CO2 capture process cost baseline for 555 MWe NGCC power plant using non-proprietary 30 wt% MEA solution as solvent to guide project developers as well as new technology developers to assess the project feasibility as well as merits of new technologies.
This study explains in detail all the essential steps involved in developing a cost baseline starting from the model development, validation with pilot scale operational data, simulation of process at the desired scale, detailed equipment design, project costs estimation and finally analysis of the costs to estimate cost of CO2 capture. Multiple AspenTech software tools (Aspen Plus, Aspen EDR, ACCE) as well as TCM internal calculation tools together with TCM practical operational knowledge is used to come up with reliable costing.
From the current study, it can be seen that using AspenTech products together with domain expertise, amine based post-combustion carbon capture projects costs can be estimated and these estimates can provide reliable budgetary estimation for CO2 capture projects.
For FEED studies, the accuracy of the total project estimation is likely not sufficient since FEED studies are often relative to specific sites. But the estimation of alternatives (process, utilities) may be useful with AspenTech tools during FEED studies.
For other studies, AspenTech tools are useful for:
- Feasibility studies
- Screening of project alternatives
- Conceptual studies
- Preliminary cost estimations
Acknowledgements
The authors gratefully acknowledge the staff of TCM DA, Gassnova, Equinor, Shell and TotalEnergies for their contribution and work at the TCM DA facility. The authors also gratefully acknowledge Gassnova, Equinor, Shell, and TotalEnergies as the owners of TCM DA for their financial support and contributions. The authors also thank AspenTech for providing working sessions for the present study
References
- D. Thimsen, A. Maxson, V. Smith, T. Cents, O. Falk-Pedersen, O. Gorset, E.S. Hamborg, Results from MEA testing at the CO2 Technology Centre Mongstad. Part I: Post-Combustion CO2 capture testing methodology, Energy Procedia. 63 (2014) 5938–5958. https://doi.org/10.1016/j.egypro.2014.11.630.
- E.S. Hamborg, V. Smith, T. Cents, N. Brigman, O.F.- Pedersen, T. De Cazenove, M. Chhaganlal, J.K. Feste, Ø. Ullestad, H. Ulvatn, O. Gorset, I. Askestad, L.K. Gram, B.F. Fostås, M.I. Shah, A. Maxson, D. Thimsen, Results from MEA testing at the CO2 Technology Centre Mongstad. Part II: Verification of baseline results, Energy Procedia. 63 (2014) 5994–6011. https://doi.org/10.1016/j.egypro.2014.11.634.
- L. Faramarzi, D. Thimsen, S. Hume, A. Maxon, G. Watson, S. Pedersen, E. Gjernes, B.F. Fostås, G. Lombardo, T. Cents, A.K. Morken,M.I. Shah, T. de Cazenove, E.S. Hamborg, Results from MEA Testing at the CO2 Technology Centre Mongstad: Verification of Baseline Results in 2015, Energy Procedia. 114 (2017) 1128–1145. https://doi.org/10.1016/j.egypro.2017.03.1271.
- C. Benquet, A.B.N. Knarvik, E. Gjernes, O.A. Hvidsten, E. Romslo Kleppe, S. Akhter, First Process Results and Operational Experience with CESAR1 Solvent at TCM with High Capture Rates (ALIGN-CCUS Project), SSRN Journal. (2021). https://doi.org/10.2139/ssrn.3814712.
- S.A. Hume, M.I. Shah, G. Lombardo, T. de Cazenove, A. Maxson, C. Benquet, Results from MEA testing at the CO2 Technology Centre Mongstad. Verification of Residual Fluid Catalytic Cracker (RFCC) baseline results, SSRN Electronic Journal. (2021) 12.
- B. Languille, A. Drageset, T. Mikoviny, E. Zardin, C. Benquet, Ø. Ullestad, M. Aronson, E.R. Kleppe, A. Wisthaler, Atmospheric Emissions of Amino-Methyl-Propanol, Piperazine and Their Degradation Products During the 2019-20 ALIGN-CCUS Campaign at the Technology Centre Mongstad, SSRN Journal. (2021). https://doi.org/10.2139/ssrn.3812139.
- S.A. Hume, B. McMaster, A. Drageset, M.I. Shah, E.R. Kleppe, Results from CESAR1 testing at the CO2 Technology Centre Mongstad. Verification of Residual Fluid Catalytic Cracker (RFCC) baseline results, in: Lyon, France, 2022.
- J. Haslback, N. Kuehn, E. Lewis, L.L. Pinkerton, J. Simpson, M.J. Turner, E. Varghese, M. Woods, Cost and Performance Baseline for Fossil Energy Plants, Volume 1: Bituminous Coal and Natural Gas to Electricity, Revision 2a, National Energy Technology Laboratory (NETL), Pittsburgh, PA, Morgantown, WV, and Albany, OR (United States), 2013. https://doi.org/10.2172/1513268.
- K.R. Putta, D.D.D. Pinto, H.F. Svendsen, H.K. Knuutila, CO2 absorption into loaded aqueous MEA solutions: Kinetics assessment using penetration theory, International Journal of Greenhouse Gas Control. 53 (2016) 338–353.
- AspenTech, Rate-Based Model of the CO2 Capture Process by MEA using Aspen Plus, 2018.
- K.R. Putta, H.F. Svendsen, H.K. Knuutila, Kinetics of CO2 Absorption in to Aqueous MEA Solutions Near Equilibrium, Energy Procedia. 114 (2017) 1576–1583.
- S. Roussanaly, N. Berghout, T. Fout, M. Garcia, S. Gardarsdottir, S.M. Nazir, A. Ramirez, E.S. Rubin, Towards improved cost evaluation of Carbon Capture and Storage from industry, International Journal of Greenhouse Gas Control. 106 (2021) 103263. https://doi.org/10.1016/j.ijggc.2021.103263.
Development of process model of CESAR1 solvent system and validation with large pilot data (2022)
Joshua C. Morgana,b,*, Matthew Campbellc, Koteswara Rao Puttac, Muhammad Ismail Shahc, Michael Matuszewskia,b, Benjamin Omella
a National Energy Technology Laboratory (NETL), 626 Cochrans Mill Road, Pittsburgh PA, 15236
b NETL Support Contractor, 626 Cochrans Mill Road, Pittsburgh PA, 15236
c CO2 Technology Centre Mongstad, Mongstad 71, 5954 Mongstad, Norway
*Corresponding author. Tel.: +1-412-386-5357, E-mail address: Joshua.Morgan@netl.doe.gov
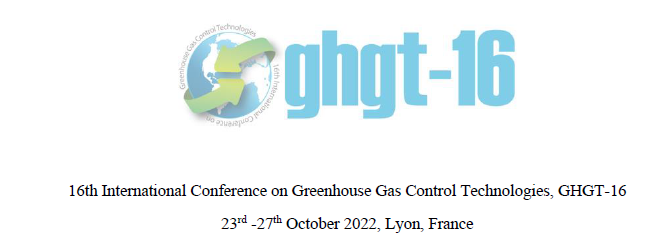
Abstract
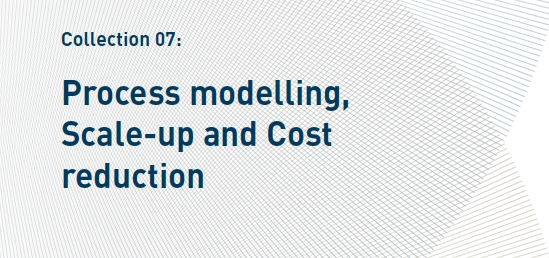
The United States (U.S.) Department of Energy (DOE)-sponsored Carbon Capture Simulation for Industry Impact (CCSI2) is collaborating with Norway’s Technology Centre Mongstad (TCM) on the development and validation of a process model of the CESAR1 solvent system for post-combustion carbon capture applications. The CESAR1 solvent, developed through the CO2 Enhanced Separation and Recovery (CESAR) project, is an aqueous blend of 2-amino-2-methyl-1-propanol (AMP) and piperazine (PZ) with concentrations of approximately 3 M and 1.5 M, respectively. The process model, developed in the Aspen Plus® software platform, uses thermodynamic and kinetic models from the AMP-H2O-CO2 and PZ-H2O-CO2 system models distributed by Aspen Tech. Enhancements in this work include calibrating the interaction parameters for the AMP-PZ pair with thermodynamic data from the open literature for the AMP-PZ-H2O-CO2 system and updating the reaction kinetics parameters to ensure thermodynamic consistency with the chemical equilibria. The process model is validated with a set of seven steady-state test runs, collected over a wide range of operating conditions at the pilot plant at TCM (12 MWe scale) with natural gas-based combined cycle turbine flue gas (~ 3.5 vol% CO2). The integrated process model developed for the TCM pilot plant includes rate-based models for the CO2 absorption and solvent regeneration processes and predicts key output variables (e.g., CO2 capture percentage, specific reboiler duty) within ± 5% for the validation data set. This paper presents model development and validation work for an initial version of the CESAR1 process model along with discussion of future updates to be made to the model prior to its open-source release.
1. Introduction
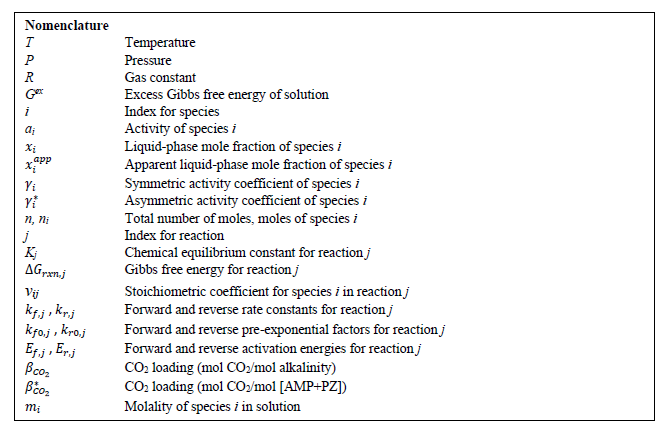
The United States (U.S.) Department of Energy (DOE)-sponsored Carbon Capture Simulation for Industry Impact (CCSI2) is a collaboration among national laboratories, industry organizations, and academic institutions focused on application of the open-source toolset developed through its predecessor program, Carbon Capture Simulation Initiative (CCSI) [1]. The CCSI Toolset (https://github.com/CCSI-Toolset) includes a suite of computational tools and models for accelerating the development, deployment, and scale-up of CO2 capture technologies. This toolset includes a process model, implemented in Aspen Plus®, of a monoethanolamine (MEA)-based carbon capture process, which was validated with steady-state data from the National Carbon Capture Center (NCCC) [2–3] and Technology Centre Mongstad (TCM) [4], at scales of 0.5 and 12 MWe, respectively. TCM, owned jointly by Gassnova, Equinor, Shell, and TotalEnergies, is the world’s largest facility for evaluation of novel process technologies in the carbon capture and storage (CCS) field. The TCM staff also provides expertise in process modeling and analysis in addition to 10 years of experience in CCS process operation. In a recent work by Putta et al. [5], TCM has collaborated with Aspen Tech on a rigorous economic analysis of a natural gas combined cycle (NGCC) power plant integrated with an aqueous MEA CO2 capture system achieving 90% capture. This work uses a model of the MEA system that has been validated with process data from TCM for operation with various flue gas sources: natural gas-based (~ 4 vol% CO2), steam- methane reform-based (~ 8-10 vol% CO2), and coal-based (~ 12-14 vol% CO2). In contrast, the previous MEA model developed by the CCSI/CCSI2 team [2–4] has been validated with NCCC data for 7-12 vol% CO2 flue gas and TCM data for 8-10 vol% CO2 flue gas and has yet to be re-evaluated for operation with natural gas-based flue gas.
This paper is based on a collaboration between CCSI2 and TCM focused on the development and validation of a process model of a second-generation amine solvent (CESAR1) for point source carbon capture applications. The CESAR1 solvent, developed through the CO2 Enhanced Separation and Recovery (CESAR) project, is an aqueous blend of 2-amino-2-methyl-1-propanol (AMP) and piperazine (PZ) with concentrations of approximately 3 M (5 m) and 1.5 M (2.5 m), respectively. This corresponds to an aqueous solution of approximately 27 wt% AMP and 13 wt% PZ. This solvent blend has been identified as an improvement over MEA, long considered the nonproprietary standard for solvent-based carbon capture, due to the combination of high reactivity associated with PZ and the lower heat of absorption of AMP, which leads to a reduction in solvent regeneration energy requirement [6–7] as well as stability of the solvent (lower solvent degradation rates).
In this work, the CESAR1 process model was developed by combining existing thermodynamic models distributed with Aspen Tech software of the CO2-loaded aqueous PZ [8] and AMP [9] systems and regressing the interaction parameters for the PZ–AMP pair to fit CO2 partial pressure [6, 10] data for the temperature range of 40–120℃ and heat of absorption data for 40–80℃ [10–11]. A simulation of the pilot plant at TCM was developed in Aspen Plus, with absorber and stripper columns modeled as rate-based columns. In the absorber model, reactions are specified on activity basis with forward and reverse kinetic parameters fit to ensure consistency with the chemical equilibria calculated from the solution thermodynamic model. The process model is validated with seven steady-state data sets from the TCM pilot plant, and the overall model validation set includes variation in solvent (35000–65000 kg/hr) and flue gas flowrates (60000–70000 kg/hr), solvent CO2 loading (0.049–0.176 mol CO2/mol alkalinity), and absorber packing height (12–24 meter). The process model accurately predicts, generally within ± 5%, the key output variables of interest for the process, including percentage of CO2 capture from absorption and reboiler duty for solvent regeneration. Finally, future work on the CESAR1 model, which will be released to the open-source CCSI Toolset in the near future, is discussed. This includes sub-model level uncertainty quantification (UQ) and the extension of the thermodynamic model to operating conditions for the water wash column to allow for accurate characterization of solvent emissions.
2. Model Development
2.1 Thermodynamic Model
The thermodynamic models for the CO2-loaded aqueous PZ and AMP systems were adapted from the documentation distributed with Aspen Tech software [8–9]. In these models, the liquid and vapor phase fugacity values are calculated from the electrolyte non-random two liquid (e-NRTL) model [12] and the Redlich-Kwong equation of state, respectively. Thermodynamic data of relevance to the combined AMP-PZ-H2O-CO2 system were identified from the literature. Hartono et al. [10] includes CO2 partial pressure data and heat of absorption for solutions at 40–80℃. Brúder et al. [6] includes a CO2 partial pressure data set that is generally consistent with that of Hartono et al. [10], but also includes data for 100–120℃, the temperature range of interest for stripping CO2 from solvent. A data set for heat of absorption for solutions at 40–80℃ from Xie et al. [11] was also identified. The parameters of interest for characterizing the AMP-PZ interaction in the activity coefficient model are embedded in the local interactions term in the e-NRTL model for excess Gibbs energy, which is related to the activity coefficient as follows:
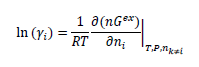
A summary of the e-NRTL activity coefficient model and the details of the parameters used for fitting solvent- based CO2 capture systems is found in our previous work on thermodynamic modeling of the aqueous MEA system [13]. The regressed parameter values for the molecule-molecule interaction for the AMP/PZ pair are given in Table 1. These values were estimated through use of a maximum likelihood objective function implemented in the Aspen Plus data regression system.

When developing this model, it was assumed that the binary pair parameters for the AMP-PZ interaction are symmetric, in that the values for NRTL/1 and NRTL/2 are equal for (AMP/PZ) and (PZ/AMP) pairs. It was found that simultaneous regression of the parameters for (PZ/AMP) in addition to those for (AMP/PZ) did not result in a statistically significant improvement in the model. Furthermore, the model fit did not significantly improve when regressing electrolyte pair parameters for the AMP-PZ interaction. The fit of the model to CO2 partial pressure and heat of absorption data is shown in Figures 1–2, respectively.
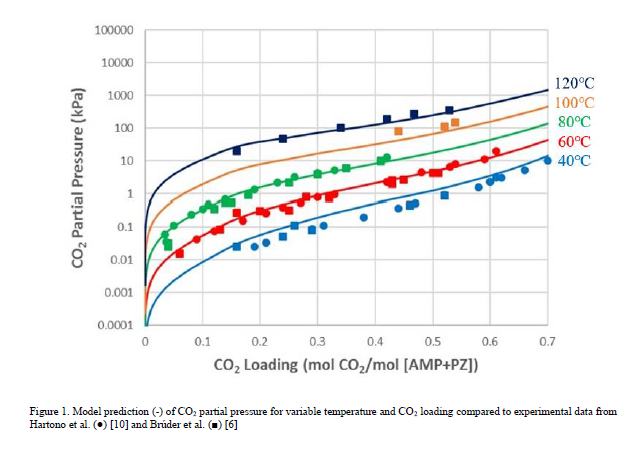
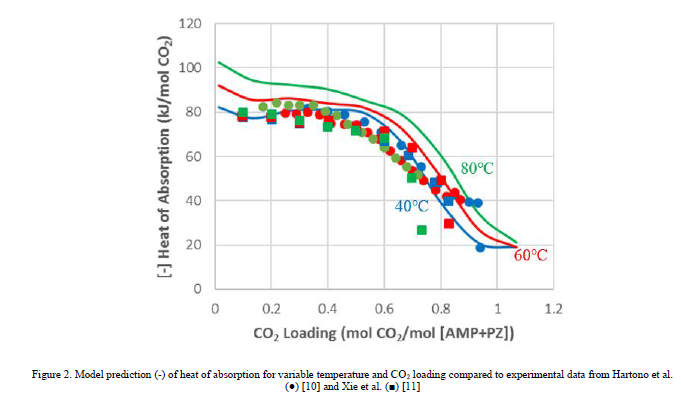
As shown in Figures 1-2, the model generally gives good prediction of the CO2 partial pressure isotherms and captures the trend of heat of absorption with respect to the lean loading. For the CO2 partial pressure, the R2 values for the model fits are 0.922 for data from Brúder et al. [6] and 0.876 for data from Hartono et al. [10]. There is some discrepancy among the two data sources used for heat of absorption [10–11] and the effect of temperature on heat of absorption is unclear. The model predicts that the magnitude of heat of absorption increases with temperature, consistent with the observation for the aqueous MEA system [13]. As a result of this discrepancy, the R2 values for the model fit are lower for the heat of absorption than for the CO2 partial pressure – 0.835 for the data from Hartono et al. [10] and 0.815 for the data from Xie et al. [11]. As the model fit to both heat of absorption and CO2 partial pressure data is generally good at 40°C and 60°C, it is expected that the model will predict with reasonable accuracy absorber operation, in terms of both CO2 capture and temperature bulge due to the exothermic reaction between CO2 and amine. Since no heat of absorption data are available for temperatures above 80°C, the heat of absorption is extrapolated in the modeling of the stripper column, and further analysis would be required to determine the effect this has on model accuracy. Further improvements to the thermodynamic model would likely require uncertainty quantification, for which discussion is forthcoming, or reevaluation of the accuracy of the thermodynamic models of the AMP-H2O-CO2 and PZ-H2O-CO2 subsystems.
2.2 Chemical Equilibrium and Kinetic Models
In this work, the solution chemistry for the AMP-PZ-H2O-CO2 system is represented by the equilibrium reactions shown in Table 2 [8-9].
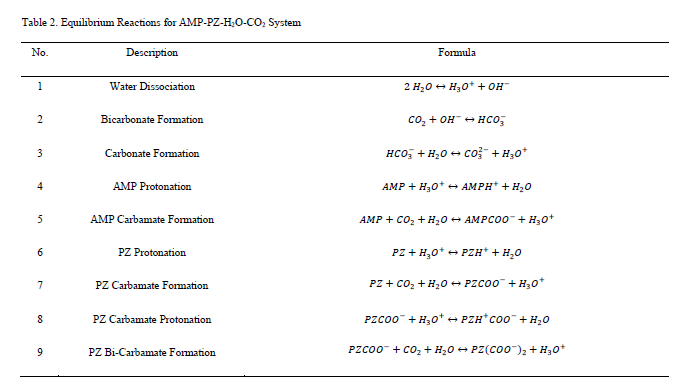
The aggregate reaction set includes amine-independent reactions (No. 1–3) that describe the interactions of CO2 and H2O in addition to reactions specific to each amine sub-system. The amine-specific reactions are represented schematically in Figure 3.
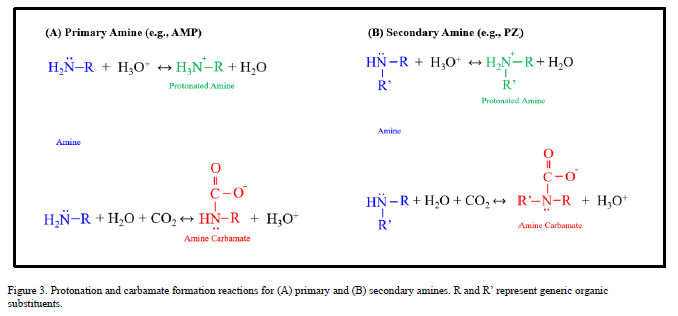
The chemistry of AMP (No. 4–5), a sterically hindered primary amine, is typical for a CO2-loaded aqueous amine system. The amine molecule is in equilibrium with its protonated form through a proton exchange reaction. The amine also forms a carbamate through displacement of a proton by CO2. The chemistry of PZ (No. 6-9), a cyclic compound with two secondary amine groups is more complex in that the additional amine group on the PZ carbamate ion [PZCOO-] can react with hydronium to form a zwitterion [PZH+COO-] or with CO2 to form a bi-carbamate ion [PZ(COO-)2]. For each equilibrium reaction (j), the chemical equilibrium constant is defined as:

Note that CO2 is defined as a Henry component in the model. Thus, the activity is defined in terms of the asymmetric activity coefficient, whereas the symmetric activity coefficient is used for all other species. As in the process models distributed with Aspen Plus [8–9], only the reactions involving CO2 are represented as kinetic reactions in the absorber model. These are summarized in Table 3.
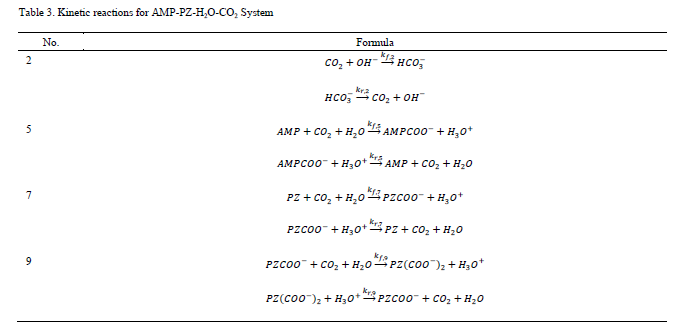
For each reaction (j) for which kinetics are defined, the rate expressions for both forward and reverse reactions are in elementary form and use activity as the concentration basis. The forward reaction rate constants are represented as:

The reverse reaction rate constants are defined analogously. Kinetic parameters for all reactions are given in Table 4.
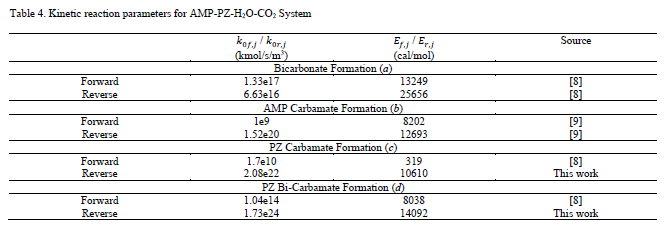
In determining the kinetic parameters for the individual reactions, the rate constants of the forward and reverse reactions should be related to the chemical equilibrium by:

As the kinetic parameter values provided for the bicarbonate formation and AMP carbamate formation reaction in the Aspen Tech models [8–9] are consistent with the chemical equilibria as shown in Eq. 5, these parameter values are also used in this work. This consistency was not observed for the PZ carbamate and bi-carbamate formation reactions, and the reverse rate parameters (𝑘0𝑟,𝑗 and 𝐸𝑟,𝑗) were adjusted to ensure that Eq. 5 is also satisfied for each reaction. This model update was found to be essential not only for ensuring consistency between thermodynamic and kinetic sub-models, but also for obtaining adequate prediction of absorber performance.
2.3 Pilot Plant Model
A schematic of the TCM pilot plant, on which the process model is based, is shown in Figure 4.
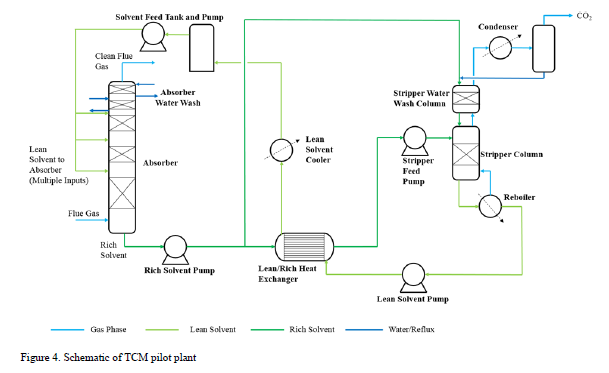
This process represents a typical post-combustion carbon capture system, with two notable process enhancements. The absorber tower consists of two 6-meter packed beds and one 12-meter bed for gas/solvent contacting. There are three inlets to the absorber for the CO2-lean solvent so that testing may be performed with either 12, 18, or 24 meters of packing. Moreover, a bypass system is included so that a fraction (~ 20%) of the CO2-rich solvent exiting the absorber is bypassed from the lean/rich heat exchanger and heated in the stripper water wash column by the vapor exiting the top of the stripper. This process configuration was implemented at TCM to reduce the required energy input to the reboiler. The flowsheet shown in Figure 4 is implemented in Aspen Plus V11, using the thermodynamic and kinetic models described in the previous sections. The absorber and stripper are modeled as rate-based columns with mass transfer and interfacial area models based on the work of Bravo et al. [14]; the liquid hold-up in the absorber column uses the model of Billet and Schultes [15]. The model of the absorber water wash was not included in this work because it was not needed for validation of the absorber/stripper loop but it will be developed in future work focused on prediction of amine emissions, for which discussion is forthcoming.
3. Model Validation
Seven steady-state data sets, collected at TCM during a test campaign in September–October 2019 sponsored by the Accelerating Low Carbon Industrial Growth through Carbon Capture, Utilisation, and Storage (ALIGN-CCUS) program [16], are used to validate the process model. Shown in Table 5, the overall data set includes substantial variation in process operating conditions, including absorber packing height and the flowrates of circulated solvent, flue gas, and reboiler steam. For all cases, natural gas-based combined cycle turbine flue gas, containing 3.5% by volume CO2, was used. It is also notable that the level of CO2 capture varies from 90–98.5%, while the approximate level of CO2 capture required for net-zero emissions for this flue gas source is approximately 99%.
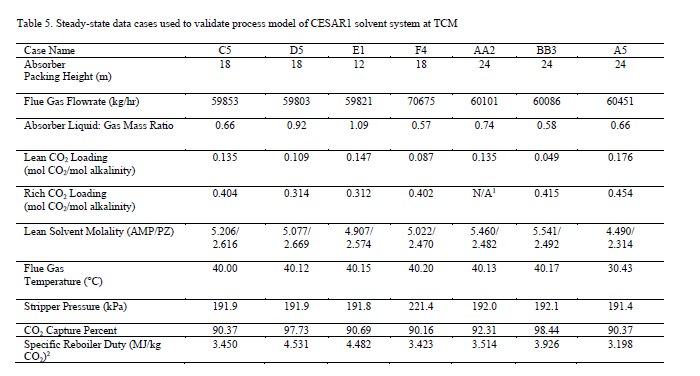
2 Specific reboiler duty (SRD) is the ratio of the reboiler duty to the mass of CO2 captured – which is determined through a mass balance around the absorber
In Table 5, the CO2 loading is defined in terms of total alkalinity:

This is done for consistency with the liquid phase composition measurements at TCM. However, the convention used for CO2 loading in the context of thermodynamic model validation (Figures 1 – 2) is:

This convention follows that used in the sources for which thermodynamic data were obtained [6; 10 –11]. It can be shown that the two definitions of CO2 loading are related as follows:

The process model of the TCM pilot plant with CESAR1 solvent system developed in this work is evaluated for all data cases. The absorber model performance is evaluated by comparing experimental values and model predictions of CO2 capture percentage and CO2 loading in the rich solvent exiting the absorber. A perturbation factor in the liquid holdup sub-model is included to minimize the error in the absorber model, although this assumption will be analyzed further through uncertainty quantification of the mass transfer, kinetic, and holdup sub-models in future work. Parity plots for the model predictions of the absorber output variables are shown in Figure 5.
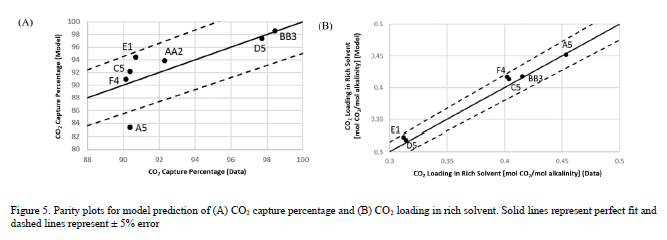
As shown in Figure 5, the model predicts the CO2 capture percentage and CO2 loading in the rich solvent within ±5 for most data cases, although the model seems to underpredict the percentage of CO2 capture for case A5. However, the model accurately predicts the CO2 loading in the rich solvent for this case, which suggests an inaccuracy in the mass balance closure for this case. The stripper model performance is evaluated through the specific reboiler duty, which is the ratio of the reboiler duty and the mass of CO2 captured, and the temperature of the lean solvent exiting the bottom of the column. Parity plots for the model predictions of the stripper output variables are shown in Figure 6. Moreover, Table 6 includes a summary of the percentage error in the model prediction of key process variables for the CESAR1 model.
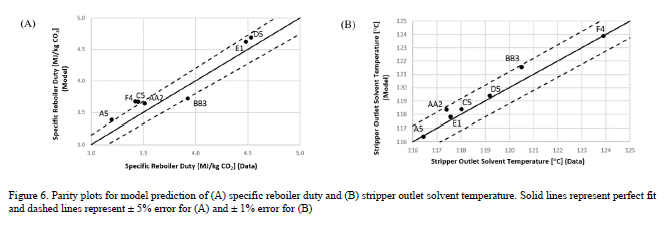
Figure 6. Parity plots for model prediction of (A) specific reboiler duty and (B) stripper outlet solvent temperature. Solid lines represent perfect fit and dashed lines represent ± 5% error for (A) and ± 1% error for (B).

The specific reboiler duty is generally predicted with percentage error of ± 5% for all cases, and the percentage error in the prediction of the stripper outlet temperature is less than 1% for all cases.
4. Conclusions
In summary, a model was developed for the CESAR1 post-combustion carbon capture process at the TCM pilot plant for a flue gas source consistent with natural gas conditions. The model was validated with seven steady-state data sets, representing a variety of operating conditions and CO2 capture levels near the point of net-zero emissions (~ 99% capture for flue gas from natural gas combustion), and found to be predictive of key output variables for both the CO2 absorption and solvent regeneration processes. Ongoing and future work on the CESAR1 model will focus on analysis of the solution thermodynamics and reaction kinetics of the underlying sub-systems of the AMP-PZ-H2O-CO2 system (i.e., CO2-loaded aqueous PZ and AMP systems). While the thermodynamic models were deemed accurate for characterization of the absorber and stripper performance for the concentration of interest for the CESAR1 solvent system (5 m AMP, 2.5 m PZ), further analysis of the sub-systems would be required to ensure accuracy of the model for AMP–PZ blends of varying concentrations. Parametric UQ will be implemented on the key process sub-models, including thermodynamics, reaction kinetics, liquid holdup, and mass transfer/interfacial area to enable stochastic estimation of model performance at pilot scale. This will be essential for quantifying technical process risk associated with scale-up as well as estimating the economic performance prior to the deployment of CESAR1 as a plant-scale CCS system. In a separate effort under the Sustainable OPEration of post-combustion Capture plants (SCOPE) program the CESAR1 modeling framework will be expanded to include the absorber water wash section for quantifying the level of solvent emissions associated with the process. The SCOPE project [17], funded through the Accelerating CCS Technologies (ACT) program, is focused on supporting the development of technology for greenhouse gas emissions control through large scale deployment of effective emissions management tools and demonstration at CO2 capture pilot plants. Accurate prediction of amine emissions requires thermodynamic models that are valid for solutions with much lower amine concentrations than those encountered in the absorber/stripper loop. One of the goals of SCOPE is to fill the current gap in the literature for vapor-liquid equilibria data for solutions of low amine concentration, thus enabling the development of more accurate amine emissions models. Finally, the CESAR1 process model will be released as part of the open-source CCSI Toolset in the near future to accompany the existing baseline aqueous MEA model for use by researchers for such analyses including, but not limited to, multi- scale modeling and validation, techno-economic analysis, and rigorous comparison of solvent-based CO2 capture systems.
References
[1] Miller DC, Syamlal M, Mebane DS, Storlie C, Bhattacharyya D, Sahinidis NV, Agarwal D, Tong C, Zitney SE, Sarkar A, Sun X, Sundaresan S, Ryan E, Engel D, Dale C, 2014. Carbon capture simulation initiative: a case study in multiscale modeling and new challenges. Annu. Rev. Chem. Biomol. Eng. 5: 301-323.
[2] Morgan JC, Chinen AS, Omell B, Bhattacharyya D, Tong C, Miller DC, Buschle B, Lucquiaud M, 2018. Development of a rigorous modeling framework for solvent-based CO2 capture: Part 2: steady-state validation and uncertainty quantification with pilot plant data. Ind. Eng. Chem. Res. 57: 10464-10481.
[3] Morgan JC, Chinen AS, Anderson-Cook C, Tong C, Carroll J, Saha C, Omell B, Bhattacharyya D, Matuszewski M, Bhat KS, Miller DC, 2020. Development of a framework for sequential Bayesian design of experiments: application to a pilot-scale solvent-based CO2 capture process. Appl. Energy. 262: 114533.
[4] Morgan JC, Omell B, Matuszewski M, Miller DC, Shah MI, Benquet C, Knarvik ABN, de Cazenove T, Anderson-Cook CM, Ahmed T, Tong C, Ng B, Bhattacharyya D, 2021. Application of sequential design of experiments (SDoE) to large pilot-scale solvent-based CO2 capture process at Technology Centre Mongstad (TCM). Proceedings of the 15th International Conference on Greenhouse Gas Control Technologies. 15-18 March 2021, Available at SSRN: https://ssrn.com/abstract=3811695
[5] Putta KR, Saldana D, Campbell M, Shah MI, 2022. Development of CO2 capture process cost baseline for 555 MWe NGCC power plant using standard EA solution. Proceedings of the 16th International Conference on Greenhouse Gas Control Technologies. 23 – 27 October 2022, Lyon, France.
[6] Brúder P, Grimsvedt A, Mejdell T, Svendsen HF, 2011. CO2 capture into aqueous solutions of piperazine activated 2-amino-2-methyl-1- propanol. Chem. Eng. Sci. 66: 6193-6198.
[7] Cousins A, Feron P, Hayward J, Jiang K, Zhai R, 2019. Further Assessment of Emerging CO2 Capture Technologies for the Power Sector and their Potential to Reduce Costs: IEAGHG Technical Report. Available at http://documents.ieaghg.org/index.php/s/YKm6B7zikUpPgGA?path=%2F2019%2FTechnical%20Reports
[8] Aspen Plus documentation, 2014. Rate-based model of the CO2 capture process by PZ using Aspen Plus. Aspen Technology Inc, Cambridge MA.
[9] Aspen Plus documentation, 2014. Rate-based model of the CO2 capture process by AMP using Aspen Plus. Aspen Technology Inc, Cambridge MA.
[10] Hartono A, Ahmad R, Svendsen HF, Knuutila HK, 2021. New solubility and heat of absorption data for CO2 in blends of 2-amino-2-methyl- 1-propanol (AMP) and piperazine (PZ) and a new eNRTL model representation. Fluid Phase Equilib. 550: 113235.
[11] Xie Q, Aroonwilas A, Veawab A, 2013. Measurement of heat of CO2 absorption into 2-amino-2-methyl-1-propanol (AMP)/piperazine (PZ) blends using differential reaction calorimeter. Energy Procedia. 37: 826-833.
[12] Chen CC, Evans LB, 1986. A local composition model for the excess Gibbs energy of aqueous electrolyte systems. AIChe J. 32(3): 444-454.
[13] Morgan JC, Chinen AS, Omell B, Bhattacharyya D, Tong C, Miller DC, 2017. Thermodynamic modeling and uncertainty quantification of CO2-loaded aqueous MEA solutions. Chem Eng Sci. 168: 309-324.
[14] Bravo JL, Rocha JA, Fair JR, 1985. Mass transfer in gauze packings. Hydrocarbon Proc. 64(1):91.
[15] Billet R, Schultes M, 1993. Predicting mass transfer in packed columns. Chem Eng Technol. 16, 1-9.
[16] Benquet C, Knarvik A, Gjernes E, Hvidsten OA, Kleppe ER, Akhter S, 2021. First process results and operational experience with CESAR1 solvent at TCM with high capture rates (ALIGN-CCUS project). Proceedings of the 15th International Conference on Greenhouse Gas Control Technologies. 15-18 March 2021, Abu Dhabi, UAE.
[17] Kvamsdal HM, van Os P, Moser P, Korre A, Martin A, Matuszewski MS, Gupta NC, Khakharia P, McNally C, 2022. SCOPE: an ERA-NET ACT project on sustainable operation of post-combustion capture plant. Proceedings of the 16th International Conference on Greenhouse Gas Control Technologies. 23 – 27 October 2022, Lyon, France.
Disclaimer
This project was funded by the Department of Energy, National Energy Technology Laboratory an agency of the United States Government, through a support contract. Neither the United States Government nor any agency thereof, nor any of its employees, makes any warranty, express or implied, or assumes any legal liability or responsibility for the accuracy, completeness, or usefulness of any information, apparatus, product, or process disclosed, or represents that its use would not infringe privately owned rights. Reference herein to any specific commercial product, process, or service by trade name, trademark, manufacturer, or otherwise does not necessarily constitute or imply its endorsement recommendation, or favoring by the United States Government or any agency thereof. The views and opinions of authors expressed herein do not necessarily state or reflect those of the United States Government or any agency thereof.
Acknowledgements
The authors graciously acknowledge funding from the U.S. Department of Energy, Office of Fossil Energy and Carbon Management, through the Carbon Capture Program.
The authors gratefully acknowledge the staff of TCM DA, Gassnova, Equinor, Shell, and TotalEnergies for their contribution and work at the TCM DA facility. The authors also gratefully acknowledge Gassnova, Equinor, Shell, and TotalEnergies as the owners of TCM DA for their financial support and contributions.
Application of Sequential Design of Experiments (SDoE) to Large Pilot-Scale Solvent-Based CO2 Capture Process at Technology Centre Mongstad (TCM) (2021)
Joshua C. Morgana,b,*, Benjamin Omella, Michael Matuszewskia, David C. Millera, Muhammad Ismail Shahc, Christophe Benquetc, Anette Beate Nesse Knarvikc, Thomas de Cazenovec, Christine M. Anderson-Cookd, Towfiq Ahmedd, Charles Tonge, Brenda Nge, Debangsu Bhattacharyyaf
aNational Energy Technology Laboratory, 626 Cochrans Mill Road, P.O. Box 10940, Pittsburgh PA 15236-0940, USA b NETL Support Contractor, 626 Cochrans Mill Road, P.O. Box 10940, Pittsburgh PA 15236-0940, USA cCO2 Technology Centre Mongstad, Mongstad 71, 5954 Mongstad, Norway dLos Alamos National Laboratory, P.O. Box 1663, Los Alamos NM 87545, USA eLawrence Livermore National Laboratory, 7000 East Ave, Livermore CA 94550, USA fWest Virginia University, Department of Chemical and Biomedical Engineering, 1306 Evansdale Drive, P.O. Box 6102, Morgantown WV 26506-6102, USA *Corresponding author
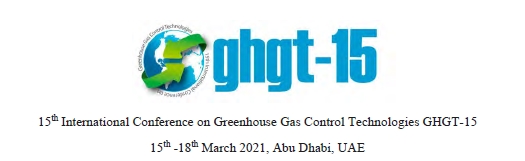
Abstract
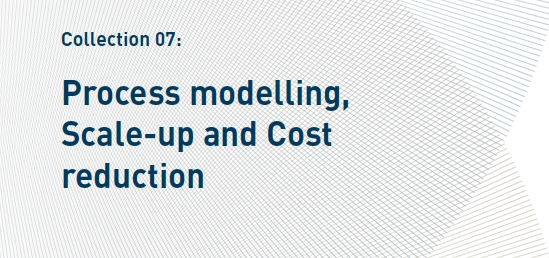
The United States Department of Energy’s Carbon Capture Simulation for Industry Impact (CCSI2) program has developed a framework for sequential design of experiments (SDoE) that aims to maximize knowledge gained from budget- and schedule- limited pilot scale testing. SDoE was applied to the planning and execution of campaigns for testing CO2 capture systems at pilot- scale in order to optimally allocate resources available for the testing. In this methodology, a stochastic process model is developed by quantifying the parametric uncertainty in submodels of interest; for a solvent-based CO2 capture system, these may include physical properties and equipment performance submodels (e.g., mass transfer, interfacial area). This uncertainty is propagated through the full process model, over variable operating conditions, for estimating the resulting uncertainty in key model outputs (e.g., percentage of CO2 capture, solvent regeneration energy requirement). In developing a data collection plan, the predicted output uncertainty is incorporated into an algorithm that seeks simultaneously to select process operating conditions for which the predicted uncertainty is relatively high and to ensure that the entire space of operation is well represented. This test plan is then used to guide operation of the pilot plant at varying steady-state conditions, with resulting process data incorporated into the existing model using Bayesian inference to refine parameter distributions. The updated stochastic model, with reduced parametric uncertainty from data collected, is then used to guide additional data collection, thus the sequential nature of the experimental design.
The SDoE process was implemented at the pilot test unit (12 MWe in scale) at Norway’s Technology Centre Mongstad (TCM) in a summer 2018 test campaign with aqueous monoethanolamine (MEA). During the test campaign, the varied operating conditions included the flowrates of circulated solvent, flue gas, and reboiler steam and the CO2 concentration in the flue gas. The process data were used to update probability distributions of mass transfer and interfacial area parameters of a stochastic process model developed by the CCSI2 team. Two iterations of the SDoE process were executed, resulting in the uncertainty in model predicted CO2 capture percentage decreasing by an average of 58.0 ± 4.7% over the full input space of interest. This work demonstrates the potential of the SDoE process for model refinement through reduction in process model parametric uncertainty, and ultimately risk in scale-up, in CO2 capture technology performance.
1. Introduction
The United States Department of Energy’s Carbon Capture Simulation for Industry Impact (CCSI2) program is a collaboration of national laboratories, universities, and industrial organizations that provides research and development support for novel CO2 capture technologies with the objective of reducing risk and accelerating their commercialization. These efforts involve continuing advancements in and applications of the open-source toolset1 developed as part of its predecessor project, the Carbon Capture Simulation Initiative (CCSI). The CCSI Toolset includes a suite of computational tools and models with the overarching goal of accelerating the development, deployment, and scale-up of CO2 capture technologies. The toolset includes a rigorous process model, implemented in Aspen Plus®, of the aqueous monoethanolamine (MEA) solvent system, which is the industrial standard for solvent- based CO2 capture. This model includes quantification of parametric uncertainty for solvent physical property models such as viscosity, density, and surface tension [1], the thermodynamic framework [2], and packing-specific models such as mass transfer, interfacial area, and hydraulics [3]. These submodels combine with a full process model that was validated with process data from the 0.5 MWe pilot test unit at the National Carbon Capture Center (NCCC) in 2014 [4]. In 2017, an additional test campaign for the aqueous MEA system was held at NCCC, incorporating the CCSI2 framework for SDoE. In this methodology, the existing process model is leveraged to inform collection of data that are subsequently used to refine the model and modify the test plan accordingly [5,6]. Over two iterations of the SDoE process, parametric distributions for process submodels were refined through experimental observations of absorber CO2 capture percentage, resulting in an average uncertainty reduction of approximately 50% for the model prediction of CO2 capture percentage throughout the input space of interest.
The CCSI aqueous MEA process model was scaled up to 12 MWe for consistency with the pilot test unit at Norway’s Technology Centre Mongstad (TCM) and was used in the planning and execution of a test campaign at TCM in summer 2018. TCM is one of the world’s largest facilities for testing carbon capture technologies, and previous test results with the MEA solvent system have been reported in the open literature [7-12], including variation in many process variables and both steady-state and dynamic operation. The pilot plant at TCM notably has two sources of flue gas: combined cycle gas turbine (CCGT) based heat and power plant (CHP), with ~3.5 vol% CO2, and residual fluidized catalytic cracker (RFCC) unit, with ~13-14 vol% CO2. The TCM plant also contains two stripper columns, each designed for process operation with one of the flue gas sources. This work focused on collecting additional data for the MEA process at TCM with variation in the flowrates of solvent, flue gas, and reboiler steam, the concentration of CO2 in the flue gas, the packing height of the absorber, and the stripper configuration. During the first three weeks of the test campaign, which are the primary focus of this paper, the SDoE framework was used to guide the collection of process data using the existing MEA process model and multiple test objectives. The data were used to update the model by refining the distributions of parameters in the mass transfer and interfacial area submodels, ultimately resulting in a reduction of predicted uncertainty in the CO2 capture percentage from 10.5 ± 1.5% to 4.4 ± 0.4%, or an average reduction of 58.0 ± 4.7%, over the full input space of interest. In the final two weeks of the campaign, data were collected for a modified process configuration in which the packing height of the absorber was reduced to 18 meters, and eventually 12 meters, and the stripper configuration was modified so that a fraction (~20%) of the rich solvent exiting the absorber bypasses the lean-rich heat exchanger and is heated in the water wash of the stripper. This work, along with the previous test campaign at NCCC, demonstrates the potential of the SDoE methodology for refining predictions of stochastic process models through strategic data collection. The reduction of model uncertainty effectively reduces expected risk in process design and operation, thus improving confidence when predicting process performance and conducting economic analyses.
2. Methodology
2.1 SDoE Methodology
The SDoE process developed by CCSI2 uses a stochastic model, with parametric uncertainty quantified in the submodels, to inform collection of process data in order to maximize the value of data obtained during a test campaign. Moreover, it provides a framework for directly reducing uncertainty in model prediction of capture rates based on new process knowledge gained from data collection. The SDoE process is represented schematically in Fig. 1.
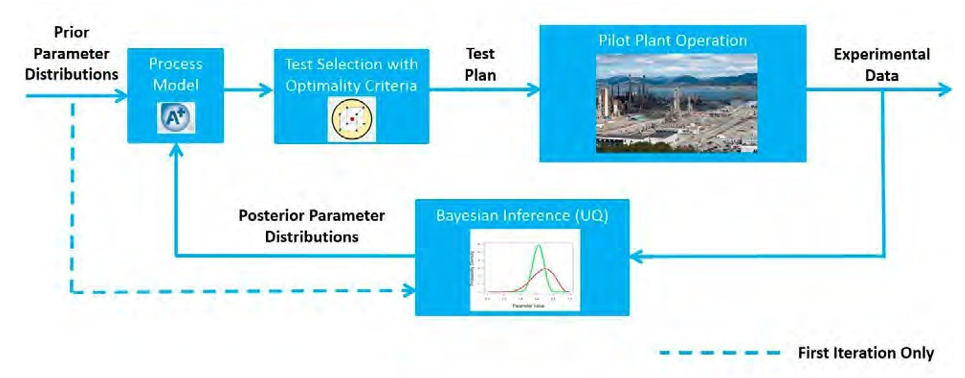
Fig. 1. Schematic representation of SDoE methodology.
As shown in Figure 1, a priori probability distributions of submodel parameters are propagated through a process model, denoted as 𝑦(𝒙, 𝜽, 𝜽∗), where 𝒙 is a set of input variables that can be manipulated in plant operation and 𝜽 and 𝜽∗ are sets of model parameters. These sets differ in that 𝜽 contains parameters for which uncertainty is reduced over the course of executing the SDoE methodology whereas 𝜽∗ has parameters with irreducible uncertainty based on the type of data collected. For the example of a solvent-based CO2 capture system, 𝜽 includes process specific parameters from submodels such as mass transfer or interfacial area that may be informed through collection of plant data (e.g. CO2 capture percentage in the absorber column). Conversely, 𝜽∗ includes parameters from physical property submodels, for which uncertainty is readily estimated through direct measurements of the corresponding properties and cannot reasonably be informed from plant level data. If the process model y is sufficiently complex, it may be necessary to replace it with a surrogate model, denoted as 𝑦̂(𝒙, 𝜽, 𝜽∗), developed and validated over the full input space. For a given point in the input space, a confidence interval for the model prediction are computed by propagating the uncertainty in the full set of parameters (𝜽𝑻 = [𝜽 𝜽∗]) through the surrogate model. The 95% confidence interval, estimated by taking a sample of size M over the full parameter space (𝜽𝑻,(𝒋), ∀ 𝑗 = 1, … 𝑀), is given as:

where {𝑦̂(𝒙(𝒊), 𝜽𝑻,(𝟏)), . . . , 𝑦̂(𝒙(𝒊), 𝜽𝑻,(𝑴))} is the set of values of an output variable calculated from propagating all of the individual 𝜽𝑻,𝒋) through the surrogate model and 𝐹𝑘 represents the kth percentile of this set.
The values of 𝐶I|𝒙(𝒊) for individual 𝒙(𝒊) are considered in the test selection method; the specific optimality criterion used in this work is G-optimality [13], which minimizes the maximum prediction variance. This aim targets experimental settings 𝒙(𝒊) for which the predicted uncertainty (i.e.,𝐶|𝒙(𝒊)) is relatively large, so that the collection of data at these settings represents high potential for uncertainty reduction. Moreover, the algorithm used in this work for test selection simultaneously seeks to ensure that the full input space is well-represented in the test plan, balancing good representation of design points throughout the region while making locations with large confidence interval widths more likely to be selected.
The test plan is then implemented by running the plant accordingly, resulting in collection of experimental data (denoted Z). The data are incorporated into a Bayesian inference framework, using the PSUADE2 software package. For model parameters of fixed uncertainty, a sample (𝜽∗,(𝒋); ∀ 𝑗 = 1, … , 𝑁) is drawn from their probability distribution 𝑃(𝜽∗). For each sample point 𝜽∗,(𝒋), a posterior distribution for the remaining parameters (𝜽) is calculated:

(2) and given in the form of a set of sample points. Here, 𝐿(𝑍|𝜽, 𝜽∗,(𝒋)) represents the likelihood (some metric used to express the distance between simulation predictions and experimental data) of observing a set of experimental data
(Z) conditioned on the values of the parameters, 𝑃(𝜽) the prior distribution of the parameters for which uncertainty is updated, and 𝜋𝑗(𝜽|𝑍,𝜽∗,(𝒋)) the posterior distribution of 𝜽 conditioned on the observed experimental data and the value of 𝜽∗ for sample j. The overall posterior distribution 𝜋(𝜽|𝑍, 𝜽∗,(𝒋)) is obtained through the process of marginalization, combining all individual 𝜋𝑗(𝜽|𝑍, 𝜽∗,(𝒋)). The updated stochastic model, with refined estimates of parameter uncertainties, is then used to re-calculate 𝐶𝐶𝐶𝐶|𝒙𝒙(𝒊𝒊) throughout the input space. For all subsequent iterations of SDoE, the prior distribution 𝑃𝑃(𝜽𝜽) is replaced by the posterior distribution 𝜋𝑗(𝜽|𝑍, 𝜽∗,(𝒋)) from the previous iteration.
2.2 Overview of TCM Test Campaign
The TCM test campaign ran for five weeks in summer 2018, in five distinct test phases as outlined in Table 1.
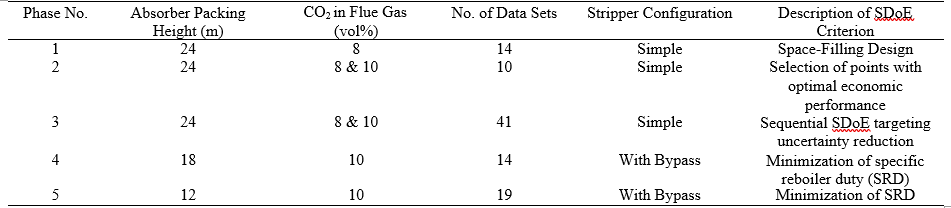
In the first three phases of the campaign, the absorber column was operated with all three packing beds (total height of 24 meters). A conventional stripper configuration was used in which the full amount of rich solvent exiting the absorber is heated in the lean-rich heat exchanger and sent to the top of the stripper column. Throughout the test campaign, flue gas from the CCGT plant (3.5 vol% CO2) was combined with recycle of the captured CO2, increasing the flue gas concentration to 8 or 10 vol% CO2 as required by the test plan. Due to the increased CO2 concentration in the flue gas, and the corresponding increase in the required solvent circulation rate for capturing CO2, the larger stripper intended for use with RFCC flue gas was used during this campaign in lieu of the smaller stripper intended for CCGT flue gas. In Phases 4-5, the packing height of the absorber was reduced by changing the number of beds and the stripper configuration was modified so that approximately 20% of the rich solvent exiting the absorber column bypassed the lean-rich heat exchanger and was instead heated with hot vapor leaving the top of the stripper. This portion of the test campaign, also guided with use of the process model, was focused on identifying the optimal solvent circulation for minimizing the specific reboiler duty for the process. Other process variables were fixed for this portion of the test campaign, including a flue gas flowrate of 50,000 sm3/hr with 10 vol% CO2 and 85% CO2 capture. For the purpose of brevity, the details of Phases 4-5 are not included in this paper.
The first three phases differed in the choice of criteria used for developing the test plan. Phase 1 used a space-filling design to ensure that the process model predicts key outputs such as CO2 capture and specific reboiler duty with reasonable accuracy. Phase 2 focused on collecting data in regions where the model predicts optimal economic performance. Phase 3 focused on collecting data to target regions where the model predicts high uncertainty based on the SDoE procedure in Section 2.1. For all three phases, a desired region of process operation was established based on ranges of operation for flue gas flowrate (𝐺𝐺), CO2 capture percentage (𝐶𝐶𝐶𝐶𝐶𝐶), CO2 loading in the lean solvent entering the absorber column (𝛼𝛼𝑙𝑙𝑙𝑙𝑙𝑙𝑙𝑙), and the volume fraction of CO2 in the flue gas (𝑦𝑦𝐶𝐶𝐶𝐶2 ), with ranges:
𝐺𝐺 ∈ [36,000 − 75,000] 𝑘𝑘𝑘𝑘/ℎ𝑟𝑟 (3a)
𝐶𝐶𝐶𝐶𝐶𝐶 ∈ [80 − 95] % (3b)
𝛼𝛼𝑙𝑙𝑙𝑙𝑙𝑙𝑙𝑙 ∈ [0.10 − 0.25] 𝑚𝑚𝑚𝑚𝑚𝑚 𝐶𝐶𝐶𝐶2/𝑚𝑚𝑚𝑚𝑚𝑚 𝑀𝑀𝑀𝑀𝐶𝐶 (3c)
𝑦𝑦𝐶𝐶𝐶𝐶2 ∈ {0.08,0.10} (3d)
The first three variables are treated as continuous whereas the CO2 fraction in the flue gas is treated as a categorical variable with two process operation levels. For each value of CO2 fraction, a test set consisting of candidate experiments with a unique combination of variables {𝐺𝐺, 𝐶𝐶𝐶𝐶𝐶𝐶, 𝛼𝛼𝑙𝑙𝑙𝑙𝑙𝑙𝑙𝑙} is generated by sampling independently from uniform distributions for each variable with upper and lower limits based on the ranges given in Eq. 3. An Aspen Plus simulation is run for each point in the candidate set to estimate the corresponding values of lean (𝐿𝐿𝑙𝑙𝑙𝑙𝑙𝑙𝑙𝑙) and rich (𝐿𝐿𝑟𝑟𝑟𝑟𝑟𝑟ℎ) solvent flowrate, steam flowrate (𝑆𝑆) and mass of CO2 captured (𝑚𝑚̇ 𝐶𝐶𝐶𝐶𝐶𝐶). To be included in the final candidate set, a point must satisfy the following conditions based on operational limits for the TCM plant:
𝑚𝑚̇ 𝐶𝐶𝐶𝐶𝐶𝐶 < 8,000 𝑘𝑘𝑘𝑘/ℎ𝑟𝑟 (4a)
𝑆𝑆 < 14,000 𝑘𝑘𝑘𝑘/ℎ𝑟𝑟 (4b)
Separate candidate sets (for 𝑦𝑦𝐶𝐶𝐶𝐶2 = 0.08 and 𝑦𝑦𝐶𝐶𝐶𝐶2 = 0.10) were developed using a space-filling approach based on the input vector 𝒙𝒙 = [𝐺𝐺 𝑆𝑆 𝛼𝛼𝑙𝑙𝑙𝑙𝑙𝑙𝑙𝑙]. These candidate sets were used in Phases 1 and 3, although Phase 1 used a space- filling design on the model input space while Phase 3 incorporated the predicted uncertainty in the model output, using the methodology described in Section 2.1. Moreover, only the candidate set for 8 vol% CO2 in flue gas was implemented during Phase 1 of the test campaign due to time considerations.
Phase 2, however, was designed based on an optimization problem of the form:
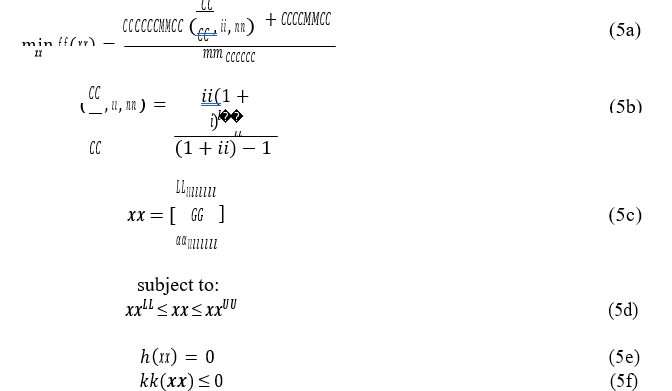
The objective function is the ratio of the equivalent annual operating cost (EAOC) associated with the CO2 capture to the mass of CO2 captured. The EAOC is the sum of the capital cost (CAPEX) multiplied by an annuity factor (𝐶𝐶 , 𝑖𝑖, 𝑛𝑛) and the operating cost (OPEX). Within the annuity factor, 𝐶𝐶 is the ratio of annuity to present value, i is the interest rate, and n is the number of years. The vector of decision variables is denoted as 𝒙𝒙 with lower and upper bounds 𝒙𝒙𝑳𝑳 and 𝒙𝒙𝑼𝑼. The equality constraints denoted by ℎ(𝒙𝒙) includes heat and material balances, and the inequality constraints denoted by 𝑔𝑔(𝒙𝒙) includes the constraints for process operation listed in Eq. 4. The optimization was performed separately for the cases with 8 and 10 vol% CO2 in flue gas. In addition the optimal points in the test plan, additional test points near the optimal points were included. The space surrounding the optimal point can be represented by a cube created by perturbing the input variable values by a chosen amount (10% for this study) from their estimated optimal values. A design that permutes each factor away from this estimated optimum one at a time would require seven test points, or six for the center of each face of the cube (if each factor is manipulated one at a time) and one for the center (optimal) point. As shown in Fig. 2., the design size was reduced to five by considering a fractional factorial structure, which also allows exploration of potential interactions between input factors around the optimum [14].
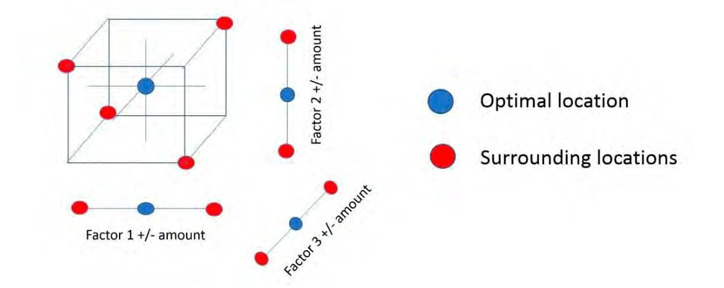
Since two levels of 𝑦𝑦𝐶𝐶𝐶𝐶2 were included in the analysis, the reduction of the overall number of points required for the Phase 2 test plan from 14 to 10 was highly beneficial due to the limited amount of time available for the test campaign.
3. Results
3.1 Phase 1
In Phase 1, the test plan was developed using a minimax space-filling methodology [15] to provide an initial data set that was well-representative of the process. For all testing in this phase, the CO2 concentration in the flue gas was fixed at 8 vol%. The set of input variables included in the test matrix differs from that used for space-filling design in that the input variables for the space-filling design were chosen for modeling convenience whereas the input variables in the test matrix were those directly manipulated in the plant operation. In developing the test matrix, the Aspen simulation was used to estimate the rich solvent flowrate and the flue gas flowrate was converted from mass to volumetric units. The test matrix, which was organized in terms of increasing flue gas flowrate for ease of process operation, for Phase 1 is given in Table 2.
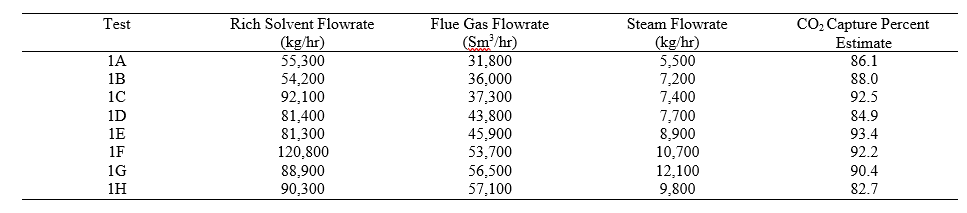
Table 2. Test matrix for Phase 1 design of MEA test campaign at TCM.
When obtaining data for test cases 1A-1B, it was noted that the CO2 capture percentage was substantially lower than the model predictions. This discrepancy was attributed to solvent maldistribution, or uneven flow through the packing, in the RFCC stripper column, resulting in inefficient performance of the column. This stripper was designed to operate at a solvent flowrate of approximately 200,000 kg/hr, or almost four times higher than the solvent flowrate in cases 1A-1B. Therefore, the lean solvent loading for these test runs was substantially higher than that predicted by the model, and the CO2 capture percentage lower. This issue was addressed by dividing each subsequent test run into two intervals with distinct operating goals, so that two data sets were collected for test runs 1C-1H. First, the test was executed with the value of steam flowrate specified in the original test matrix. Upon achieving the steady-state, the steam flowrate was manipulated to match the estimated value of CO2 capture. Parity plots for the model prediction of CO2 capture percentage in the absorber and steam requirement in the stripper are given in Figure 3.
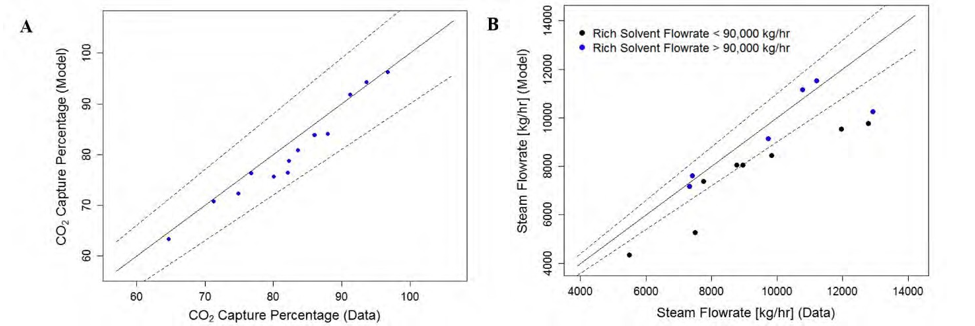
Figure 3. Parity plots for (A) CO2 capture percentage and (B) steam flowrate required for test runs performed in Phase 1. Dashed lines represent
±10% error.
The original deterministic model, or the model in which all parameters are fixed as point values, predicts the CO2 capture with a range of ±10% error for all test runs. The average error for CO2 capture percentage is -2.51 ± 2.29%, with the negative error indicating that the model generally underpredicts the data. The model predicts stripper steam requirement with an average error of -10.83 ± 10.82%, although the error is notably higher for cases in which the solvent flowrate is below 90,000 kg/hr (average error of -16.43 ± 8.49%) than when it is higher than 90,000 kg/hr (average error of -3.67 ± 9.29%). This discrepancy is likely due to liquid maldistribution in the stripper column, as discussed previously. The results obtained in the first phase of the test campaign demonstrated that the initial process model was sufficiently accurate to proceed with the sequential experimental design in subsequent stages.
3.3 Phases 2-3
During the test campaign, data for Phases 2-3 were collected simultaneously and used to update the model parameter distributions through Bayesian inference. The majority of the data for Phase 2 were actually collected after those for Phase 3 due to scheduling convenience. The optimization problem described in Eq. 5 was implemented separately for the 8 and 10 vol% CO2 cases, and used to develop the test matrix given in Table 3.
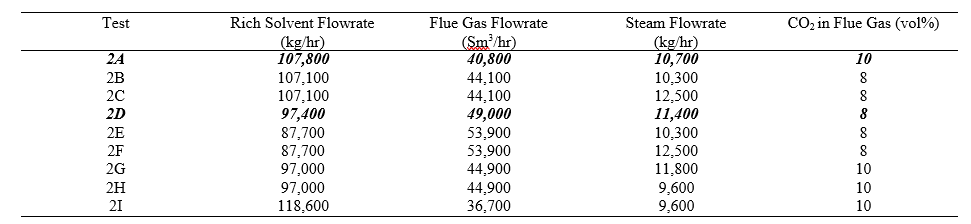
Table 3. Test matrix for Phase 2 design of MEA test campaign at TCM.
In Table 3, the optimal points determined from solving separate optimization problems (Eq. 5) for the 𝑦𝑦𝐶𝐶𝐶𝐶2 = 0.08
(2A) and 𝑦𝑦𝐶𝐶𝐶𝐶2 = 0.10 (2D) cases are highlighted, and additional test points were selected by perturbing the variables by ± 10% from the optimal values. Parity plots for the model prediction of CO2 capture percentage in the absorber and steam requirement in the stripper are given in Fig. 4.
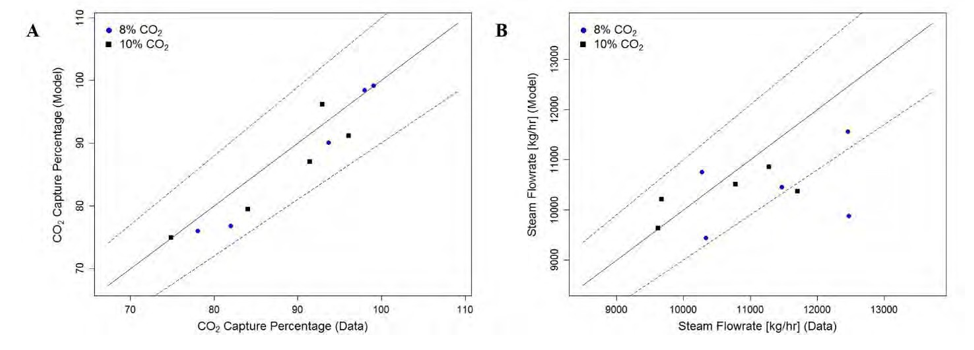
Fig. 4. Parity plots for (A) CO2 capture percentage and (B) steam flowrate required for test runs performed in Phase 2. Dashed lines represent
±10% error.
As shown in Figure 4, the accuracy of the model for the data collected in Phase 2 is comparable to that in Phase 1. The average percent error for the CO2 capture prediction and steam requirement prediction is -2.40 ± 3.27% and -5.28
± 8.00%, respectively. The percentage error for the steam requirement prediction is substantially lower for the data collected in Phase 2 than in Phase 1 due to the absence of test runs with very low (< 85,000 kg/hr) solvent flowrate. Therefore, the model was shown to be sufficiently accurate for the region of the input space likely to be economically optimal.
Phase 3 of the test campaign was focused on data collection in regions where the stochastic model predicts relatively high uncertainty for the absorber CO2 capture percentage. These data, along with those collected in Phase 2, were used to update the mass transfer and interfacial area model parameter distributions. The test matrix for Phase 3 is shown in Table 4.
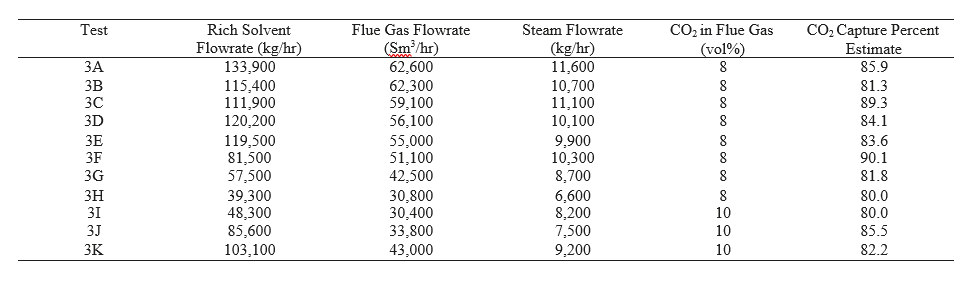
Table 4. Test matrix for Phase 3 design of MEA test campaign at TCM (First Iteration).
The data collected in Table 4, along with case 2A from Table 3 were used in the Bayesian inference procedure based on Eq. 2. In this work, the parameters contained in 𝜽𝜽 included the leading coefficients for the interfacial area and mass transfer submodels developed in previous work [3], and the parameters contained in 𝜽𝜽∗ included the thermodynamic model parameters for which distributions were estimated in previous work [2]. Upon obtaining the updated parameter distributions, the refined stochastic model was used to develop a new test matrix, shown in Table 5.
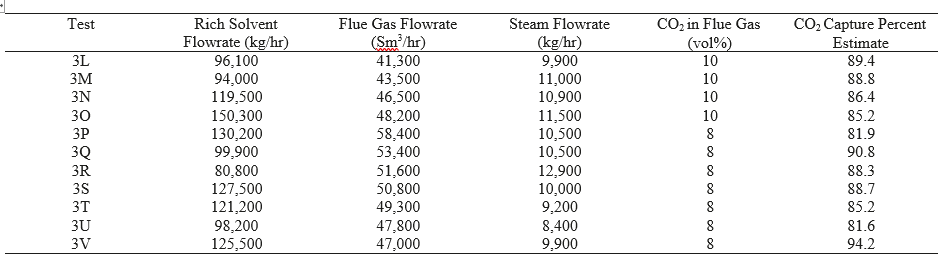
Table 5. Test matrix for Phase 3 design of MEA test campaign at TCM (Second Iteration)
The data collected from the test plan given in Table 5, along with cases 2B-2I in Table 3, were used in a second iteration of the SDoE procedure to update the parameter distributions again. Parity plots for the model prediction of CO2 capture percentage in the absorber and steam requirement in the stripper for all data collected in both iterations of Phase 3 are given in Figure 5.
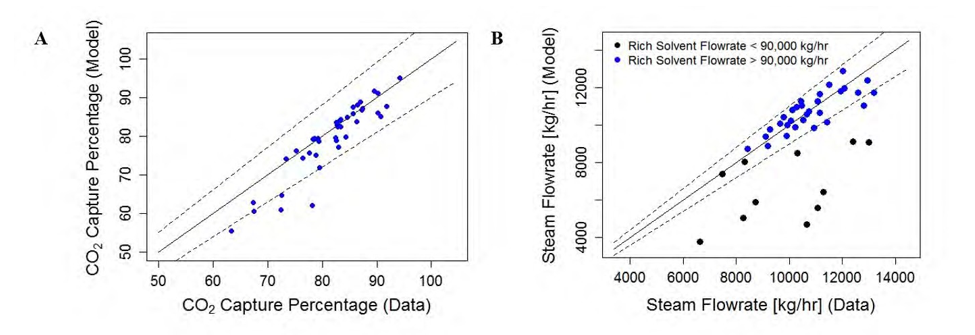
Figure 5. Parity plots for (A) CO2 capture percentage and (B) steam flowrate required for test runs performed in Phase 3. Dashed lines represent ±10% error.
The average percentage error values for the model predictions of the data collected in Phase 3 are -2.91 ± 5.27% for CO2 capture percentage and -8.53 ± 17.20% for the steam flowrate. As with the data collected in Phase 1, there is greater discrepancy in the stripper model for cases in which solvent flowrate is low; the average percentage error in the steam requirement is -31.05 ± 17.81% for cases in which the solvent flowrate is below 90,000 kg/hr and -0.27 ± 6.04% when it exceeds 90,000 kg/hr. As previously suggested, the underprediction in steam flowrate is likely due to operation inefficiency of the RFCC stripper caused by solvent maldistribution, as the process is operated at much lower solvent flowrate than the stripper design condition.
The probability density functions of the mass transfer and interfacial area parameters, including the prior and posterior distributions obtained after each SDoE iteration, are given in Fig. 6.
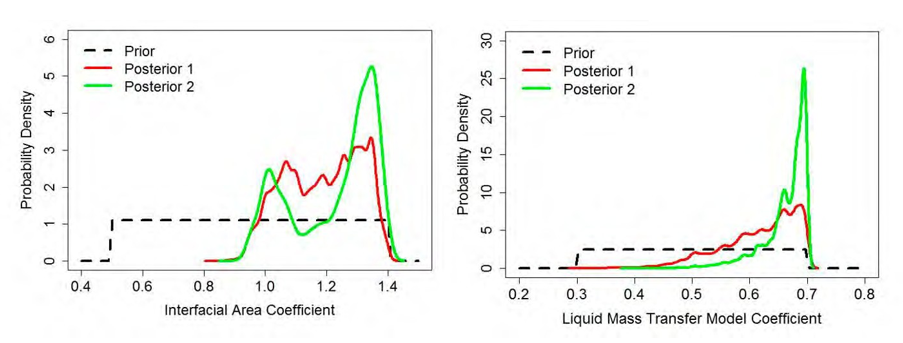
Uniform prior distributions were initially chosen for the two parameters. The parameter space of plausible values was significantly reduced after incorporating the experimental data from the first iteration of SDoE into the stochastic model through Bayesian inference, with less reduction in the second round of SDoE. The effect of SDoE on model uncertainty reduction is more apparent when considering the model output, namely the CO2 capture percent in the absorber. The effect of the first iteration of SDoE on reducing model prediction of uncertainty in CO2 capture percentage is shown in Fig. 7.
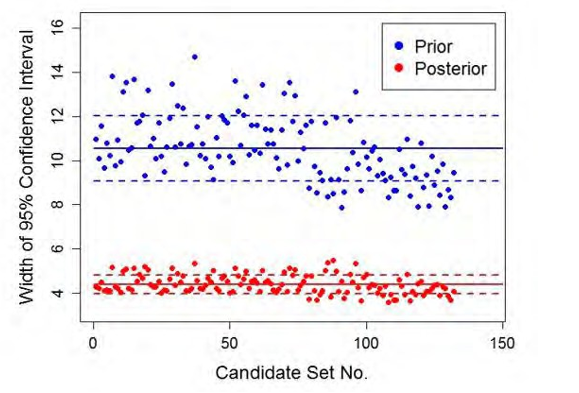
Figure 7. Effect of first round of Bayesian inference on CO2 capture prediction confidence interval for individual points in candidate set.
For the stochastic model prediction using the uniform prior distributions, the average confidence interval width for the CO2 capture percentage was approximately 10.5% (denoted in Figure 7 by solid line) with standard deviation 1.5% (denoted by dashed lines). For the stochastic model prediction with the posterior distribution obtained after the first iteration of SDoE, the average confidence interval width was approximately 4.4% with standard deviation 0.4%. No further significant reduction in the predicted uncertainty in CO2 capture percentage was demonstrated in the second round of SDoE. In Figure 7, the candidate set number refers to an index representing a unique combination of input variables (liquid and gas flowrates, CO2 loading, and CO2 fraction in flue gas). The percentage of reduction in uncertainty for a given point (𝒙𝒙(𝒊𝒊)) in the candidate set is calculated as:

where [𝐶𝐶𝐶𝐶|𝒙𝒙(𝒊𝒊) ]𝑖𝑖𝑖𝑖𝑖𝑖𝑡𝑡𝑖𝑖𝑡𝑡𝑡𝑡 and [𝐶𝐶𝐶𝐶|𝒙𝒙(𝒊𝒊) ]𝑓𝑓𝑖𝑖𝑖𝑖𝑡𝑡𝑡𝑡 represent the 95% confidence intervals in the model prediction of CO2 capture percentage before and after updating the parameter distributions through Bayesian inference, respectively. For the entire candidate set, the average percent reduction in the uncertainty is 58.0 ± 4.7%, which is comparable to the reduction in the previous SDoE-based test campaign executed at NCCC [5,6]. As the ability of the SDoE methodology to reduce parametric uncertainty in a process model for an aqueous MEA system has been demonstrated in multiple campaigns, it may be considered a promising technique for designing future test campaigns to effectively increase fundamental understanding of novel CO2 capture systems.
4. Conclusions and Future Work
In summary, a sequential design of experiments methodology was implemented for executing a test campaign for aqueous MEA at TCM, guiding collection of process data to refine the parameter distributions in the stochastic process model. This resulted in an average reduction of around 58% in the uncertainty in the prediction of CO2 capture percentage. The deterministic model, or the model without parameter uncertainty, also predicted the plant performance accurately, with an average error in percentage of CO2 capture of -2.74 ± 4.47% for the first three phases and an average error of -8.52 ± 14.85% for the reboiler steam requirement. An exception to the accurate performance of the model is for data collected under impractical operating conditions (low solvent circulation rate, in which solvent maldistribution in the stripper column was noted). For data collected when the system was operated with rich solvent flowrate below 90,000 kg/hr, the percent error in the reboiler steam prediction was -23.92 ± 15.70%. However, the percentage error in the steam prediction is -1.17 ± 6.65% for data collected when the rich solvent flowrate was above 90,000 kg/hr. The insights gained during the execution of SDoE guided the development of a new SDoE module with capability for straightforward implementation of the aims used in this experiment [16] that has been implemented in the Framework for Optimization, Quantification of Uncertainty, and Surrogates (FOQUS). This is available as part of the aforementioned CCSI Toolset and will enable the SDoE process to be implemented in a more streamlined manner in future applications. In planned future work, the CCSI2 team will apply the SDoE methodology to novel CO2 capture technologies with the primary goal of refining initial process models by reducing their uncertainty, and thus the inherent risk associated with preliminary models of new processes, through guided data collection.
Disclaimer
This project was funded by the United States Department of Energy, National Energy Technology Laboratory, in part, through a site support contract. Neither the United States Government nor any agency thereof, nor any of their employees, nor the support contractor, nor any of their employees, makes any warranty, express or implied, or assumes any legal liability or responsibility for the accuracy, completeness, or usefulness of any information, apparatus, product, or process disclosed, or represents that its use would not infringe privately owned rights.
Reference herein to any specific commercial product, process, or service by trade name, trademark, manufacturer, or otherwise does not necessarily constitute or imply its endorsement, recommendation, or favoring by the United States Government or any agency thereof. The views and opinions of authors expressed herein do not necessarily state or reflect those of the United States Government or any agency thereof.
Acknowledgement
The authors gratefully acknowledge the staff of TCM DA, Gassnova, Equinor, Shell and Total for their contribution and work at the TCM DA facility. The authors also gratefully acknowledge Gassnova, Equinor, Shell, and Total as the owners of TCM DA for their financial support and contributions.
References
- Morgan JC, Bhattacharyya D, Tong C, Miller DC. Uncertainty quantification of property models: methodology and its application to CO2 loaded aqueous MEA solutions. AIChE J 2015;61(6):1822-39.
- Morgan JC, Chinen AS, Omell B, Bhattacharyya D, Tong C, Miller DC. Thermodynamic modeling and uncertainty quantification of CO2 loaded aqueous MEA solutions. Chem Eng Sci 2017;168:309-24.
- Chinen AS, Morgan JC, Omell B, Bhattacharyya D, Tong C, Miller DC. Development of a rigorous modeling framework for solvent-based CO2 capture. Part 1: hydraulic and mass transfer models and their uncertainty quantification. Ind Eng Chem Res 2018;57:10448-63.
- Morgan JC, Chinen AS, Omell B, Bhattacharyya D, Tong C, Miller DC. Development of a rigorous modeling framework for solvent-based CO2 capture. Part 2: steady-state validation and uncertainty quantification with pilot plant data. Ind Eng Chem Res 2018;57:10464-81.
- Soepyan B, Anderson-Cook CM, Morgan JC, Tong CH, Bhattacharyya D, Omell BP, Matuszewski MS, Bhat KS, Zamarripa MA, Eslick JC, Kress JD, Gattiker JR, Russell CS, Ng B, Ou JC, Miller DC. Sequential design of experiments to maximize learning from carbon capture pilot plant testing. Comput Aided Chem Eng 2018;44:283-8.
- Morgan JC, Chinen AS, Anderson-Cook C, Tong C, Carroll J, Saha C, Omell B, Bhattacharyya D, Matuszewski M, Bhat KS, Miller DC. Development of a framework for sequential Bayesian design of experiments: application to a pilot-scale solvent-based CO2 capture process. App Energy 2020;262:114533.
- Brigman N, Shah MI, Falk-Pedersen O, Cents T, Smith V, De Cazenove T, Morken AK, Hvidsten OA, Chhaganlal M, Feste JK, Lombardo G, Bade OM, Knudsen J, Subramoney SC, Fostås BF, de Koeijer G, Hamborg ES. Results of amine plant operations from 30 wt% to 40 wt% aqueous MEA testing at the CO2 Technology Centre Mongstad. Energy Procedia 2014;63:6012-22.
- Gjernes E, Pedersen S, Cents T, Watson G, Fostås BF, Shah MI, Lombardo G, Desvignes C, Flø NE, Morken AK, De Cazenove T, Faramarzi L, Hamborg ES. Results from 30 wt% MEA performance testing at the CO2 Technology Centre Mongstad. Energy Procedia 2017;114:1146- 57.
- Faramarzi L, Thimsen D, Hume S, Maxon A, Watson G, Pedersen S, Gjernes E, Fostås BF, Lombardo G, Cents T, Morken AK, Shah MI, De Cazenove T, Hamborg ES. Results from MEA testing at the CO2 Technology Centre Mongstad: verification of baseline results in 2015. Energy Procedia 2017;114:1128-45.
- Hamborg ES, Smith V, Cents T, Brigman N, Falk-Pedersen O, De Cazenove T, Chhaganlal M, Feste JK, Ullestad Ø, Ulvatn H, Gorset O, Askestad I, Gram LK, Fostås BF, Shah MI, Maxson A, Thimsen D. Results from MEA testing at CO2 Technology Centre Mongstad. Part II: Verification of baseline results. Energy Procedia 2014;63:5994-6011.
- Montañés RM, Flø NE, Nord LO. Dynamic process model validation and control of the amine plant at CO2 Technology Centre Mongstad. Energies 2017;10:1527.
- Bui M, Flø NE, De Cazenove T, Mac Dowell N. Demonstrating flexible operation of the Technology Centre Mongstad (TCM) CO2 capture plant. Int J Greenh Gas Con 2020;262:114533.
- Myers RH, Montgomery DC, Anderson-Cook CM. Practical design optimality. In: Response surface methodology: process and product optimization using design experiments. 4th ed. New York: Wiley Plus; 2016. p. 467-74.
- Myers RH, Montgomery DC, Anderson-Cook CM. The one-half fraction of the 2k design. In: Response surface methodology: process and product optimization using designed experiments. 4th ed. New York: Wiley Plus; 2016. p. 162-174.
- Johnson ME, Moore LM, Ylvisaker D. Minimax and maximin distance designs. J Stat Plan Infer 1990;26:131-48.
- Lu L, Anderson-Cook CM, Ahmed T. Non-uniform space-filling designs. J Qual Tech 2021; to appear.
Cost Reduction Study for MEA based CCGT Post-Combustion CO2 Capture at Technology Center Mongstad (2021)
Muhammad Ismail Shaha, Eirik Falck da Silvab,*, Erik Gjernesc and Knut Ingvar Åsend
aTechnology Centre Mongstad, 5954 Mongstad, Norway bStavanger Research Center, TOTAL EP Norge AS, 4029 Stavanger, Norway cGassnova SF, Dokkvegen 10, 3920 Porsgrunn, Norway dEquinor ASA, PO Box 8500, 4035 Stavanger, Norway *Corresponding author
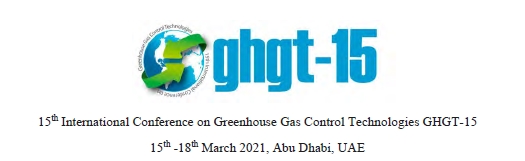
Abstract
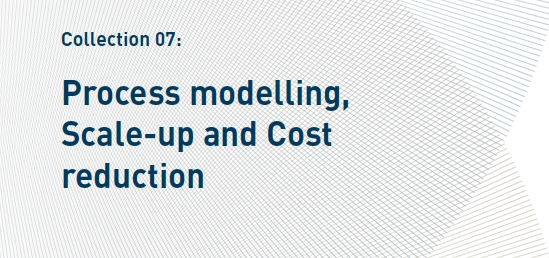
A campaign was carried out by Technology Center Mongstad (TCM) and its owners in the amine capture plant to study various cost saving operating options relevant for combined cycle gas turbine plants. The owners chose MEA as solvent for this test because it is a well-studied solvent system with no commercial constraints regarding sharing of data. Learnings obtained from MEA studies are also expected to be of relevance for other solvent systems.
During this campaign the main focus was on thermal energy optimization at different flue gas flow rates through the absorber column and MEA emissions, with target for reduced CAPEX and OPEX. During the campaign, new options such as a rich solvent bypass to stripper overhead and higher concentration of MEA (up to 38wt%) were tested. Tests were carried out to identify plant configurations and process parameters with the potential for CAPEX and OPEX reduction in a post-combustion carbon capture plant. Significant cost of CO2 avoided reductions were achieved compared to the previous TCM campaigns for MEA.
1. Introduction
TCM is located next to the Equinor refinery in Mongstad (outside Bergen, Norway) which is the source of the two types of flue gases supplied to TCM. These sources are the combined cycle gas turbine (CCGT) based heat and power plant (CHP) and residual fluid catalytic cracker (RFCC). The owners of TCM started their third monoethanolamine (MEA) test campaign (MEA-3) in June 2017 and continued with MEA-4 and MEA-5 that lasted until October 2018. MEA-3, MEA-4 and MEA-5 have been the most significant collaboration campaigns that TCM has conducted since its inauguration in 2012. The large number of industrial, research and academic participants involved in these campaigns have enriched the projects and ensured that the results will serve a broad range of audiences.
A sub-campaign on cost reduction during MEA-5 was carried out by TCM owners in this capture plant to study various cost saving options. MEA was chosen as solvent for this test because it is a well-studied solvent system with no commercial constraints regarding sharing of data. This work follows previous work carried out on the same subject at TCM [1].
2. Test Campaign and Results
The TCM amine plant is shown in Figure 1. The plant has been in operation since 2012 carrying out tests on a number of proprietary and non-proprietary solvent systems. A recent modification to the plant is the addition of a rich solvent partial-bypass upstream of the lean-rich amine heat exchanger to the stripper top. This is intended to improve the energy performance of the plant. A noteworthy feature of the plant is that there are three different feed locations for the lean solvent to the absorber. This allows for testing at different packing height for CO2 absorption (12m, 18m, and 24m), as shown in yellow in the Figure. Another important feature is that the plant has two strippers with dedicated reboilers, the Combined Heat and Power (CHP) stripper suited for stripping of lower amounts of CO2 and the Residual Fluidized Catalytic Cracker (RFCC) stripper suited for larger volumes of CO2. Only CHP flue gas was utilized in the present campaign.
The purpose of the present campaign has been to explore different modes of operation with cost reduction potential. Such as higher flue gas throughput, lower packing height, more concentrated solvent and higher capture rate. Simple configurations such as operating with only one water wash section and higher flue gas inlet temperatures were also investigated. Table 1 summarizes the phases A to F of the test campaign.
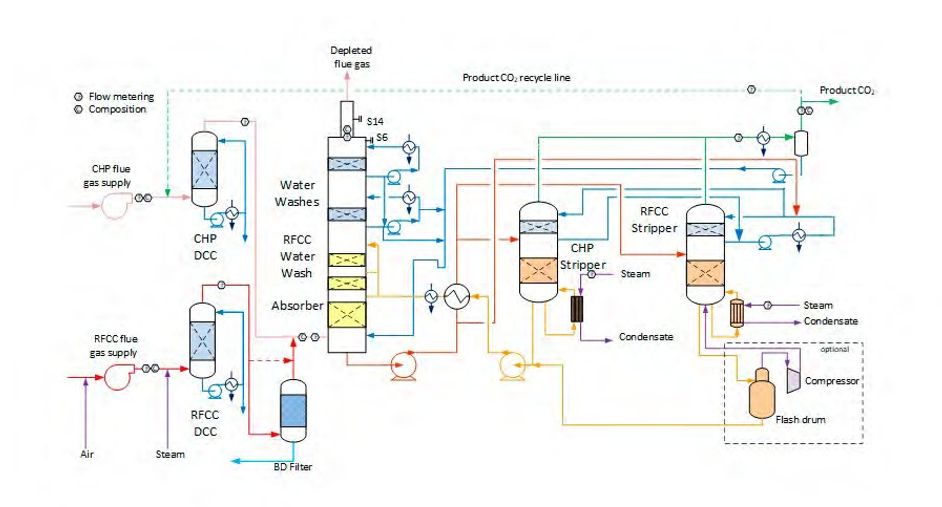
Fig. 1. A process flow diagram of the TCM Amine plant. The diagram illustrates the two different flue gas configurations (CHP and RFCC) as well as the available strippers. The CHP flue gas can be further enriched with CO2 from a CO2 recycle line, and the RFCC flue gas can be diluted with air to reach a target CO2 concentration.
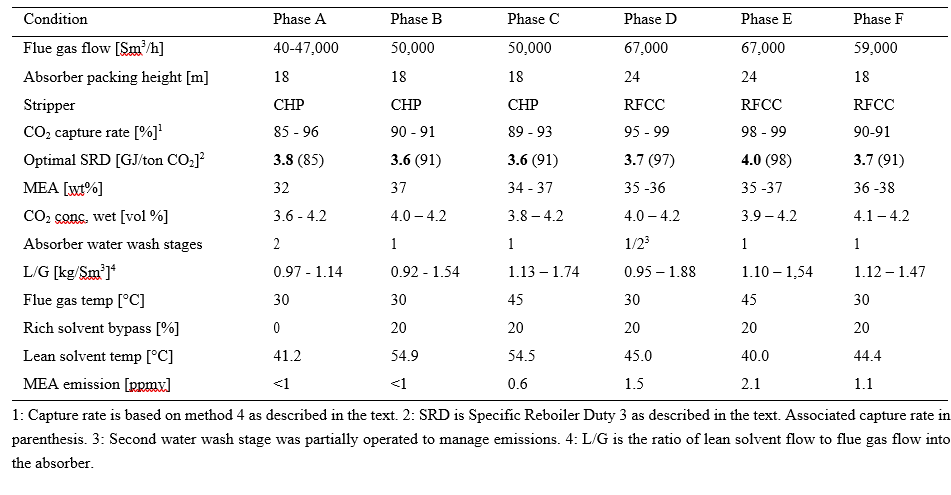
The rich solvent bypass was in operation during all phases of the campaign except phase A. Flue gas CO2 concentrations varied between 3.6 % and 4.2% during the campaign. The variations in CO2 concentration were achieved by use of a recycle. Phase A was operated at 32 wt% MEA and was investigating effect of CO2 concentration and flue gas flow.
Phase A was mainly intended to validate previous work and will not be discussed further. The rest of the cases were operated at MEA concentration up to 38 wt% and with the target of having only the lower absorber water wash section in operation.
Phase B and C were operated at 50,000 Sm3/h, 18-meter absorber packing, CHP stripper and with flue gas temperature out the absorber of 30 and 45°C, respectively. Operating with higher flue gas temperature could offer potential savings since this will enable the use of a smaller Direct Contact Cooler.
During Phase D and E this temperature variation was intended to be repeated at flue gas flow of 67,000 Sm3/h, 24- meter absorber packing and RFCC stripper in operation. The flue gas flow of 67,000 Sm3/h rate is the highest practically possible operating point at TCM and represents 113% of design capacity.
Finally, Phase F was operated at 18-meter absorber packing and 59,000 Sm3/h flue gas flow at 36-38wt% MEA.
The energy efficiency of the capture process is given by the Specific reboiler duty (SRD), this is defined as the heat delivered to the reboiler from the steam system divided by the amount of captured CO2:
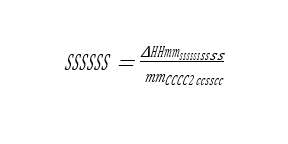
where 𝑚𝑚steam is the steam flow to the reboiler heat exchanger. ∆𝐻𝐻 is the enthalpy difference between steam and condensate calculated from measured temperature and pressure, see also reboiler, steam and condensate in Figure 1. Steam pressure is typical around 2.5 barg and up to 160 °C for the tests reported in this paper. CO2 capture rate is the mass fraction of CO2 being captured out of the amount of CO2 flowing into the absorber. The amount of captured CO2 can be derived from the CO2 product flow out of the stripper or from difference in CO2 flow in and out of the absorber. SRD and capture rate are presented utilizing both methods below, while the economical assessment is based on difference in and out of the absorber such that the results can be compared to previous work [1]. This method is in the present paper referred to as “SRD 3”. SRD based on the product flow out of the stripper is referred to as “SRD 1”. The reported results are two-hour averages within a test slot that lasts more than 6 hours. Typically, one liquid solvent sample is taken for each test point.
In the present paper we report CO2 capture rates based on two methods, Capture Method 1 and Capture Method 4. Method 1 is based on the ratio between the stripper product flow and the absorber inlet flow, while Method 4 is based on the mass balance over the absorber [2].
TCM is equipped with multiple flue gas analyzers and flow meters for each of the main three gas streams. Table 2 shows the ones selected for the current analysis. Water is calculated assuming saturated conditions based on pressure and temperature. Flow out of the absorber is calculated from measured flow into the absorber assuming conservation of all components in and out of the absorber except water and CO2. Finally, it is assumed that product gas out of the stripper is only CO2 and water.
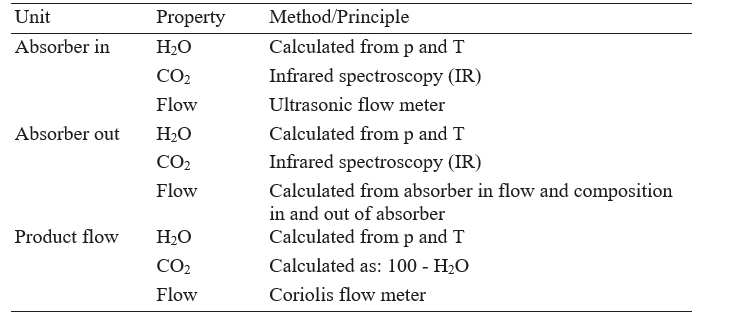
Table 2. Measurement of gas flows.
In Figure 2 SRD and capture rate vs. L/G for phase B is shown. At TCM optimum SRD is obtained by varying the lean flow rate (and thereby the L/G) and adjusting the reboiler duty at each solvent circulation point to maintain the required/targeted capture rate. The optimum SRD achieved here was 3.6 GJ/ton CO2 (based on SRD 3).
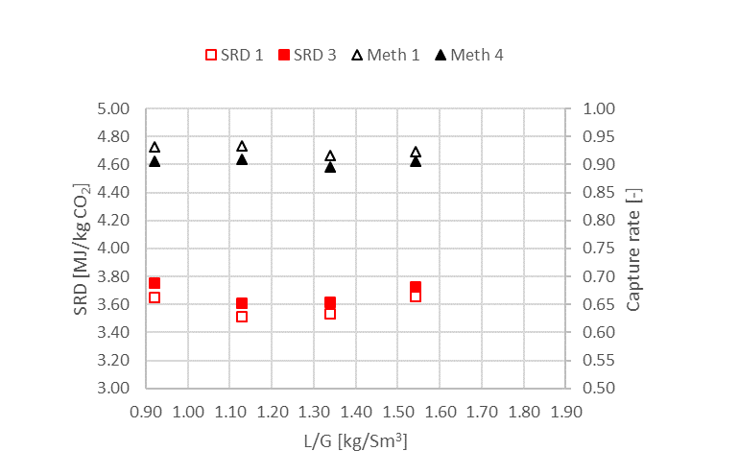
Fig. 2 SRD (left y-axis) and capture rate (right y-axis) for phase B as function of liquid to flue gas ratio (flue gas 50,000 Sm3/h).
One of the best cases demonstrated is “B3-rep” (at L/G of 1.13). The capture rate for this case is a bit above 90%, with SRD of 3.6 GJ/tCO2 with 18m packing height, 37 wt% MEA, one water wash and MEA emissions below 1 ppm. Flue gas flow is 50,000 Sm3/h and liquid to gas ratio (L/G) for “B3-rep” is about 1.13 kg solvent/Sm3 of flue gas. This can result in significant reduction of CAPEX and OPEX, compared to the MEA baseline.
In Figure 3 SRD and capture vs. L/G for phase D is shown. During Phase D 97% CO2 capture at an SRD of 3.7 GJ/ton CO2 were achieved. This SRD is perhaps lower than that would have been expected for a very high capture rate. The point at L/G of 1.38 has a high SRD, this is due to high levels of steam being utilized to achieve high capture rates. With Method 4 the capture rate was 99%, with Method 1 it was slightly over a 100% (due to accuracy limitations in calculation). Since the number is over 100% it is not visible in the plot.
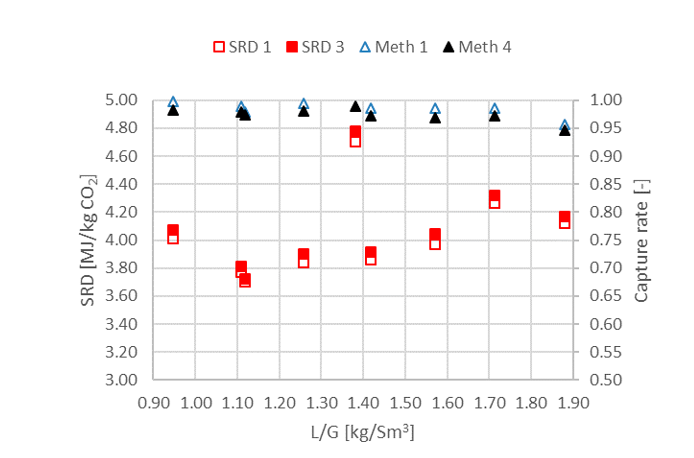
Fig. 3. SRD (left y-axis) and capture rate (right y-axis) for phase D as function of liquid to flue gas ratio (flue gas 67,000 Sm3/h).
Higher flue gas capacity tests at 67,000 Sm3/h resulted in high amine emission, which is partly due to mechanical entrainment and partly due to only one water wash section being in operation. However high solvent entrainment can be potentially reduced by improving the absorber design by installing a more efficient demister at appropriate location in the absorber. Very high CO2 capture rate of almost 99% was demonstrated with SRD of 3.8 GJ/tCO2 with 24 m absorption bed and 67,000 Sm3/h of flue gas capacity. The increase in SRD is about 6% when the capture rate increases from 90% to 99%, however the comparison is not fair as the plant capacity and configuration is not similar in the two cases.
During phase E higher flue gas capacity tests at 67,000 Sm3/h and flue gas temperature of 45°C resulted in a relatively high SRD and most importantly higher MEA emission as expected.
SRD and capture results from Phase F are shown in Figure 4. Case F2 with 3.8 GJ/ton CO2 and 90% capture rate was selected for further assessment in the next section.
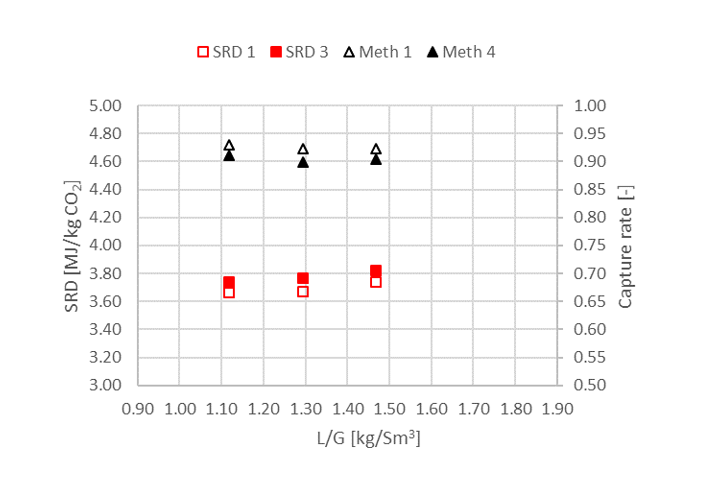
Fig. 4. SRD (left y-axis) and capture rate (right y-axis) for phase F as function of liquid to flue gas ratio (flue gas 59,000 Sm3/h).
In the present campaign the plant was mostly operated with 37 wt% MEA. There have been some relatively short campaigns at TCM in the past where the plant has operated with a concentration slightly higher than 40 wt% MEA [1]. From an energy performance perspective 40 wt% is expected to give better results. There is however a concern that solvent degradation will be more severe for 40 wt% MEA. Degradation can besides being a problem in itself also impact corrosion in the plant. 35 wt% MEA is what TCM is currently comfortable with operating, however long-term continuous test with 37-38 wt% MEA needs to be conducted to better understand degradation, emission and energy performance of the solvent.
Table 3 summarizes the selected cases for economical evaluation along with the reference case named MEA 3 (9-
4) [1]. Stripper pressures were maintained at 0.9 barg for all cases. The MEA-3 and B3-rep were operated with CHP stripper while F2 and D3-rep utilized the RFCC stripper. Liquid sample for case D3-rep is taken about 1 hour outside the 2 hours being averaged, but still within the test period.
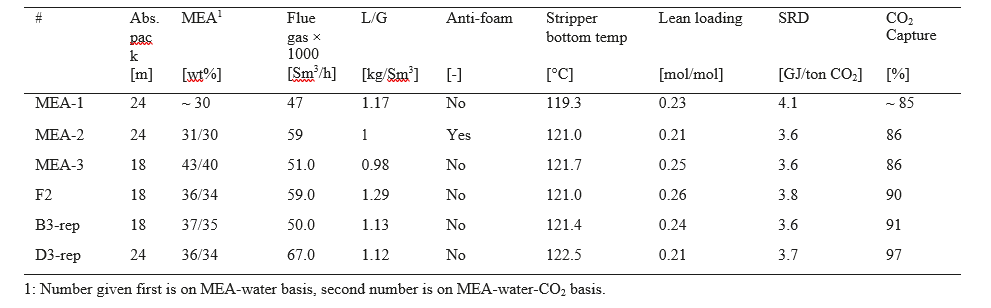
Table 3. Selected cases for economical evaluation [1-3]
3. Economic Evaluation
The economic evaluations of power and capture plants in this paper is based on standard “Cost of Electricity” (COE) and “Cost of CO2 avoided” metrics. These calculations are based on aligned and standardized estimates and assumptions on technology process performance such as energy efficiency, CO2 generation and capture rates, see e.g. [4]. Cost estimates include CAPEX, operations and maintenance (O&M) including fuel and a set of general price and rate of return assumptions. For each case below, a complete sized capture plant equipment list is established. Aspen In-Plant Cost Estimator (IPCE) V9 is used to estimate equipment cost. Equipment installation factors are then used to estimate total installed costs. Aligned with known projects, including contingency, 30% added to the Aspen Equipment cost and average installation factor of around 5.5. The OPEX can be split in annual cost (of CAPEX), power loss, maintenance, chemicals and fixed operating costs. The gas fired power plant specific cost and performance is based on GTPro simulation of a GE 9HA CCGT plant. 25% contingency is added to the estimated GTPro CCGT CAPEX number. All calculations are furthermore carried out at:
- normalized, per unit (kWh) output from the base industrial (power) plant
- pretax, pre-financing basis
- annual cost basis, applying a capital charge factor corresponding to a standard discount factor and project time horizon
Cost of CO2 avoided ($/ton CO2) is calculated according to Equation below and is based on cost of electricity (COE) and CO2 emission per kWh (CO2 emission) for a power plant with capture (cap) and without CO2 capture (no cap).

The calculated cost of CO2 avoided implicitly accounts for the capture systems’ own energy demand and its
inherent CO2 emissions. The following economic assumptions are applied:
- Fuel gas price: 0.1875 US $/Sm3
- On-stream hours: 7884 (90 %)
- Discount rate: 5 % real (pretax)
- Time horizon: 30 years
These were chosen to be consistent with a previous economical study [1].
This paper will only report percentage cost reduction and no absolute cost numbers. The main reasons are that the absolute numbers are not useful for the purpose of this work and are partially confidential. In this work one consistent method and one consistent set of assumptions are used for calculating the cost, which is important for a fair comparison.
The experiments targeted lowest possible absorber packing height, lowest possible L/G and SRD while maximizing the captured CO2 and capture rate. In Table 4 below the experimental data for the selected cases are scaled to a full- scale design at a fixed inlet CO2 flow of 150 tonnes CO2/h and measured capture rate case by case.
In order to compare on the same basis in the cost assessment, the CO2 inlet concentrations for all cases are adjusted to 4.2 % (wet) since the tests was done with close to 4.2 % (wet) CO2 in the flue gas. Typically, an H class gas-turbine will produce flue gas with at least 4.5% CO2 which will reduce the CO2 capture cost further. An increased CO2 content will impact all the cases in this paper equally (see discussion in previous paper [1]).
The adjusted/scaled absorber packing height and the most important cost parameter, the packing volume, are calculated from the experimental data for the cases selected in the test campaign. The scaled-up absorber volume is based on packing height utilized for each TCM test case and a scaled up cross sectional area. The latter is calculated based on TCM cross sectional area and the ratio of full-scale (150 tonnes CO2/h, corresponding to 700 MWlhv fuel input) to TCM (case by case) CO2 inlet flow.
For all scaled up cases the cross-sectional areas are adjusted to fit with a superficial velocity of 2 m/s (at 0 °C, 1 atm). This will secure less differences in pressure drop between the cases and less impact on the flue gas fan duty in a full-scale plant. This means that it is assumed that the CO2 capture rate depends mainly on the total packing volume and less on the differences in flue gas velocity through the absorber.
Thus, packing height, see Table 4, is adjusted in order to maintain the scaled-up absorber packing volume. The packing volume per captured CO2 will be equal for each TCM case and corresponding scaled up case. The data are shown in Table 4 including lean solvent flow rate, specific packing volume, amount of captured CO2 and CO2 capture rate.
Packing volume is a significant CAPEX element and the most cost-effective packing volume demonstrated in this campaign was 34 m3/tonne CO2 capture per hour for the current test conditions. In Case B3-rep and D3-rep a significantly larger stripper (RFCC) was used removing any limits in the stripper process. This had a positive effect in allowing operating with a lower inlet lean loading at an increased solvent flow rate to the absorber (i.e. keeping an optimal L/G) increasing the absorber CO2 capture capacity and significantly increasing the CO2 capture rate.
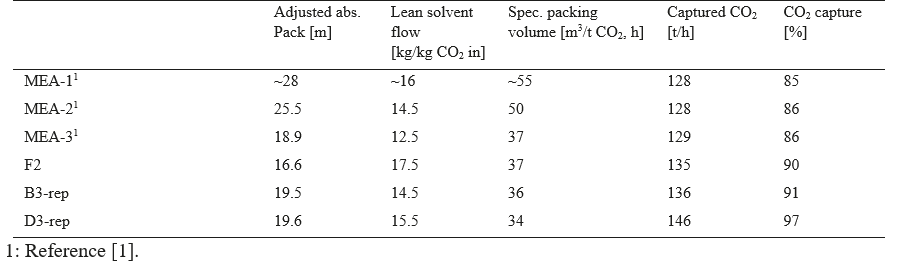
Table 4. Key cost parameters.
The section above introduced the economic evaluation and cost of CO2 avoided. In Figure 5 the demonstrated cost reduction for the selected three test cases are compared with the best result from the MEA-3, 2 and 1 campaign [1]. The previous assessment [1] has been revisited and the presented relative cost of CO2 avoided are updated based a newer gas turbine (H class).
Case D3-rep demonstrates the largest cost reduction contribution, i.e. 4.8 % down relative to MEA-3 and close to 20% relative to MEA 1 The trend is a reduction in CO2 capture cost with increasing CO2 capture rate. Since more CO2 is being captured for a given flue gas stream the total cost (CAPEX) of CO2 handling will increase somewhat also increasing cost of electricity. For case D3-rep this represents an increased cost of electricity with 3-4% compared to the MEA-3 case. Due to the significantly higher amount of CO2 captured, the avoided CO2 cost will still be reduced as shown in Figure 5 below. The cost estimation was done with two water wash stages for the case D3-rep. Results from the campaign did suggest that one stage could have been sufficient, resulting in some additional savings.
Since the cost reduction potential of these measures is experimentally verified in one of the world’s largest demonstration plants, the cost reduction should be highly accurate, and hence relevant for future post-combustion plants.
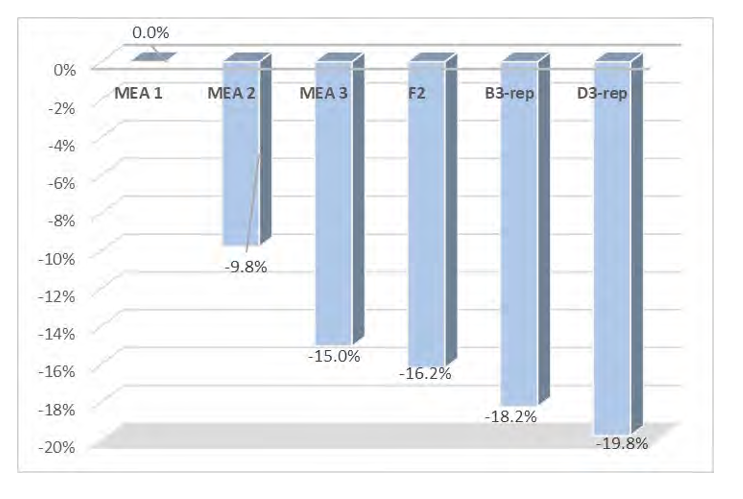
Fig. 5. Demonstrated reduction in cost of CO2 avoided for the selected cases compared with the earlier MEA campaigns.
4. Conclusions
The results show encouraging cost reduction potential such as lower energy numbers for a more concentrated (35 wt%) MEA solvent and more cost-efficient design of the capture plant. Cost improvements of 4.8% were achieved compared to the previous TCM benchmark (MEA 3) and close to 20% compared to the first campaigns. These results can be viewed as a form of debottlenecking of the amine plant, optimizing its throughput. However, the impact of working with a more concentrated solvent on corrosion, solvent degradation and emissions is something that must be explored further.
It is important to notice that these results are generated at one of the world’s largest capture demonstration units, and that it is one of the first times such a structured campaign is executed. Similar testing can be carried out with different amine-based solvents. Therefore, these results at TCM scale represent a very relevant basis for scale up and industrial design of amine solvent capture technologies.
Acknowledgements
The authors gratefully acknowledge the staff of TCM DA, Gassnova, Equinor, Shell and Total for their contribution and work at the TCM DA facility. The authors also gratefully acknowledge Gassnova, Equinor, Shell, and Total as the owners of TCM DA for their financial support and contributions.
References
- Gjernes E, Pedersen S, Jain D, Åsen KI, Hvidsten OA, de Koeijer G, Faramarzi L, de Cazenove T. Documenting modes of operation with cost saving potential at the Technology Centre Mongstad. GHGT-14, 21st-25th October 2018, Melbourne, Australia
- Faramarzi F, Thimsen D, Hume S, Maxon A, Watson G, Pedersen S, Gjernes E, Fostås BF, Lombardo G, Cents T, Morken AK, Shah MI, Cazenove Td, Hamborg ES. Results from MEA testing at the CO2 Technology Centre Mongstad: Verification of baseline results in 2015. Energy Procedia, 114, 2017, 1128-1145
- Hamborg ES, Smith V, Cents T, Brigman N, Falk-Pedersen O, de Cazanove T, Chhagnlal M, Feste JK, Ullestad Ø, Ulvatn H, Gorset O, Askestad I, Gram LK, Fostås BF, Shah MI, Maxson A, Thimsen D. Results from MEA testing at the CO2 Technology Centre Mongstad. Part II: Verification of baseline results. Energy Procedia 63, 2014, 5994-6011
- Cost and Performance Baseline for Fossil Energy Plants Volume 1a: Bituminous Coal (PC) and Natural Gas to Electricity Revision 3, July 6 2015, DOE/NETL-2015/1723
A Benchmark for Compact CO2 Capture Plant Designs by Monoethanolamine Solvent Testing at Technology Centre Mongstad (2021)
Gelein de Koeijera, Christophe Benquetbc, Anette Knarvikac, Erik Gjernesd, Steinar Pedersena, Odd Arne Hvidstenac
aEquinor ASA, P.O. Box 8500, 4035 Stavanger, Norway b Total E&P Norge, Finnestadveien 44, Dusavik, 4029 Stavanger, Norway cTechnology Centre Mongstad, 5954 Mongstad, Norway dGassnova SF, Dokkvegen 10, 3920 Porsgrunn, Norway
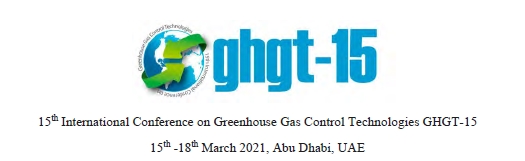
Abstract
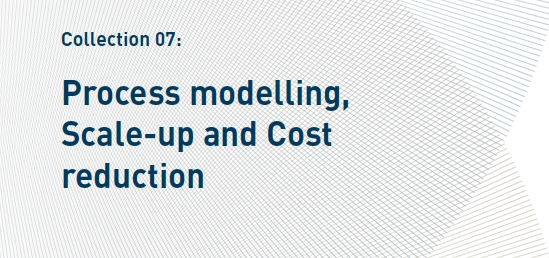
Technology Centre Mongstad (TCM) is a large and flexible demonstration site for post-combustion CO2 capture. It is located next to the Equinor refinery at Mongstad (Norway) which is the source of the flue gases supplied to TCM. It has been used for testing CO2 capture with MEA solvent and a compact design, providing a benchmark for compact CO2 capture technologies.. The absorber was used with its lowest possible packing height of 12m, and only one of two water washes was used (3m height). The plant was operated with a high flue gas flow rate (67,000 Sm3/h) and 35 wt% MEA with a sensitivity down to 30 wt%. The CO2 inlet concentration was 6% mimicking some industrial exhausts, small turbines with exhaust gas recycle or modern turbines with high turbine inlet temperatures. The tests demonstrated that such a low absorber can capture more than 80% of the CO2 with only a slightly higher steam demand than conventional applications with higher packing heights of 18-24m. The low absorber gave 3.9 MJ/kg CO2 for the specific reboiler duty, while previous tests at other conditions and with higher absorbers gave
3.5-3.7. Acceptable emissions were observed, while degradation was higher than earlier TCM campaigns due to the choice of running with 35 w% MEA. Overall, a benchmark has been provided for future improved compact capture technologies.
1. Introduction
Technology Centre Mongstad (TCM) is a large and flexible demonstration site for post-combustion CO2 capture. It is located next to the Equinor refinery at Mongstad (Norway) which is the source of the two types of flue gases supplied to TCM. These sources are the combined cycle gas turbine (CCGT) based heat and power plant (CHP) and residue fluid catalytic cracker (RFCC). TCM is owned by Gassnova (on behalf of the Norwegian state), Shell, Total and Equinor. Various proprietary amine solvents have been tested and matured at TCM since the start-up in 2012. In addition, various campaigns have been executed with a numbered series of open non-proprietary monoethanolamine (MEA). Most of the results have been published. The most significant campaigns were the third monoethanolamine test campaign (MEA-3) in June 2017 and the following MEA-4 and MEA-5 that lasted until October 2018. The large number of public, industrial, research and academic participants involved in these campaigns have enabled that the results served a broad scientific audience. The main objectives of these campaigns were to gain knowledge and information that can be used to reduce the cost as well as technical, environmental and financial risks of commercial scale deployment of post-combustion capture (PCC). This includes demonstration of a model-based control system, dynamic operation of the amine plant, investigating amine aerosol emissions, establishment of residue fluid catalytic cracker (RFCC) baseline performance with MEA, and specific tests targeted at experimentally verifying measures that can reduce the cost of CO2 avoided [1].
This paper describes a part of the MEA-5 campaign tests relevant for reducing the size of a CO2 capture plant as the most important driver. Reduced size can be beneficial or even enable CO2 capture in certain applications. One example is brownfield retrofit of capture on exhausts with very limited spaces, e.g. existing industry near urban areas and refineries. Another example are turbines or engines on offshore oil&gas production unit. Equinor is currently developing its 3CWI concept “CO2 Capture with Carbonated Water Injection” for greenfield Floating Production, Storage and Offloading (FPSO) units [2]. In this concept CO2 is captured from a gas turbine, compressed, mixed with produced and/or sea water and injected as dissolved bicarbonate ions. For these examples the main constraints is available space and equipment weight rather than the energy efficiency. As the absorber is the unit of largest mass and volume, experiments that can reduce the uncertainty at low absorber heights are of value for these applications. Not much specific research has been done yet with this motivation.
The tests in this paper are done at elevated CO2 concentration relative to earlier CHP based campaigns at TCM. 6 vol% was chosen, which reduces the size of the absorber relative to the usual 3.5-4 vol%. The reason for this is that elevated CO2 concentrations are likely in applications where weight and volume are restricted. One motivation for this choice is the possible use of exhaust gas recycle (EGR) both on turbines and piston engines. Another motivation is the gradual development of increasing the CO2 content due the higher turbine inlet temperatures in modern turbines allowing for less air cooling of the expander blades. In order to achieve 6 vol% of CO2 in the flue gas going to the capture plant, the TCM amine plant was operated with flue gas from the Mongstad CCGT plant and with recycling a portion of the captured CO2.
The absorber packing height was set at 12m, which is the lowest possible at TCM’s amine unit. Moreover, one of the two water washes was disabled. This combination simulates the lowest absorber setting possible at TCM, but has significant dry bed height in between. The results will be used for discussing the viability of making post-combustion capture more compact. It is acknowledged that the amine unit at TCM is not specifically designed for testing compact capture. Consequently, the result may not be representative for commercial use as the unit will operate outside the operational window TCM’s amine unit is designed for. The data can be utilized as a source for insight, comparison and for benchmarking commercial compact capture technologies.
2. System
TCM’s amine unit was used (see e.g. [4][5][6]) and its flexibility was utilized to collect data relevant for a compact capture plant design. This means that the absorber was operated with only the lower packing section (12 m) and with one water wash bed (3 m, Lower (L) in operation, Upper (U) is idle) in service. This is the smallest total amount of packing possible at TCM without modifications. The set-up is illustrated and compared to the full amine plant set-up in Figure 1.
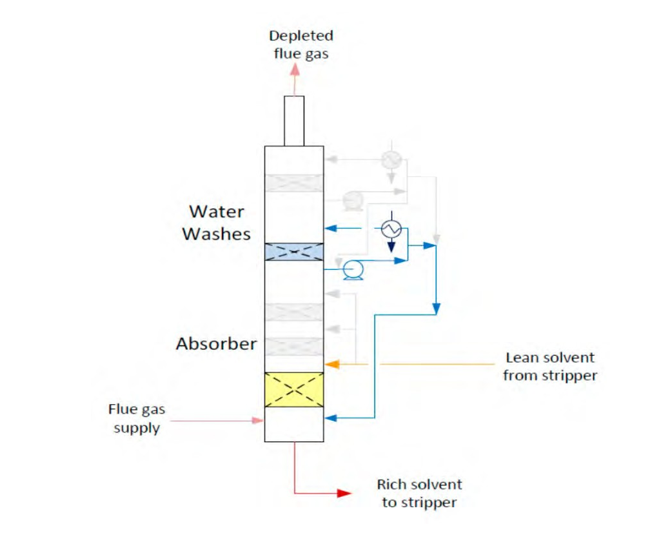
Figure 1. Illustration of the compact flue gas absorber set-up.
The flue gas flow rate was adjusted to its highest practically possible operating point (67,000 Sm3/h, 113% of design capacity). To mimic higher capacity solvent types, the MEA concentration was increased to 35 wt% from the normally used 30 wt%.
The amine plant has extensive instrumentation with multiple measurements on the streams required for performance assessment. Details on the CHP flue gas characteristics, the main systems in the plant and an assessment of instrumentation quality as well as calculation methods for the main parameters are described in previously published papers, see Gjernes et al [1][7].
3. Method
3.1 Strategy
For this work various test series were executed named by the first letters in the alphabet. The plant was operated with a high flue gas flow rate (67,000 Sm3/h). An overview of the test series is given in Table 1. The strategy in this work was to start out with the highest achievable capture rate in test series A. Therefore, the steam flow rate to reboiler was maximized and the solvent flow rate was high (106-138 kg/hr). The series B to D were systematic experiments to produce steam demand optimum curves (so-called U-curves) for identifying the lowest specific reboiler duty (SRD) for each series over a selected range of target capture rates (~ 83 to 72 %). In the E and F-series the flue gas temperature into the absorber was higher (45 instead of 30 °C), for assessing potential reduction in the cooling requirement and the equipment size of the direct contact cooler (DCC). As in the A series, the E cases were maximizing the steam flow rate to achieve a high capture rate and the F series was systematic experiments to find the energy optimum. The E case consists only of one test. All these series were run with around 35 w% MEA. Next, some tests were added to put the series A-F in a wider perspective. The cases named B5-1 and B5-2 are two reference runs with MEA at 30 wt% and with lower and upper water wash in operation, respectively. The motivation was to see whether there are differences between the upper and lower water washes. The case named 15-3 is included in the results section to have a comparison against the most compact absorber line-up used in the previous TCM study on modes of operation with cost saving potential back in 2018 [1]. The objectives for the experimental work were to investigate capture rates and specific reboiler duty during parameter testing. Steam flow to reboiler and solvent flow rates were the main variables, while flue gas flow and CO2 inlet concentration were kept constant.
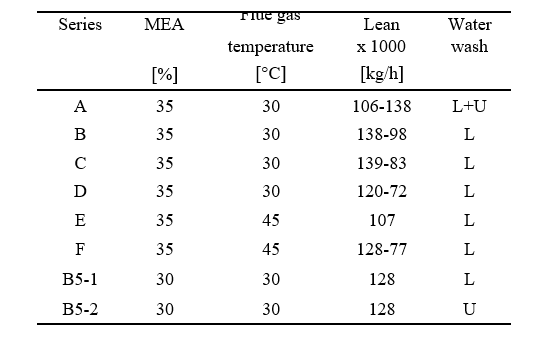
Table 1. Overview of the tests.
With this strategy, it was the intention to inspire, and stretch the targets for new technology developments of compact and low weight CO2 capture plants. With more advanced solvents and optimized systems, it is expected that significant improvements beyond what TCM demonstrates here are possible.
The so-called U-curves were produced by variations in the lean amine to gas flow rate ratio (L/G). The cold rich by-pass of the lean rich heat exchanger (17% by-pass) was in operation during all test runs. This variable was left unchanged. It is acknowledged varying it could provide somewhat lower steam demand or higher capture rate, but not significant.
3.2 Measurements
The combination of methods used in the current work is shown in Table 2. The flow out of absorber is based on flow into the absorber assuming all components except water and CO2 are conserved. Moisture is calculated from temperature and pressure. The product flow is assumed to be CO2 and moisture only.
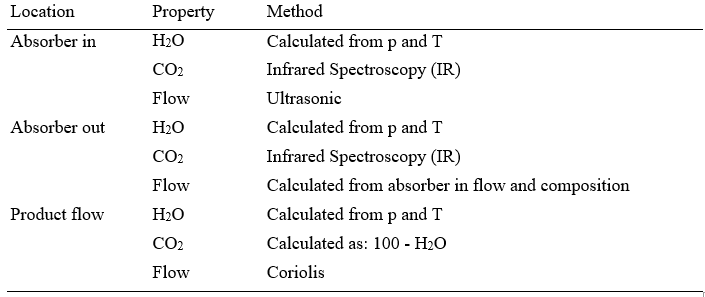
In each test run the plant is first allowed to stabilize over several hours. A set of key performance indicators is used to assess the quality of data. A two-hour stable period is selected for data extraction. Liquid sampling is conducted within this period. All properties except liquid samples (amine concentration and loading) are averaged over the two-hour period. Thus, reported data are based on stable operation with respect to all streams. The total and CO2 mass balances should be within 100 ± 2%. This procedure allows normally not more than two single test runs per day (24 hours). To make comparisons between the test runs, it is important to maintain stable MEA concentration (35 wt%, CO2 free) and the absorber inlet CO2 concentration (6 vol%, dry). MEA concentration, lean and rich loading are calculated from laboratory analysis of the liquid samples. In case there is a missing liquid sample, the lean loading is extrapolated using amine density (only for the following tests: B6-opt, C2, D4 and F2).
The solvent loading is calculated from total inorganic carbon (mole CO2/kg solvent) and total alkalinity (mole amine/kg solvent):
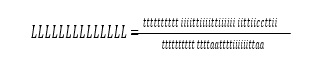
The specific reboiler duty (SRD) is the heat delivered to the reboiler from the steam flow divided by the amount of captured CO2:
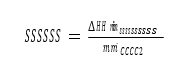
In this work the captured CO2 (𝑚𝑚̇ 𝐶𝐶𝐶𝐶2) is based on the difference in mass flow of CO2 over the absorber (𝑚𝑚̇ 𝐶𝐶𝐶𝐶2abs, in – 𝑚𝑚̇ 𝐶𝐶𝐶𝐶2abs, out). This is chosen in order to aligned with earlier published results (e.g. [1]). Captured CO2 (𝑚𝑚̇ 𝐶𝐶𝐶𝐶2) based on product flow from stripper will for the present cases result in a lower CO2 product flow. The steam pressure and temperature are typically around 2.5 barg and 160 °C in this work.
The capture rate is the mass fraction of captured CO2 and the amount of CO2 flowing into the absorber.

The absorber packing volume and thus the absorber size are major CAPEX elements. The absorber packing volume (m3/ton CO2, h) was calculated for the cases with highest CO2 product flow and compared with the previous test case 15-3. The parameter is one metric used for assessing compact absorber designs. The TCM absorber cross section is 7.2 m2 and packing height applied is 12 m.
The emissions of amine and ammonia were monitored during all test runs. As higher MEA concentration is associated with increased solvent degradation [3] and plant corrosion, degradation and metal content in the solvent were closely monitored. However longer-term testing is expected to be needed to assess the solvent management aspects of higher MEA concentrations. Moreover, the solvent composition was measured before and after for estimating the cumulative degradation.
4. Results and discussion
4.1 Capture rate and steam demand
The observed relation between SRD and capture rate is shown in Figure 2 for all the series A-F and the B5-2 and B5-1 with 30 w% MEA. The lowest SRD value in the U-curves achieved within each test series is shown as transparent squares along with a dashed trend line based on the five selected optimum points. The trend line shows a corresponding decrease in capture rate and SRD from 87% and 4.3 MJ/kg CO2 down to 73% and 3.7 MJ/kg CO2. Within each series the capture rates were quite well controlled within the target. The trend line gives a good indication of what is achievable with a compact plant configuration. The results from the F-series (82% capture rate) shows promise also for reducing cooling need and size of the DCC. It is also seen that the SRD is significantly higher for the two test runs with MEA 30wt% (B5-2 and B5-1). The uncertainty at TCM is discussed in other papers [4][5][6] and is assumed not to be significant different in this campaign.
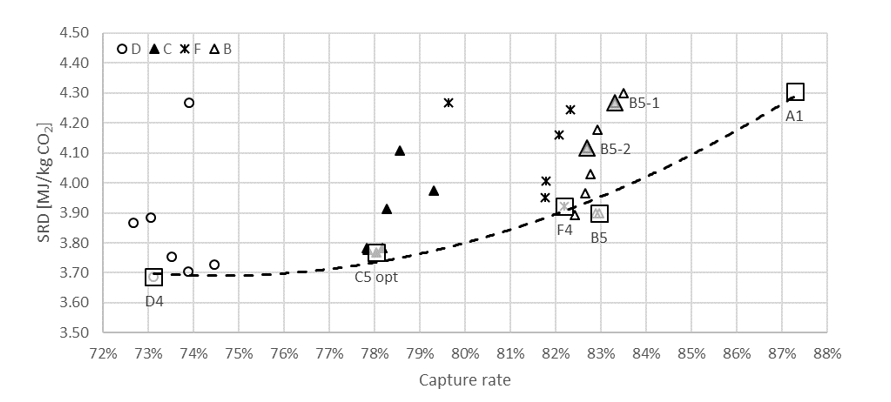
Figure 2. SRD and capture rate for the test series. Optimum points for each series are shown as transparent squares.
As expected with the low absorber height the SRD become higher than TCM has reported earlier for higher absorber heights. For 30 w% MEA Gjernes et al.[1] reports down to 3.6 MJ/kg CO2 at 86% capture with 3.6 vol% CO2 in the inlet, while this work shows around 4.0-4.2 MJ/kg CO2 at 87% capture with 6 vol% CO2 in the inlet. This increase may not be unacceptable high in situations where energy ample cheap heat is available. Figure 2 also shows that a similar low SRD of around 3.6-3.8 is achievable with a lower capture rate of 70-80%. This decrease may neither be unacceptable in the mentioned applications where space and weight are the limiting factors.
The MEA and wet CO2 inlet concentrations were aimed to be kept constant for all tests, and the achieved results are shown in Figure 3. There are small variations between the test series and the comparison between the series are representative. There is also good agreement between the two CO2 measurement methods. The figure also shows MEA concentration close to 31 wt% for the B5-1 and B5-2 cases and the CO2 concentration in good agreement with the other test series. This means that any differences are likely not to be caused by variations in CO2 inlet concentration and MEA concentration.
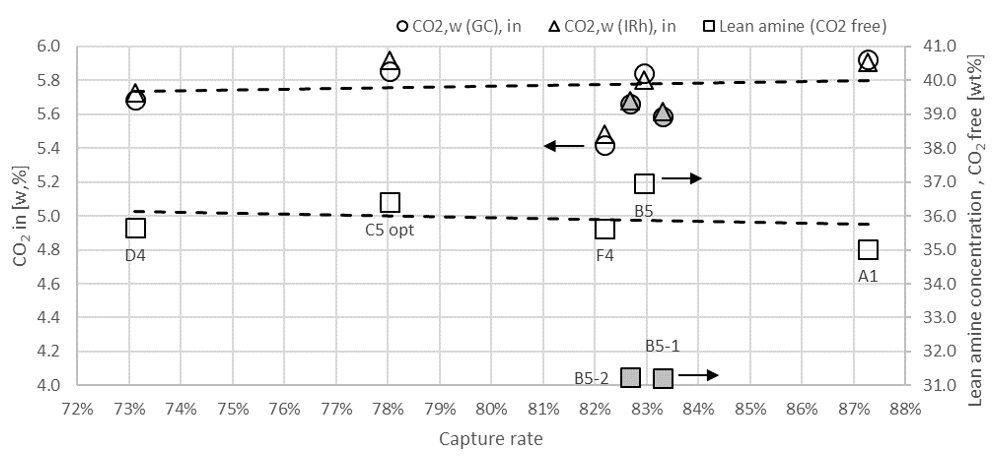
Figure 3. Wet CO2 inlet concentration and lean amine concentration were maintained at the same level for the selected optimum cases. Grey symbols are for the B5-1 and B5-2 cases that were operated at lower amine concentration.
A graph of the variations in L/G and lean loading versus capture rate is given in Figure 4. There was a wide enough experimental window with respect to lean flow and resulting loading to observe trends. A 14 percentage points higher capture rate (from 73 to 87) demands a 0.03 mole/mole lower lean loading and a 0.3 kg/Sm3 higher L/G. It is observed that the MEA 30wt% test cases (B5-1 and B5-2) needs a significantly (0.4 kg/Sm3) higher solvent flow rate to achieve the target capture rate of 82-83% than the results with 35% MEA, while the lean loading is not very different. As mentioned before, all cases are with 67,000 Sm3/h flue gas flow.
A summary of results from the test series is given in Table 3. The CO2 captured in the optimum cases shown in Figure 2 are used for calculating the absorber packing volume.
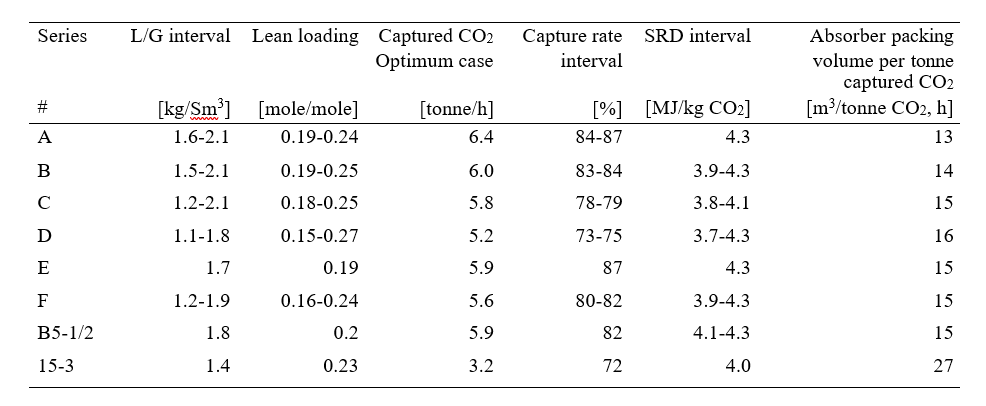
Table 3. Results summary for all test series.
The new property added in this Table is the “Absorber packing volume per ton captured CO2. This is a good property for analyzing the potential for size and weight reductions. Most interestingly, in the A-1 case the CO2 produced is 6,427 kg/h and the absorber packing volume parameter is 13. The result for the 15-3 case from earlier TCM publication
[1] was 27. This shows that there are significant differences in this property and that this campaign had low number ranging from 13-16. The difference with 15-3 up to 27 shows that there should be a significant opportunity for more compact absorber designs and corresponding cost saving for this largest equipment item in post-combustion capture. It is recommended to use this new property in future work for comparison.
A final observation is that no large differences were observed between B5-1 and B5-2. This means that there are likely no big differences between upper and lower water wash (as expected) that can explain any variation or invalidate any conclusions.
4.2 Emissions to air
When changing design of amine based post-combustion one must always keep control of the emissions since these pose of the main HSE risks [8]. Table 4 gives an overview of the measured emissions to air at moments or intervals the results were regarded as reliable and representative. The letter gives which series the measurement is taken from while the number gives which test it is (not reported in detail in this work). Two measurements were done in the C5 test. The online data were 5 min averaged data over an analyzed period of 2 hours.
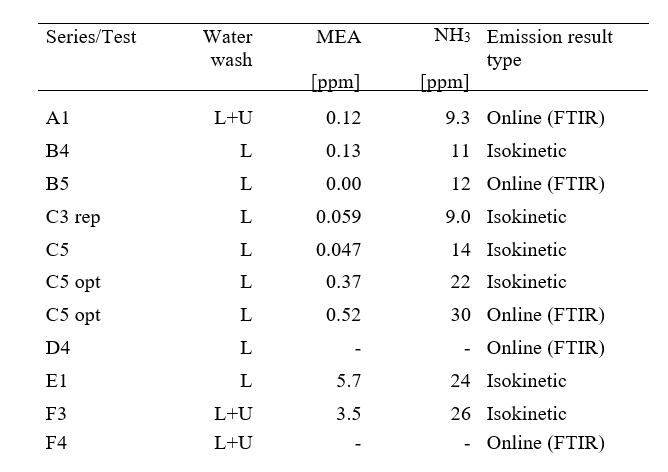
Table 4 Overview of isokinetic sampling during the period. Note that FTIR values are reported for time slots where isokinetic samplings were not available. In addition to optimum cases, emission results are included from B4, C3 rep, E1 and F3. The FTIR was out of service during case D4 and F4.
Except for E1 and F3 the emissions to air were not significantly higher than the ones published earlier on industrially representative operation [9]. In E and F especially the MEA emissions are high, probably higher than most future emission permits will allow. The reason is that the inlet temperature was increased from 30 to 45 °C. So, this seems not to be a favourable measure for compact capture. This indicates that two water washes are likely needed for compact capture if high inlet temperature is unavoidable.
The numbers presented here have a somewhat higher uncertainty than TCM presents in dedicated emission papers, e.g. Morken et al [9]. Some numbers may be somewhat higher than if the plant was operated stable for longer time. Build-up of MEA in water wash can occur. On the other hand, some numbers may be low since they can be impacted by the large amount of dry packing bed above the absorption bed. It must be noted that the quantification limit for the FTIR is 0.5 ppm due to increased instrument noise at low levels. However these uncertainties do not impact the conclusion on the overall low enough levels with 30 °C inlet and the overall too high levels at 45 °C inlet temperature.
4.3 Solvent degradation
When changing design of amine based post-combustion one must also always keep control of the degradation since it can be important for the OPEX and HSE risk. Table 5 gives an overview of metal and heat stable salt (HSS) in the solvent. The compact campaign was done after another campaign of 905 hours. So, the amine was already partly degraded at the start.
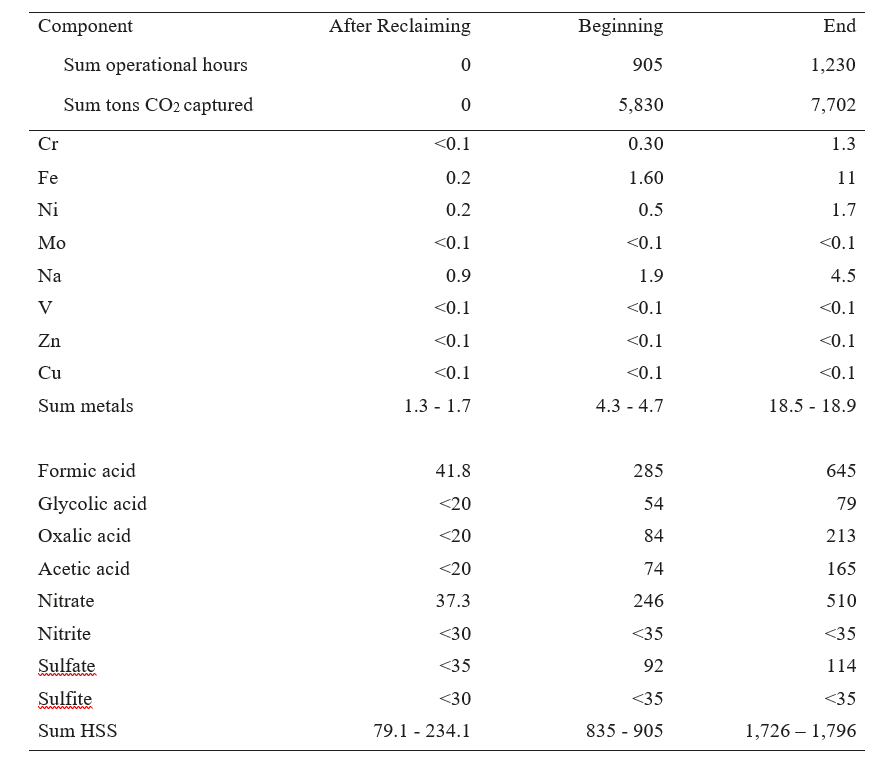
Table 5. Overview of metal and heat stable salt (HSS) concentrations after reclaiming and at the beginning and end of the campaign discussed in this paper. All concentrations are mg/kg solvent. Components below the detection limit are marked with “<”.
The degradation products increase as expected. When deciding to run this campaign with 35wt% a risk of excessive degradation was taken. This risk was mitigated by only running a relative short time of 325 hours. From all the measured concentrations the main discussion in literature focuses on the iron concentration. TCM’s own guideline
[10] recommends keeping the iron concentration below 5 mg/kg solvent. In the compact campaign it increased from from 1.6 to 11 crossing the recommended value. However, Moser [11] has observed long periods of low degradation above the 5 mg/kg iron. It seems anyhow recommended to have more mitigation actions in place for keeping the degradation under control for using 35 w%. Examples are continuous reclaiming or use any of the new O2 and/or iron removal technologies.
4.4 Comparison with previous results
Over the years various campaigns have been executed at TCM with MEA on CCGT flue gas, of which the compact campaign in this paper is the latest. From each campaign one representative result was chosen that has most industrial relevance. Table 6 below gives an overview of these representative results enabling a comparison and perspective of what TCM has been achieved at TCM.

Table 6. Comparison of representative results from all MEA campaigns at TCM.
The results from this compact campaign has as expected the largest SRD as well as the lowest capture rate. The differences with the other cases on variables that are important for cost and HSE are not very large. Hence, we can conclude that the amine unit at TCM can be used to study compact capture, although it is not specifically designed for it. Another result from this Table is a set of data that can be used to tune any overall MEA model. The data give a large enough specter to serve this purpose.
4.5 Discussion on compact capture
The results in this paper give a benchmark for any compact capture technology. Many new ideas and improvements on reducing weight and size can use this work for comparison. Preferably, these should perform better on most variables that are important for cost and HSE. Examples of such more compact but less mature technologies are rotating absorption&desorption, membrane contactors and CO2 selective gas-gas membranes.
But better results could also be obtained at TCM in the future. One improvement idea for TCM is to use another better solvent. This work already shows that increasing the MEA concentration from 30 to 35% makes compact capture design more attractive. TCM has already tested the solvent CESAR-1 for other motivations [12]. Testing this solvent on compact design like done in this work is a logic next step.
Another idea for TCM is to study in more detail metal build-up and degradation rates in compact design. This work provides a good indication but had not enough hours for industrially relevant conclusions. A final idea is further testing at 45°C and two water washes could also be interesting to be able to find the balance with the size of the direct contact cooler (DCC) upstream the absorber and the lean amine cooler.
5. Conclusions
The amine unit at TCM has been used for testing compact capture for providing a benchmark. The absorber was used with its lowest possible packing height of 12m, and only one of two water washes was used (3m height). The plant was operated with a high flue gas flow rate (67,000 Sm3/h) and 35 wt% MEA with a sensitivity down to 30 wt%. The CO2 inlet concentration was 6% mimicking some industrial exhausts, small turbines with exhaust gas recycle or modern turbines with high turbine inlet temperatures. The tests demonstrated that such a low absorber can capture more than 80% of the CO2 with only a slightly higher steam demand than conventional applications with higher packing heights of 18-24m. The low absorber gave 3.9 MJ/kg for the specific reboiler duty for the low absorber, while the higher absorbers gave 3.5-3.7. Acceptable emissions were observed, while degradation was high due to the choice of running with 35 w% MEA. Overall, a benchmark has been provided for future improved compact capture technologies.
Acknowledgements
The authors gratefully acknowledge the staff of TCM DA, Gassnova, Equinor, Shell and Total for their contribution and work at the TCM DA facility. The authors also gratefully acknowledge Gassnova, Equinor, Shell, and Total as the owners of TCM DA for their financial support and contributions.
References
- Gjernes E, Pedersen S, Jain D, Åsen KI, Hvidsten OA, de Koeijer G, Faramarzi L, de Cazenove T, Documenting modes of operation with cost saving potential at the Technology Mongstad, Energy Procedia, 14th Greenhouse Gas Control Technologies Conference Melbourne 21-26 October 2018 (GHGT-14)
- Pettersen J, Nilssen OR, De Koeijer G, Approaching Zero CO2 Emissions for Future Oil and Gas Production Offshore, Abstract and presentation to TCCS-10 conference, June 2019, Trondheim, Norway
- Supap T, Idem R, Veawab A, Aroonwilas A, Tontiwachwuthikul P, Chakma A, and Kybett BD, Kinetics of the Oxidative Degradation of Aqueous Monoethanolamine in a Flue Gas Treating Unit, Ind. Eng. Chem. Res. 2001, 40, 16, 3445–3450
- Thimsen D, Maxson A, Smith V, Cents T, Falk-Pedersen O, Gorset O, Hamborg ES, Results from MEA testing at the CO2 Technology Centre Mongstad. Part I: Post-Combustion CO2 capture testing methodology, Energy Procedia, 63, 2014, 5938-5958,
- Hamborg ES, Smith V, Cents T, Brigman N, Falk-Pedersen O, de Cazanove T, Chhagnlal M, Feste JK, Ullestad Ø, Ulvatn H, Gorset O, Askestad I, Gram LK, Fostås BF, Shah MI, Maxson A, Thimsen D, Results from MEA testing at the CO2 Technology Centre Mongstad. Part II: Verification of baseline results, Energy Procedia, 63, 2014, 5994-6011
- Faramarzi L, Thimsen D, Hume S, Maxon A, Watson G, Pedersen S, Gjernes E, Fostås BF, Lombardo G, Cents T, Morken AK, Shah MI, de Cazenove T, Hamborg ES, Results from MEA Testing at the CO2 Technology Centre Mongstad: Verification of Baseline Results in 2015. Energy Procedia, Volume 114, 2017, p 1128-1145.
- Gjernes E, Pedersen S, Cents T, Watson G, Fostås BF, Shah MI, Lombardo G, Desvignes C, Flø NE, Morken AK, de Cazenove T, Faramarzi L, Hamborg ES, Results from 30 wt% MEA performance testing at the CO2 Technology Centre Mongstad, Energy Procedia, Volume 114, 2017, 1146-1157
- De Koeijer G, Talstad VR, Nepstad S, Tønnessen D, Falk-Pedersen O, Maree Y, Nielsen C. Health risk analysis for emissions to air from CO2 Technology Centre Mongstad, International Journal of Greenhouse Gas Control, 18, 2013, 200–207.
- Morken AK, Pedersen S, Kleppe ER, Wisthaler A, Vernstad K, Ullestad Ø, Flø NE, Faramarzi L, Hamborg ES, Degradation and Emission Results of Amine Plant Operations from MEA Testing at the CO2 Technology Centre Mongstad, Energy Procedia, 114, 2017, 1245- 1262
- Morken AK, Pedersen S, Nesse SO, Flø NE, Johnsen K, Feste JK, de Cazenove T, Faramarzi L, Vernstad K, CO2 capture with monoethanolamine: Solvent management and environmental impacts during long term operation at the Technology Centre Mongstad (TCM), International Journal of Greenhouse Gas Control 82 (2019) 175-183.
- Moser P, Wiechers G, Schmidt S, Monteiro JGMS, Charalambous C, Garcia S, Fernandez ES, Results of the 18-month test with MEA at the post-combustion capture pilot plant at Niederaussem – new impetus to solvent management, emissions and dynamic behaviour, International Journal of Greenhouse Gas Control, Volume 95, 2020.
- Christophe Benquet C, Knarvik A, Gjernes E, Hvidsten OA, Kleppe ER, Akhter S, First Process Results and Operational Experience with CESAR1 Solvent at TCM with High Capture Rates (ALIGN-CCUS Project). To be presented at GHGT-15 Virtual Conference: 15-18 March 2021.
Documenting modes of operation with cost saving potential at the Technology Centre Mongstad (2018)
Erik Gjernesa*, Steinar Pedersenb, Divya Jainc, Knut Ingvar Åsenb, Odd Arne Hvidstenb,d, Gelein de Koeijerb, Leila Faramarzib,d, Thomas de Cazenoved,e
aGassnova SF, Dokkvegen 10, 3920 Porsgrunn, Norway bEquinor ASA, PO Box 8500, 4035 Stavanger, Norway cShell Global Solutions International B.V., PO Box 663, 2501CR The Hague, The Netherlands dTechnology Centre Mongstad, 5954 Mongstad, Norway eA/S Norske Shell, Tankveien 1, P.O.Box 40, 4098 Tananger, Norway *Corresponding author.
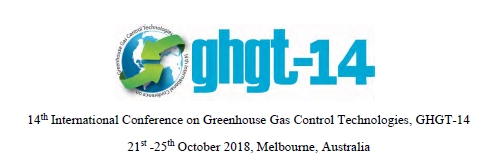
Abstract
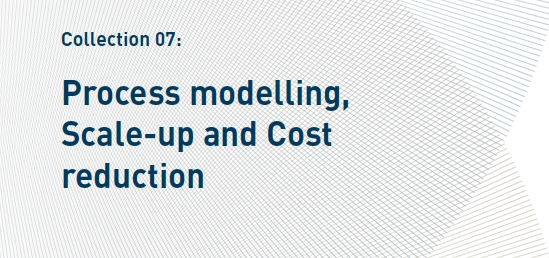
From December 2017 to February 2018 the Technology Centre Mongstad (TCM DA), operated a test campaign capturing CO2 by use of monoethanolamine (MEA) in a 80 to 200 ton CO2 per day demonstration unit. The primary objective was to provide experimental evidence for reducing operational as well as capital costs of CO2 capture. For cost assessment a selection of the test cases has been used as a basis for estimating cost of full scale amine based CO2 capture for a large combined cycle gas turbine based (CCGT) power plant. The cost of CO2 avoided is presented for these cases and the case with the lowest cost of CO2 avoided has been furthered investigated by a parameter study. The cost assessment is presented relative to two earlier MEA campaigns at TCM. A reduction in cost of CO2 avoided up to 18% was justified by experiments while further improvements were made plausible theoretically.
1. Introduction
The Technology Centre Mongstad (TCM) is the world’s leading facility for verifying and improving CO2 capture technologies. TCM is located at Mongstad, one of Norway´s most complex industrial facilities. TCM has been operating since autumn 2012, providing an arena for qualification of CO2 capture technologies on an industrial scale. In autumn 2017, Gassnova (on behalf of the Norwegian state), Equinor (formerly Statoil), Shell and Total entered into a new ownership agreement securing operations at TCM until 2020. The owners of TCM started their most recent monoethanolamine (MEA) test campaign in June 2017 where a large number of public, industrial, research and academic stakeholders were involved [1]. The campaign included demonstration of a model-based control system, dynamic operation of the amine plant, investigating amine aerosol emissions and specific tests targeted at reducing the cost of CO2 avoided. Through the testing, both flue gas sources currently available at TCM were used. These sources are the combined cycle gas turbine (CCGT) based heat and power plant (CHP) and the residual fluidized catalytic cracker (RFCC). They provide flue gases with a wide range of properties and a CO2 content from 3.6 to 14 %. TCM is located next to the Equinor refinery in Mongstad. The Mongstad refinery is the source of both flue gases supplied to TCM.
The part of the test campaign addressing cost of CO2 avoided will be reported in the current paper where the aim is to estimate the potential for cost reduction of some known measures based on experimental data from TCM’s amine unit. This means that these estimates will be experimentally verified. It is the first time such a structured cost reduction test campaign has been executed on such a large test unit. Hence the results are expected to be useful for large scale plants. Besides an experimental verification of known measures, this paper will also use this methodology to estimate other cost reduction measures on a theoretical basis using extrapolation of the verified results.
The performance of TCM’s amine plant was presented in 2014 [2] along with an independent verification protocol developed by Electric Power Research Institute (Epri) [3]. The performance was reported with a specific reboiler duty (SRD) of 4.1 GJ/ton CO2 for a case with 47,000 Sm3/h flue gas flow at 3.7 % CO2 and a capture rate around 85 %. CO2 concentration in the flow in and out of the absorber as well as in the product flow was measured by use of one FTIR unit that cycled between the three flows. One cycle lasted more than one hour, thus simultaneous gas composition measurements could not be presented. In 2015 performance was revisited after a major upgrade of the gas phase measuring system. The upgrade included multiple gas phase analyzers at each of the three flows, i.e. in and out of the absorber and out of the stripper. The use of anti-foam significantly improved the performance and resulted in an SRD of 3.6 GJ/ton CO2 [4] for operation at 59,0000 Sm3/h flue gas flow with 3.6 % CO2 . The 47,000 Sm3/h case was also revisited in 2015 [5] with a test program for energy optimization based on maintaining 85 % capture rate for various combinations of stripper bottom temperature and corresponding lean CO2 loading (mole CO2 per mole amine). This resulted in SRDs for the cases without and with the use of anti-foam of 3.9 and 3.6 GJ/ton CO2 , respectively. These results were used for establishing a baseline. This work takes the next step: how can the cost of capture based on this baseline be reduced through a structured test campaign?
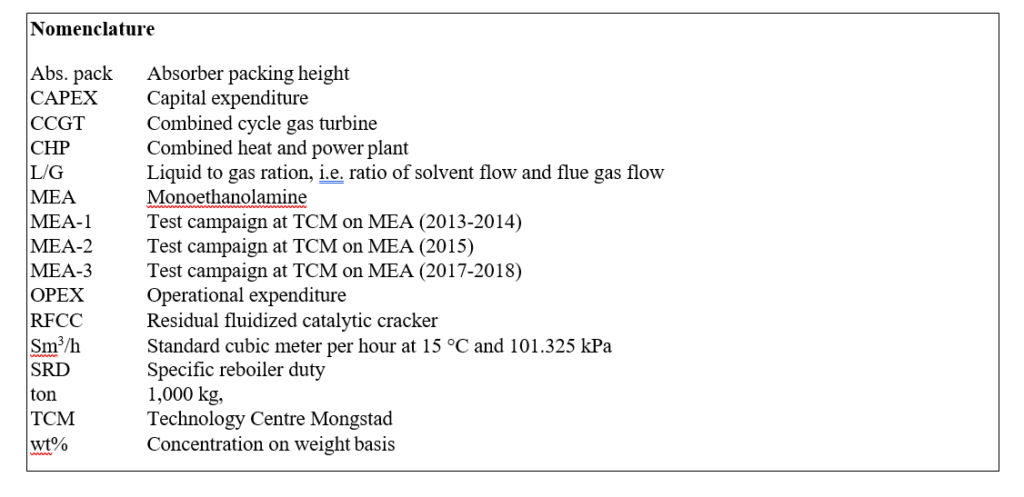
2. Overview of the tests program
The test program that is reported in this paper was executed at TCM from December 2017 to February 2018. The main elements investigated were:
- Absorber configurations with packing heights at 24, 18 and 12 meter
- Solvent concentration with MEA at 30 and 40 wt%
In addition to this, most of the tests were operated at slightly elevated CO2 concentration in the flue gas to be treated, i.e. from 3.6 to 4.2 % CO2 (wet), and during last part of the campaign anti-foam was injected based on experience from the test program in 2015 [5]. The test program contains 18 test series and the main operational parameters are listed in Appendix A.
The operation in December 2017 was stopped due to signs of corrosion i.e. increasing iron content in the solvent and high levels of ammonia emissions to air. Results from corrosion monitoring at TCM is reported in e.g. [6]. After inspection and a comprehensive plant washing operation, the test program was started up again week 3, 2018. The following two months different modes of operation were investigated. Before presenting the experimental results and cost assessments, the definitions of specific reboiler duty, capture rate and CO2 loading will be discussed.
Figure 1 shows the TCM amine plant in CHP mode. It is a flexible plant that enables testing of CO2 capture in several configurations and offers a wide range of flue gas flow rates as well as flue gas compositions [2 to 5]. In the current campaign injection of lean amine is made at three different heights in the absorber and thus utilising 24, 18 and 12 meter absorber packing (yellow boxes in figure), respectively. The CO2 recycle line has been in operation for most of the campaign in order to maintain a CO2 level of 4.2 % (wet) in the flue gas into the absorber.
Specific reboiler duty (SRD) is defined as the heat delivered to the reboiler from the steam system divided by the amount of captured CO2 :
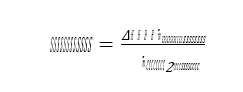
where 𝑚𝑚𝑚𝑚̇ 𝑠𝑠𝑠𝑠𝑠𝑠𝑠𝑠𝑠𝑠𝑠𝑠𝑠𝑠𝑠𝑠𝑚𝑚𝑚𝑚 is the steam flow to the reboiler heat exchanger. ∆𝐻𝐻𝐻𝐻 is the enthalpy difference between steam and condensate calculated from measured temperature and pressure, see also reboiler, steam and condensate in Figure 1. Steam pressure is typical around 2.5 barg and up to 160 °C for the tests reported in this paper.
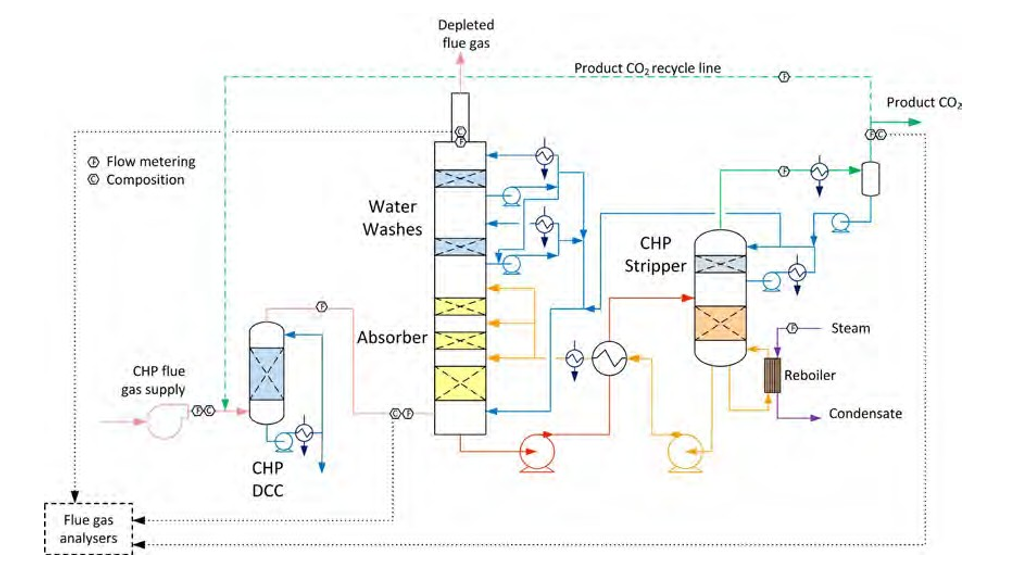
Figure 1. The TCM amine plant in CHP mode (up to 80 ton CO2 per day). Flow meters and flue gas analysers are located at absorber inlet, outlet/depleted flue gas and product flow. Captured CO2 can be recycled, see green dotted line, to increase the CO2 concentration in the flue gas flow into the absorber.
CO2 capture rate is the mass fraction of CO2 being captured out of the amount of CO2 flowing into the absorber:
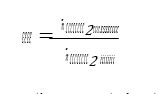
Captured CO2 (𝑚𝑚𝑚𝑚̇ 𝐶𝐶𝐶𝐶𝐶𝐶𝐶𝐶2𝑐𝑐𝑐𝑐𝑠𝑠𝑠𝑠𝑐𝑐𝑐𝑐 ) in (1) and (2) can be based on CO2 in product flow (𝑚𝑚𝑚𝑚̇ 𝐶𝐶𝐶𝐶𝐶𝐶𝐶𝐶2,𝑐𝑐𝑐𝑐𝑝𝑝𝑝𝑝𝑝𝑝𝑝𝑝𝑝𝑝𝑝𝑝) leaving the stripper or on difference in mass flow of CO2 over the absorber (𝑚𝑚𝑚𝑚̇ 𝐶𝐶𝐶𝐶𝐶𝐶𝐶𝐶2𝑠𝑠𝑠𝑠𝑎𝑎𝑎𝑎𝑠𝑠𝑠𝑠,𝑖𝑖𝑖𝑖𝑖𝑖𝑖𝑖 − 𝑚𝑚𝑚𝑚̇ 𝐶𝐶𝐶𝐶𝐶𝐶𝐶𝐶2𝑠𝑠𝑠𝑠𝑎𝑎𝑎𝑎𝑠𝑠𝑠𝑠,𝑝𝑝𝑝𝑝𝑜𝑜𝑜𝑜𝑠𝑠𝑠𝑠). There are several ways of calculating CO2 capture rate [4]. In addition to this and as outlined in more details in [4,5] TCM is equipped with multiple flue gas analysers for measuring composition in and out of the absorber and out of the stripper, see Figure 1. This also includes moisture which alternatively can be calculated based on thermodynamics using temperature and pressure of the gases in question. The flow meter at the absorber outlet is unreliable and flow out of absorber is calculated from flow into the absorber assuming that all components except moisture and CO2 are conserved. The current analysis will be based on the selection of composition analysers, flow meters and calculation methods presented in Appendix B.
Lean and rich solvent loading (mole CO2 /mole amine) are calculated from laboratory analysis of liquid solvent samples that provide total inorganic carbon (mole CO2 /kg solvent) and total alkalinity (mole amine/kg solvent):
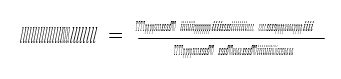
3. Optimising performance: energy
Most of the MEA-3 program was conducted with CO2 concentration at 4.2 % (wet) in the flue gas into the absorber. This was maintained by recycling captured CO2 back to the absorber inlet. This secured stable CO2 concentration in the flue gas since recycled CO2 could top the initial CO2 concentration of 3.5 to 3.9 % up to 4.2 % (wet). This CO2 level is typical for state of the art CCGT plants. Selected test series that will be discussed below are presented in Table 1.
Figure 2 shows to the left the MEA-3 test series 3 with black filled symbols and series 11 with black open symbols. These two series were operated at 47,000 Sm3/h, 24 meter absorber packing and without use of anti-foam. Compared to results from the MEA-2 campaign in 2015 [5] these two new test series resulted in a lower optimum SRD, but this may be due to several aspects and in addition the CO2 concentration in the flue gas into absorber was higher. However, during this part of the campaign the amine plant could be operated at rather high stripper bottom temperature and corresponding low lean solvent CO2 loading without the use of antifoam. Thus, the resulting optimum point was found at a higher stripper bottom temperature and lower lean CO2 loading compared to MEA-2 results, i.e. 118.1 °C /0.29 mole/mole for MEA-2 versus 121.0 °C/0.21 mole/mole for MEA-3. Results down to 3.6 GJ/ton CO2 was not achieved at 24 meter absorber packing when operated without the use of anti-foam and as will be presented below the effect anti-foam was not at all as pronounced as in the MEA-2 campaign. We acknowledge this difference in performance which could be due to several factors, however, this has not yet been concluded.
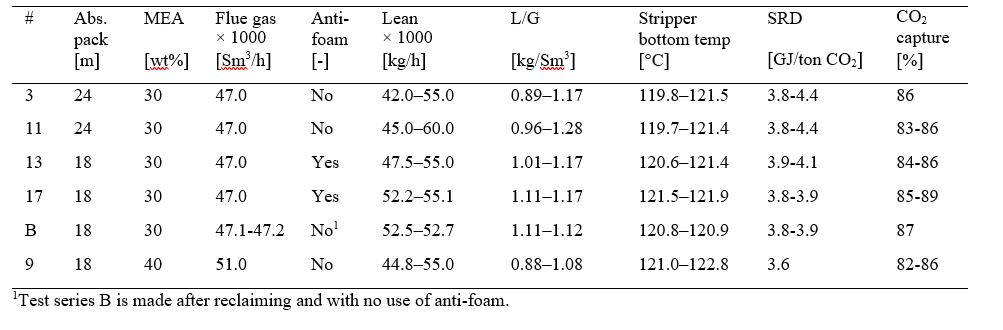
Table 1. Selected test series from MEA-3 campaign at 24 and 18 meter absorber packing, the latter operated at 30 and 40 wt% MEA. The liquid- to gas ratio (L/G) is the ratio of lean amine- to flue gas flow. SRD is based on thermal energy, see equation 1.
All SRDs and capture rates presented in Figure 2, Table 1 and Table 2 are calculated based on that captured CO2 (𝑚𝑚𝑚𝑚̇ 𝐶𝐶𝐶𝐶𝐶𝐶𝐶𝐶2𝑐𝑐𝑐𝑐𝑠𝑠𝑠𝑠𝑐𝑐𝑐𝑐 ) in equation (1) is derived from the difference in mass flow of CO2 over the absorber. Earlier reported data from MEA-2 campaign [5] was based on measured product mass flow of out of stripper. The discussion below is
based on a reassessment of these data using mass flow of CO2 over the absorber. The data points presented are made from averaging process data over a two hour time slot. This time slot also includes liquid solvent samples such that solvent CO2 loading can be calculated according to equation (3).
Performance at 18 meter absorber packing height was investigated at both 30 and 40 wt% MEA. Figure 2 shows to the right the MEA-3 test series 13 and 17 with filled and open brown symbols, respectively. The blue filled symbols are test series B without anti-foam that was executed after solvent reclaiming. The best SRDs were obtained around
3.8 GJ/ton CO2 for test series 17 which is a bit below the 24 meter tests in MEA-2 without anti-foam. The red filled symbols in Figure 2 right hand side shows MEA-3 series 9 which was operated with 51,000 Sm3/h flue gas flow, 40 wt% MEA and without the use of anti-foam. The optimum SRD is similar as the best performance from MEA-2, however, the absorber packing required was reduced from 24 meter (MEA-2) to 18 meter (MEA-3) and no use of anti- foam. Test series 9 was stopped before completion due to increasing ammonia emission and signs of corrosion i.e. increasing iron content in solvent. Thus only a limited number of parameter variations was conducted during operation at 40 wt% MEA and there might still be a potential for obtaining even lower SRDs. Another observation was that the use of anti-foam had limited effect on performance which can be seen from the brown (with anti-foam) and the blue symbols (without anti-foam) in Figure 2 to the right. Case 9-4 that was operated at 40 wt% MEA without the use of anti-foam resulted in the lowest SRD in this campaign.
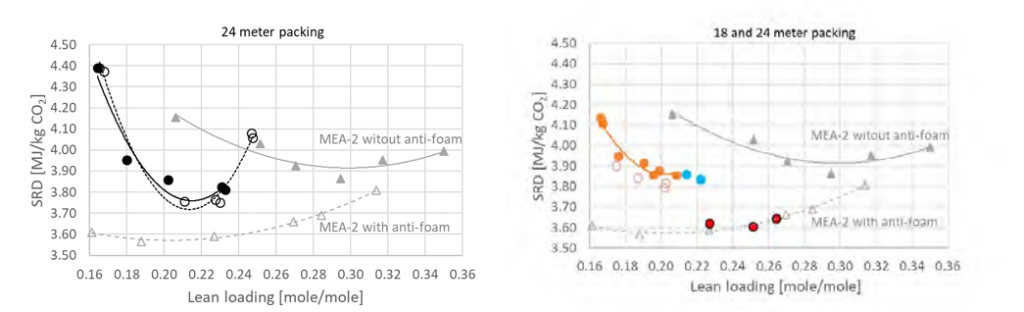
Figure 2. To the left SRD for tests utilising 24 meter absorber packing compared to results from MEA-2 in 2015 (grey symbols and lines). MEA-3 series 3 is with black filled symbols and series 11 is with black open symbols. To the right SRD for tests at 18 meter absorber packing compared to the same results from MEA-2 in 2015 (grey symbols and lines). Series 13 is with brown filled symbols, series 17 with brown open symbols, series B with blue symbols and series 9 which is with 40 wt% MEA, is with red symbols. SRDs are calculated based on difference in mass flow of CO2 over the absorber. All plots except series 9 are with 30 wt% MEA. The right and left figure present the same MEA-2 results utilising 24 meter absorber packing. Table 1 and Table 2 provide more information about the test series.
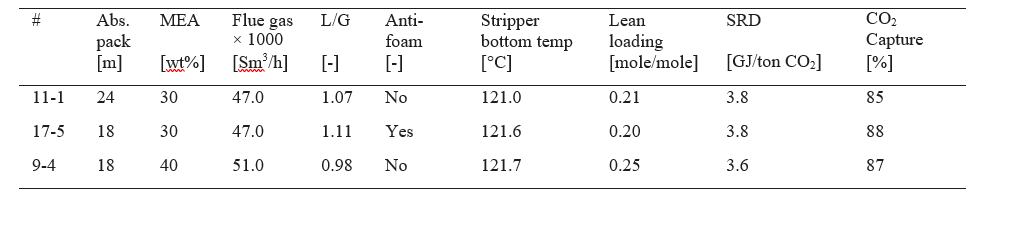
Table 2. With ref to Figure 2 operational data, SRD and capture rate for the three cases at lowest SRD values during MEA-3. SRD is based on thermal energy, see equation 1.
4. Modes of operation
Based on previous work [4,5] it was interesting to further investigate the trade-off between capital expenditure (CAPEX) and operational expenditure (OPEX) parameters for operating conditions relevant for various CCGT- and exhaust gas recycling systems with the aim of providing experimental evidence on how total capture cost can be minimized.
The flexibility of the TCM amine plant was utilized in test series with large variations in absorber packing height, flue gas flow rate, liquid- to gas flow ratio (L/G), solvent CO2 loading and inlet CO2 concentration. This experimental set-up covered a range of operating modes. Data collection and performance results such as mass balance, CO2 recovery, capture rate and SRD are according to methods described in section 2 above. Table 3 gives operational parameters and performance results for selected cases used in the cost evaluation described in section 6 below. Data from previous campaigns, MEA-1 and MEA-2 [2,4], are also included in the table for comparison.
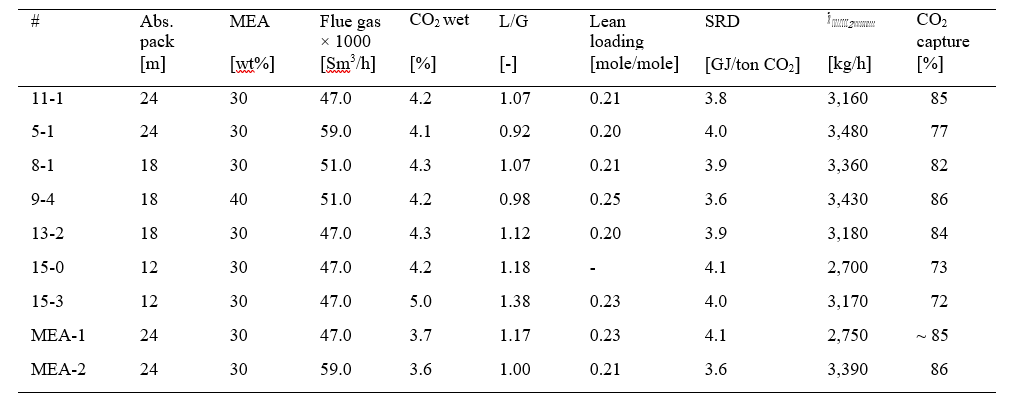
Table 3. Test cases selected for further investigation. Case 11-1 and 9-4 are optimum modes of operation selected from Figure 2. The liquid- to gas ratio (L/G) is the ratio of lean amine- to flue gas flow. SRD is based on thermal energy, see equation 1.
The initial learning at TCM during the years 2013 and 2014 are represented by the test case MEA-1. At that time the operation was mainly with 24 meter absorber packing height and flue gas flow at 47,000 Sm3/h (80 % of design flow capacity). For capture rates between 85 to 90 % the specific reboiler duty was measured to 4.1 GJ/ton CO2.
In the MEA-2 campaign in 2015 learning from several test campaigns were implemented in the test plan. Addition of anti-foam improved especially the stripper performance. This allowed operation with full flue gas load and achievement of both high capture rates and significantly lower SRD values.
In the current MEA-3 campaign, the cases 11-1 and 5-1 are utilizing 24 meter absorber packing height and were run at 47,000 and 59,000 Sm3/h flue gas flow, respectively. The stripper performance constrained the maximum possible CO2 capture to 3,480 kg/h in the case with highest flue gas flow. The corresponding capture rate was 77%. However, during the current campaign no energy optimisation was made at 59,000 Sm3/h flue gas flow and this test was done without the use of anti-foam.
From the three cases run at 18 meter absorber packing height (cases 8-1, 9-4 and 13-2) it is seen that the benefit of 40 w% MEA is lower L/G, lower SRD and still achieving high capture rate. The low L/G and the high lean CO2 loading indicates a further potential for capturing more CO2 in this system.
The two cases run at 12 meter absorber packing height achieved rather low capture rates. The benefit of increasing the CO2 concentration in the flue gas flow into absorber from 4.2 to 5.0 % (wet) is assessed based on results from these two cases.
5. Cost assessment and cost of CO2 avoided
The economic evaluations of power and capture plants in this paper is based on standard “Cost of Electricity” (COE)- and “Cost of CO2 avoided” metrics. These calculations are based on aligned and standardized estimates and assumptions on technology process performance such as energy efficiency, CO2 generation and capture rates, see e.g. [7]. Cost estimates include CAPEX, operations and maintenance (O&M) including fuel and a set of general price and rate of return assumptions. For each case in section 6 below, a complete sized capture plant equipment list is established. Aspen In-Plant Cost Estimator (IPCE) V9 is used to estimate equipment cost. Equipment installation factors are then used to estimate total installed costs. The OPEX can be split in annual cost (of capex), power loss, maintenance, chemicals and fixed operating costs. The gas fired power plant specific cost is based on GTPro and a West Europe scenario. All calculations are furthermore carried out at:
- normalised, per unit (kWh) output from the base industrial (power) plant
- pretax, pre-financing basis
- annual cost basis, applying a capital charge factor corresponding to a standard discount factor and project time horizon
Cost of CO2 avoided ($/ton CO2 ) is calculated according to (4) below and is based on cost of electricity (COE) and CO2 emission per kWh ( CO2 emission) for a power plant with capture (cap) and without CO2 capture (no cap).

The calculated cost of CO2 avoided implicitly accounts for the capture systems’ own energy demand and its inherent CO2 emissions. The following economic assumptions are applied:
- Fuel gas price: 0.1875 US $/Sm3
- On-stream hours: 7,884 (90 %)
- Discount rate: 5 % real (pretax)
- Time horizon: 30 years
This paper will only report percentage cost reduction and no absolute cost numbers. The main reasons are that the absolute numbers are not useful for the purpose of this work and are partially confidential. In this work one consistent method and one consistent set of assumptions are used for calculating the cost, which is important for a fair comparison.
6. Cost evaluation of selected cases
The experiments targeted lowest possible absorber packing height, lowest possible L/G and SRD while maximizing the captured CO2 and capture rate. In Table 4 below the experimental data for the selected cases are scaled to a full- scale design at a fixed inlet CO2 flow of 150 ton CO2 /h and measured capture rate case by case.
In order to compare the MEA-1 and MEA-2 to MEA-3 on the same basis in the cost assessment, the CO2 inlet concentrations for these two cases are adjusted up to 4.2 % (wet) and the flue gas flow rates are reduced correspondingly, reducing the size and cost of flue gas blower, DCC and absorber. The superficial gas velocity is held constant in the DCC and absorber, reducing the diameter of these units.
The adjusted/scaled absorber packing height and the most important cost parameter, the packing volume, are calculated from the experimental data for the cases selected in the MEA-3 campaign. The scaled-up absorber volume is based on packing height utilised for each TCM test case and a scaled up cross sectional area. The latter is calculated based on TCM cross sectional area and the ratio of full-scale (150 ton CO2 /h) to TCM (case by case) CO2 inlet flow. For all scaled up cases the cross sectional areas are adjusted to fit with a superficial velocity of 2 m/s (at 0 °C, 1 atm).
Thus, packing height, see Table 4, is adjusted in order to maintain the scaled-up absorber packing volume. The packing volume per captured CO2 will be equal for each TCM and corresponding scaled up case. The data are shown in Table 4 below together with calculated lean solvent flow per kg CO2 into absorber, CO2 loading in lean and rich amine. The rich CO2 loading is calculated based on solvent flow rate and captured CO2 .
Packing volume is a major CAPEX element and for operation with 30 and 40 wt% MEA the most cost-effective packing volume demonstrated at TCM was about 37 m3/ton CO2 capture per hour for the current test conditions. This result is however, design and site specific. In case 9-4 with 40 wt% MEA the main cost reduction parameters are reduced enthalpy to reboiler (low SRD) and reduced solvent flow rate.
The case 11-1 had more packing than needed and very little CO2 is captured in the upper 6 m packed bed. The cases 11-1, 8-1 and 13-2 performed close to the MEA-2 results, while the case 5-1 was performing poorer. The flue gas flow rate was very high in this case resulting in high CO2 flow into the absorber. The rich CO2 loading was high, indicating that the solvent flow rate was too low to achieve high capture rate. Solvent flow rate was 12.02 kg solvent per kg CO2 in comparison to at least 13.50 kg solvent per kg CO2 into absorber for the best cases. In new campaigns some of the cases could be further improved if higher capture rates are obtained.
The cases 15-0 and 15-3 with 12 m absorber packing achieved the lowest packing volume per kg CO2 captured. On the other hand, the capture rate was low and the solvent flow rate was higher. This resulted in higher capture cost. These cases had in fact a too low packing volume.
In MEA-1 the packing volume was slightly higher than for the 11-1 case, solvent flow was higher and the rich loading was lower. In MEA-2 with 24 meter absorber packing height, the packing volume of 50 m3 per ton CO2 captured is on the high side compared to the MEA-3 results.
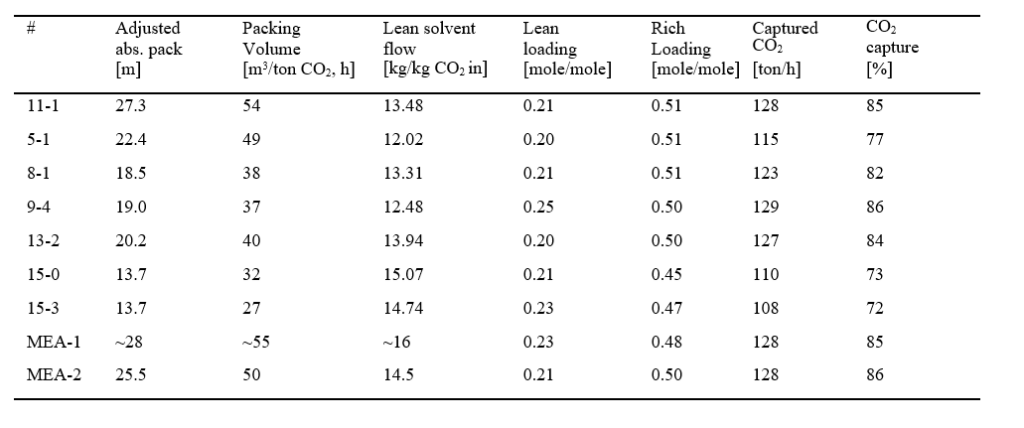
Table 4. The test cases selected for further investigation are scaled up to 150 ton of CO2/h in the flue gas into the absorber base on 2 m/s superficial velocity (at 0 °C, 1 atm) in the absorber. Case 11-1 and 9-4 are optimum cases in Figure 2 while rest of the tests documents different modes of operation.
Section 5 above introduces the economic evaluation and cost of CO2 avoided. In Figure 3 to the left the demonstrated cost reduction for the seven test cases selected from MEA-3 is presented relative to the cost of CO2 avoided of MEA-1. The demonstrated effect of increasing the CO2 concentration in flue gas into absorber from 4.2 to
5.0 % (wet) is shown by cases 15-0 and 15-3. When scaled to 150 ton CO2 /h the cost reduction for 15-0 to 15-3 is mainly due to the reduced resulting flow of flue gas, impacting the cost of the DCC, flue gas blower and absorber. Case 9-4 demonstrates the largest cost reduction contribution, i.e. 13.5 % down relative to MEA-1. This case is also presented in Figure 3 to the right (MEA-3) along with MEA-2 and a theoretically case based on 9-4 assuming 5 % CO2 (wet) in the flue gas. The latter improves the case 9-4 by about 5 % points.
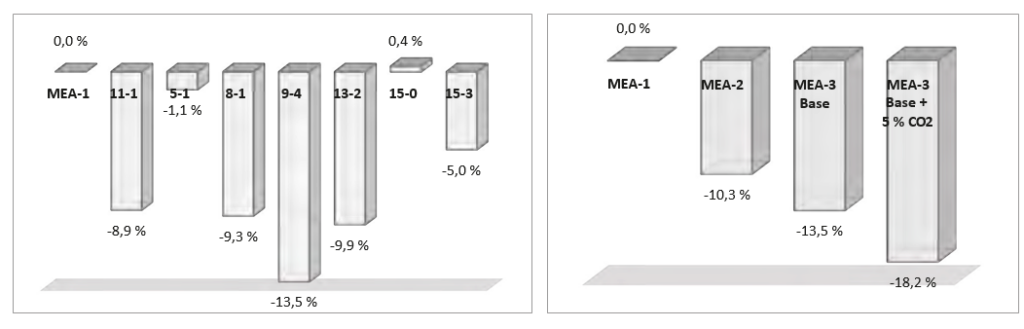
Figure 3. To the left: Demonstrated reduction in cost of CO2 avoided for seven selected MEA-3 cases. To the right: Lowering cost of CO2 avoided in campaigns MEA-1 to MEA-3. The MEA-3 is also presented with its theoretically potential if CO2 content in flue gas is 5 % (MEA-3 Base + 5
% CO2 ). Results are presented relative to assessment made for MEA-1 in 2014. Note that case 9-4 in the left plot is presented as “MEA-3 base” in the right plot.
The measures in Figure 3 do not represent radical new ways of operating or new technologies. They can rather be categorized as learning-by doing. They are typically measures relevant for the first few plants, also called FOAK – first of a kind. Since the cost reduction potential of these measures is experimentally verified in one of the world’s largest demonstration plants, the cost reduction should be highly accurate, and hence relevant for future post- combustion plants.
Based on the experience from the test campaign other reduction measures have been studies on a theoretical basis in order to investigate future potential for reducing cost of CO2 avoided. A theoretical parameter study has been made based on case 9-4, referred as “MEA-3 Base” in Figure 4. The following elements have been assessed:
- Reduce from 2 × 3 meter wash section to 1 × 3 meter wash section
- Reduce solvent consumption from 1.6 kg/ton CO2 down to 0.3 kg/ton CO2 [8,9]
- Increase CO2 capture rate from 86 to 90 %
- Reduce steam consumption to achieve SRD of 3.1 GJ/ton CO2 (other solvents than MEA)
- Increasing CO2 content in flue gas from 4.2 up to 5 %
These measures are considered to be realistic. Most of the numbers are reported in the post-combustion literature and seem reasonable. In addition to these measures reduced manning is also included in the parameter study for illustration:
- Reduced manning from 4 operators per shift to 1 operator per shift
Figure 4 shows the cumulative effect for cost of CO2 avoided from these 6 elements. Solvent and process development relates to the first five items. The assumptions on operators before and after reduction is not based on TCM experience. The second last element corresponds to state of the art CCGT plants that are expected to be operated at 5 % CO2 . The five first elements improves the “MEA-3 Base” by 17.1 % while utilizing all six elements results in 21.5 % improvement.
All in all, these initiatives will represent a reduction in cost of CO2 avoided of the order of 30 % when compared to MEA-1. However, note that these measures are not necessary cumulative, i.e. all combinations may not be possible at the same time.
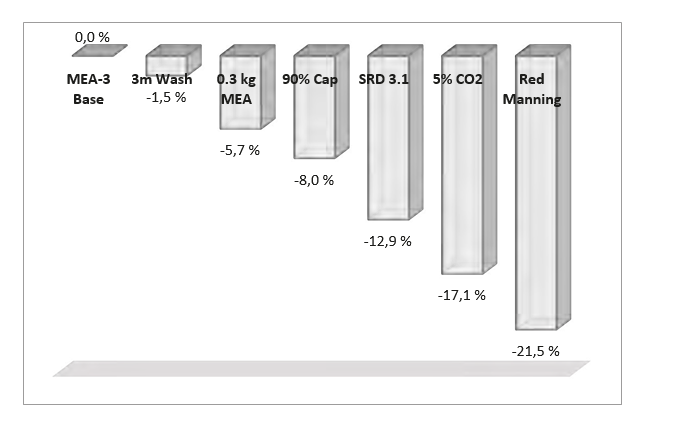
Figure 4. Relative cost of CO2 avoided based test case 9-4 (MEA-3 Base) and a theoretical parameter study involving 6 cost reduction initiatives introduced on top of each other.
7. Conclusion
Different modes of operation with cost saving potential were executed as part of the MEA-3 campaign at TCM from December 2017 to February 2018. The target was to explore learning from five years of operation at TCM with respect to overall cost reduction potential using the relative cost of CO2 avoided metric. The new results were therefore compared to those reported from the MEA-1 and MEA-2 campaigns. The investigation of optimum energy performance identified that SRD values below 3.6 GJ/ton CO2 for MEA are challenging to achieve with 30 wt% MEA and a CCGT like flue gas. This performance is achieved at TCM with a conventional amine plant and may be optimized with an advance process plant. In the cost reduction part of the investigation the level of 10 % cost reduction in cost of CO2 avoided as achieved in MEA-2 was confirmed with the new experiments. Packing volume is a major CAPEX element and the most cost-effective packing volume demonstrated based on TCM equipment, was about 37 m3/ton CO2 capture per hour for the current test conditions. The lowest cost of CO2 avoided was demonstrated when using MEA at 40 wt% and 18 meter absorber packing height. Compared with MEA-1 results a cost reduction of 13.5% was demonstrated. There is likely a further cost reduction potential of 5 %-points for this case. This is based on results from tests when the flue gas CO2 concentration was increased from 4.2 to 5.0 % (wet). Finally, a theoretical parameter variation showed a potential cost reduction of around 20 % compared with MEA-3 Base. Compared to MEA-1 this amounts to a reduction potential of the order of 30 %. However, all combinations may not be possible at the same time.
It is important to notice that these results are generated at one of the world’s largest capture demonstration units, and that it is the first time that such a structured campaign is executed. Similar testing can be carried out with different amine-based solvents. Therefore, these results at TCM scale represent a very relevant basis for scale up and industrial design of amine solvent capture technologies.
Acknowledgements
The authors gratefully acknowledge the staff of TCM DA, Gassnova, Equinor, Shell and Total for their contribution and work at the TCM DA facility. The authors also gratefully acknowledge Gassnova, Equinor, Shell, and Total as the owners of TCM DA for their financial support and contributions.
Appendix A
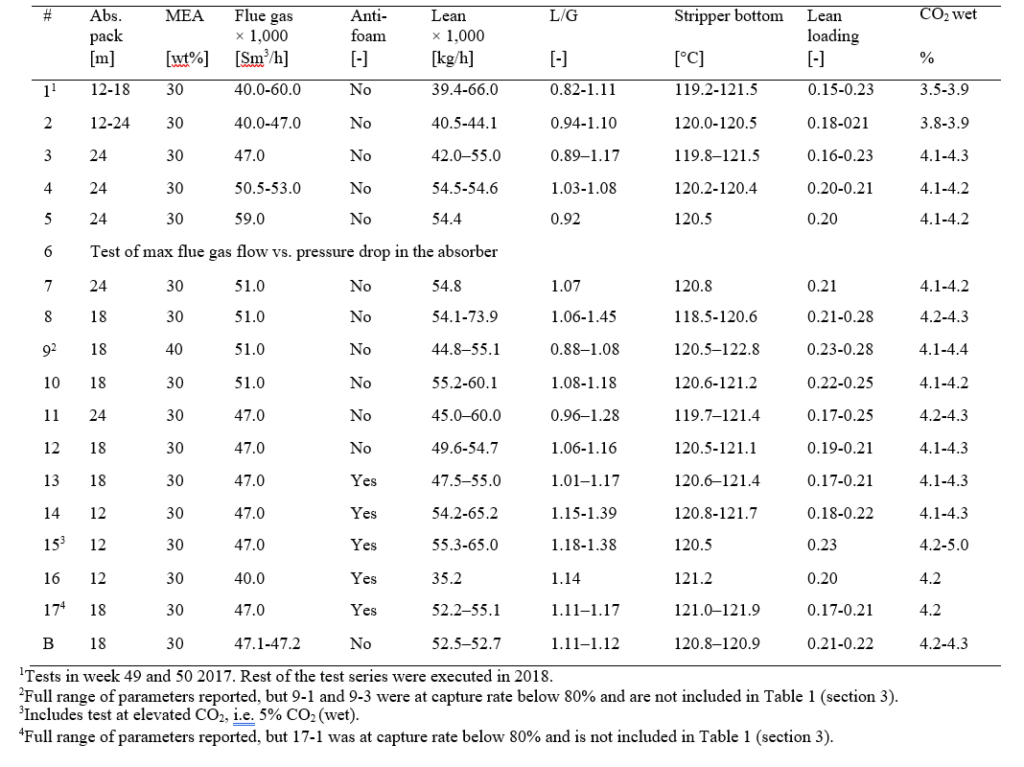
Table A1. The test series during MEA-3, 2017-2018.
Appendix B
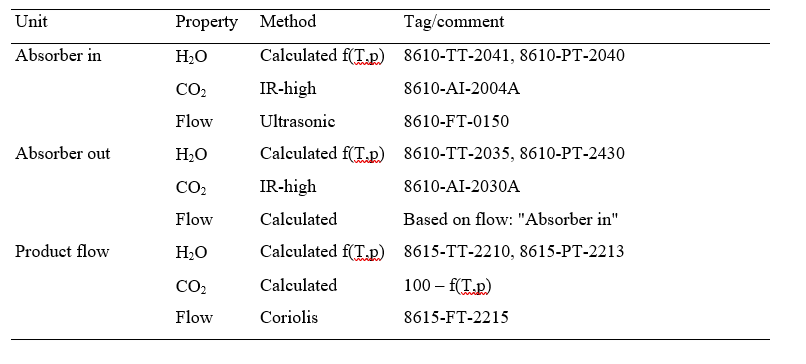
Table B1. Selected instruments and calculation methods for analysing test data.
With ref to Table B1 the volume flow out of the absorber (𝑉out) is calculated from volume flow into (Vin) the
absorber assuming all components except water (𝐶H2O) and CO2 (𝐶CO2) are conserved:
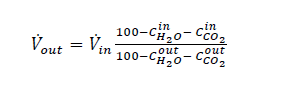
References
- The Open-source Centre at TCM, https://catchingourfuture.com/
- Hamborg ES, Smith V, Cents T, Brigman N, Falk-Pedersen O, de Cazenove T, Chhaganl M, Feste JK, Ullestad Ø, Ulvatn H, Gorset O, Askestad I, Gram LK, Fostås BF, Shah MI, Maxson A, Thimsen D. Results from MEA testing at the CO2 Technology Centre Mongstad. Part II: Verification of baseline results. Energy Procedia, Volume 63, 2014, p. 5994-6011.
- Thimsen D, Maxson A, Smith V, Cents T, Falk-Pedersen O, Gorset O, Hamborg ES. Results from MEA testing at the CO2 Technology Centre Mongstad. Part I: Post-Combustion CO2 capture testing methodology. Energy Procedia, Volume 63, 2014, p. 5938-5958.
- Faramarzi L, Thimsen D, Hume S, Maxon A, Watson G, Pedersen S, Gjernes E, Fostås BF, Lombardo G, Cents T, Morken AK, Shah MI, de Cazenove T, Hamborg ES, Results from MEA Testing at the CO2 Technology Centre Mongstad: Verification of Baseline Results in 2015. Energy Procedia, Volume 114, 2017, p 1128-1145.
- Gjernes E, Pedersen S, Cents T, Watson G, Fostås BF, Shah MI, Lombardo G, Desvignes C, Flø NE, Morken AK, de Cazenove T, Faramarzi L, Hamborg ES. Results from 30 wt% MEA performance testing at the CO2 Technology Centre Mongstad. Energy Procedia, Volume 114, 2017, p 1146-1157.
- Flø NE, Faramarzi L, Iversen F, Kleppe ER, Graver B, Byntesen HN, Johnsen K. Assessment of material selection for the CO2 absorption process with aqueous MEA solution based on results from corrosion monitoring at Technology Centre Mongstad. To be presented at GHGT- 14, 2018, Melbourne, Australia.
- Cost and Performance Baseline for Fossil Energy Plants Volume 1a: Bituminous Coal (PC) and Natural Gas to Electricity Revision 3, July 6, 2015, DOE/NETL-2015/1723.
- Morken, AK, Pedersen S, Kleppe ER, Wisthaler A,Vernstad K, Ullestad Ø, Flø NE, Faramarzi L, Hamborg ES. Degradation and Emission Results of Amine Plant Operations from MEA Testing at the CO2 Technology Centre Mongstad. Energy Procedia, Volume 114, 2017, p 1245- 1262.
- Gorset O, Knudsen JN, Bade OM, Askestad I. Results from Testing of Aker Solutions Advanced Amine Solvents at CO2 Technology Centre Mongstad. Energy Procedia, Volume 63, 2014, p 6267-6280.
Monitoring real time, in- line variations of noble gas concentrations during CO2 capture operations by means of a portable mass spectrometer (2018)
Anja Sundal1*, Ulrich W. Weber1, 2, Matthias S. Brennwald2, Philip Ringrose3, 4, Nina Enaasen Flø4, 5, Kim Johnsen4, 5, Leila Faramarzi4, 5, Per Aagaard1, Rolf Kipfer2
1Department of Geosciences, University of Oslo, Sem Saelands vei 1, 0371Oslo, Norway 2Swiss Federal Institute of Aquatic Science and Technology, Dübendorf, Switzerland 3Norwegian University of Science and Technology, Trondheim, Norway 5Technology Centre Mongstad, Norway *Corresponding author
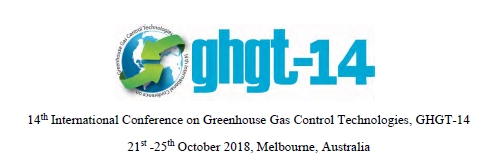
Abstract
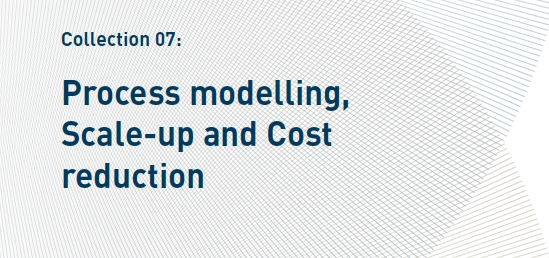
In the ICO2P-project the overall aim is to develop an innovative and cost-effective monitoring scheme for CO2 capture and storage operations, implementing new methods for in situ noble gas measurements. The first step is to establish a basis for fluid source identification by recording temporal variance of noble gas composition in CO2 product. Studies of noble gases related to CCS typically include few, single point samples. In ICO2P, a portable mass spectrometer (i.e. miniRUEDI) is utilized to directly measure real time variability of the noble gas content in CO2 gas streams at operating CCS facilities. The first study was performed at the Technology Centre Mongstad (TCM) in Norway, a large-scale test facility for post-combustion CO2 capture operations. During an open scientific test campaign for amine-based capture (TSA-MEA), noble gases (He, Ar, Kr) as well as CO2 , N2, O2 concentrations in the CO2 product stream were recorded every 10 to 15 minutes during a 5-day period. He concentrations (<0.001ppm)were depleted post-capture and too low for temporal variation measurements in the CO2 product line. Ar concentrations (0.15 – 0.65 ppm) were significantly higher and temporal variation was successfully recorded in the CO2 product line. Ar was found to be sensitive to capture operations, e.g. CO2 recycling ratio. This new approach will provide knowledge of the uniqueness and variability of inherent noble gas fingerprints and depletion/absorption during CCS operations and provide grounds for comparison between fluid origins needed in leakage detection schemes at CO2 storage sites.
1. Introduction
During a recent feasibility study [1], to assess the potential for real-time, semi-continuous noble gas monitoring, data were collected from CO2 capture operations at the Technology Centre Mongstad (TCM) in Norway (Fig. 1), using a novel method for quantitative gas composition measurement. The overall aim has been to contribute towards developing innovative and cost-effective monitoring schemes for CO2 capture and storage operations, work that is now part of an ongoing research project – the ICO2P-2 project [1] during 2018-2021.
By recording temporal variance of noble gas composition in CO2 capture products, the inherent geochemical fingerprint can be evaluated with respect to the potential for source identification at prospective storage sites (i.e. differentiation of sources at CO2 seepage sites or natural variations in marine and terrestrial environments). Studies so far typically include only a few, single point samples, and there is a clear need for more background data on gas compositions and better sampling strategies to ensure safe storage and reliable leakage detection. The miniRuedi [2] portable mass spectrometer has the capacity to measure low concentrations (i.e. partial pressures) of He, Ar and Kr, as well as CO2 , CH4, O2 and other relevant chemical substances with high accuracy at sample intervals of < 15 minutes. This technology proved suitable for monitoring fluctuations in some components of the CO2 -product composition, as tested during an open scientific test campaign for post-combustion capture processes; amine-based temperature swing absorption (TSA), at TCM in summer 2017.
This data set, and further work, will provide important knowledge on absolute variability in CO2 product from complex and mixed sources. During capture operations and before storage of CO2 can commence, there is a need to document variability, and to evaluate the inherent compositional signature (and the potential need for adding tracers). This approach will allow for source-specific identification of fluids; differentiating injected (anthropogenic) CO2 from natural (methanogenic / biogenic) CO2 rich gases at potential leakage points.
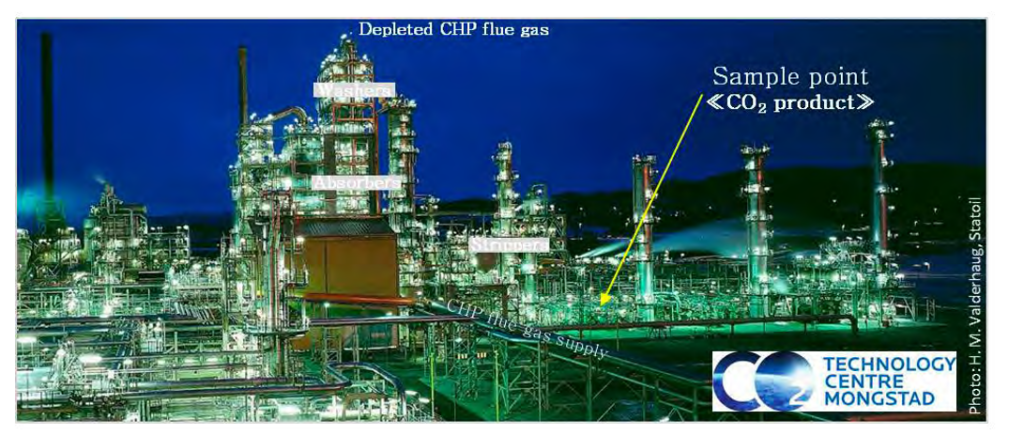
Fig. 1. Technology Centre Mongstad (TCM), Norway [Photo © Equinor] DA amine plant with CHP flue gas inlet from the front, and the high
absorber tower in the middle. “Captured” CO2 product outlet and sample point towards the right.
2. Noble gases as tracers in CCS (and EOR)
Reliable monitoring is a prerequisite for safe, long-term storage and public acceptance of CCS. Terrestrial noble gases (i.e. He, Ne, Ar, Kr, Xe) appear to be suitable natural tracers for monitoring and understanding CO2-rich gas systems [3]. They are chemically inert and non-degradable. And in contrast to several commonly applied chemical tracers (e.g. PerFluoroCarbons), inherent noble gases are not harmful to the environment and bring no additional cost. The concept of using noble gases for monitoring CO2 projects has been demonstrated at small-scale onshore facilities, and noble gas data proved to be crucial evidence to rule out an alleged leakage incident at the Weyburn project [4].
However, there are still knowledge gaps: studies so far typically include only a few, single-point samples, and there is a clear need for better data on gas compositions and variability.
To understand large-scale reservoir dynamics and fluid mixing at CO2 injection sites and along possible leakage paths, experience from research related to multi-phase hydrocarbon systems have to be used and adapted to the scientific case of CCS. Formation water in deep, saline aquifers will have a unique noble gas signature based on a blend of an atmospheric component (stable contents of 20Ne, 36Ar, 84Kr), a deep mantle component (mostly supplying 3He) and a crustal radiogenic component (production of 4He, 21Ne, 40Ar). The signature is a function of time and dependent on the in-situ lithology [5]. Crustal 4He is supplied from radiogenic decay of U and Th in minerals, and 40Ar is produced by radioactive decay of 40K. The mixing ratios of meteoric, mantle and crustal components may yield information about residence times and fluid mixing. E.g., it was found that seepage of methane causes depletion of 20Ne and 36Ar relative to 4He by partitioning, as the heavier noble gas species are more soluble in methane than in water [5]. Exchange between groundwater and oil phase may also be detected as increased 20Ne and 36Ar abundance in oil, as described for the Magnus Field in the British North Sea [6]. Injected CO2 -rich fluid will also interact with formation water and/or hydrocarbon phases present in a storage reservoir. A recent tracer test at the Cranfield enhanced oil recovery (EOR) field in the US, adding Kr and Xe, showed noble gases to be stripped from formation water and into the CO2 phase [7]. Mapping of the background noble gas abundance before injection is of utmost importance to characterize the natural gas composition and spatial distributions prior to CO2 injection. This will improve the understanding of CO2 trapping mechanisms and oil/water/gas partitioning in reservoirs, and form the grounds for comparison needed in leakage detection schemes.
2.1 Compositional variability in captured CO2
Norway is currently at the forefront in developing CCS, along with Canada, Australia, USA and China. With two active storage sites and a national plan to establish another, larger-scale offshore storage site at Smeaheia, there is an imminent need to prepare reliable, cost-effective and long-term monitoring programs. Noble gas finger-printing can provide an applicable solution, but there is a significant knowledge gap concerning the inherent signature of CO2 from various capture processes.
At the operative Sleipner and Snøhvit sites, inherent CO2 from fossil sources (natural gas reservoirs) is captured (as part of the gas processing) and re-injected into a storage formation (saline aquifer). Such single-source storage schemes are expected to display the least degree of temporal variation in the noble gas compositions of injected gas. The noble gas signature of a given natural gas accumulation is related to long-term, slow accumulation rates of e.g. 3He, 4He, 21Ne, 40Ar in a given geological setting. Lateral or vertical compositional gradients in reservoirs related to the lithology and contact time with fluids in traps and along migration paths, may be documented as compositional changes during production. However, these changes are expectedly subtle, compared to complex mixed-source and post-combustion schemes. At the Technology Centre Mongstad (TCM) the source is mixed, and natural gas from several reservoirs are combusted before CO2 -rich flue-gas enters the capture facility. CO2 is captured from a combination of flue gases; (1) from a combined cycle gas turbine (CCGT) based heat and power plant (CHP) or (2) the residual fluid catalytic cracker (RFCC) at the Equinor refinery at Mongstad. At the CHP plant large quantities of gases from different fossil sources are combined, but after initial mixing and combustion, the signature of the flue gases are expected to become relatively homogenous within a few days. Then, with addition of residual refinery gas, the compositional variability is expected to increase. Further, the combustion process will add an atmospheric component.
Depletion of the radiogenic/nucleogenic components of reservoir derived noble gases occurs during combustion and capture [8]. In the product line recirculation rates, addition of different solutes with given solubilities for the gases and their isotopes under varying pressure and temperature conditions will affect noble gas contents. In this study, we attempt to document temporal changes, and the next step is to interpret and decipher the relative effects of capture processes on noble gas fingerprinting. Additionally, documenting variation in the fossil gas feed is highly relevant in evaluating the traceability of mixed-source gas in future storage schemes, involving captured CO2 also from other industries (e.g. cement, ammonia, waste incineration, biogas). E.g. the presented storage scheme for Smeaheia involves storage of CO2 from mixed sources, after post-combustion capture.
3. Methodology
3.1 Capture processes at TCM
The Technology Centre Mongstad (TCM) is a large-scale test facility for CO2 capture operations. This study was conducted in July 2017 during an open scientific test campaign for post-combustion capture processes, using amine-based temperature swing absorption (TSA) [e.g. 9, 10, 11]. Monoethanolamine (MEA) is used as solvent for CO2 . The combined cycle gas turbine and power plant is run with reservoir gas from different fields in the North Sea mixed with a fraction (up to 50 %) of residual gases from the nearby refinery. Flue gas is fed into the TCM plant, cooled, run through the absorber, before CO2 is stripped off by heating the solvent and vented to a safe location in a dedicated vent stack (Fig. 2). During the test period, the capture plant was run with flue gas from the CHP plant, with a CO2 concentration of approximately 4 vol%. In addition, recycling (i.e. return of CO2 product back to feed gas upstream absorber) was performed, increasing the CO2 content to mimic concentrations similar to coal combustion capture (Fig. 2).
3.2 Portable mass spectrometer
The adapted mass spectrometric technology for analyzing extremely low concentrations and the isotopic fractionations of He, Ne, Kr, Xe, Ar in water and gas is available only in few laboratories worldwide, with the research laboratory at Eawag/ETH (Zürich) being one of the pioneers in this field.
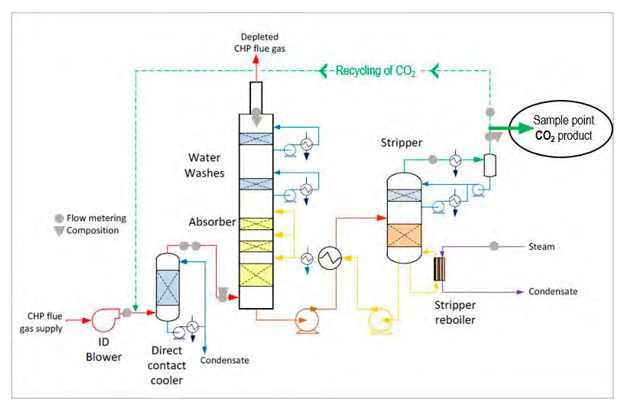
Fig. 2. Post-Combstion CO2 capture at the Technology Centre Mongstad during TSA-MEA test campaigns. A mobile mass spectrometer was connected to the CO2 product line. Modified from Thimsen et a. [9].
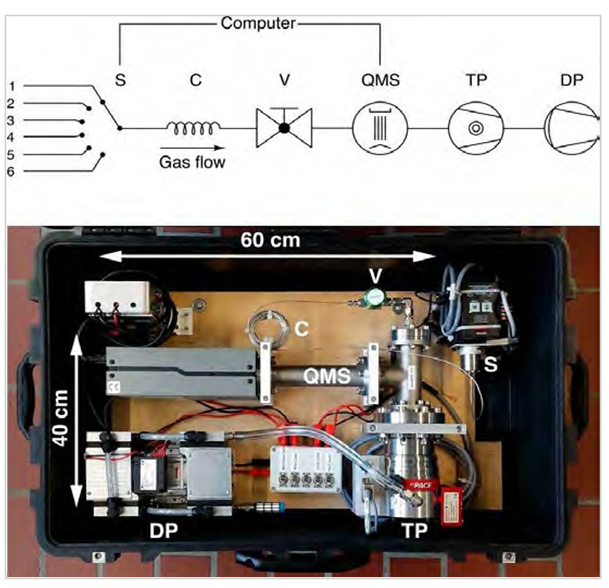
Fig. 3. The miniRuedi portable mass spectrometer (figure from Brennwald et al. [2]) set up with 6-port inlet selector valve (S), capillary (C), inlet valve (V), quadrupole masspectrometer (QMS), turbomolecular pump (TP), and diaphragm pump (DP).
3.3 Sample set-up
A miniRuedi [2] mass spectrometer was connected to the outgoing CO2 product line, downstream the overhead condenser of the CO2 stripper (Fig. 2). This stream is water-saturated, and the slip-stream to the instrument was passed through a dryer to avoid the risk of condensation in the instrument. A pressure regulator was mounted between the sample point and the membrane inlet to decrease the inlet pressure to atmospheric pressure. This inlet pressures were fairly constant and recorded with a pressure sensor. The instrument was mounted in less than 1 hour, and ran continuously during a 5-day test period. The analytical sequence was set to repeating cycles of one air-standard analysis block (calibration), followed by three CO2 output stream sample analysis blocks. An ambient air sample analysis block was added intermittently to remove residual CO2 from the ion source. The analysis blocks lasted between 10 and 15 minutes depending on the number of components measured. During the test He, H2O, Ne, N2, O2, Ar, CO2 and Kr were measured. In this way, a unique, semi-continuous data series of gas content was collected and suitable measurement routines were established. Single samples were gathered for lab analysis of noble gas isotopes.
4. Results
The instrument ran steadily throughout the sampling period. However, as this was a feasibility study, parameters such as air-calibration and sampling intervals as well as the selection of analyzed components were tweaked and tested underway. Absolute concentrations of CO2 , O2 and N2 were compared with measurements performed by TCM (in- line gas-chromatography). Low concentrations of noble gases throughout the measuring campaign confirm depletion during capture. Kr and Ne were excluded after initial sampling tests, as their partial pressures were below the detection limit. He was too low (< 0.001 ppm) throughout for proper quantification with the miniRuedi, as peak variation could not be deciphered from background. This was confirmed in new, preliminary data analysed after the GHGT poster presentation, and thus He plots are excluded from this final summary. Further studies of single samples and continuous measurement of pre-capture variation (source inlet) are ongoing. Ar (150 – 650 ppm) contents were significantly higher compared to He, Kr, Ne, and was measured throughout the 5-day sampling period (Fig. 4). Some significant changes were observed (i.e. sudden increase/decrease).
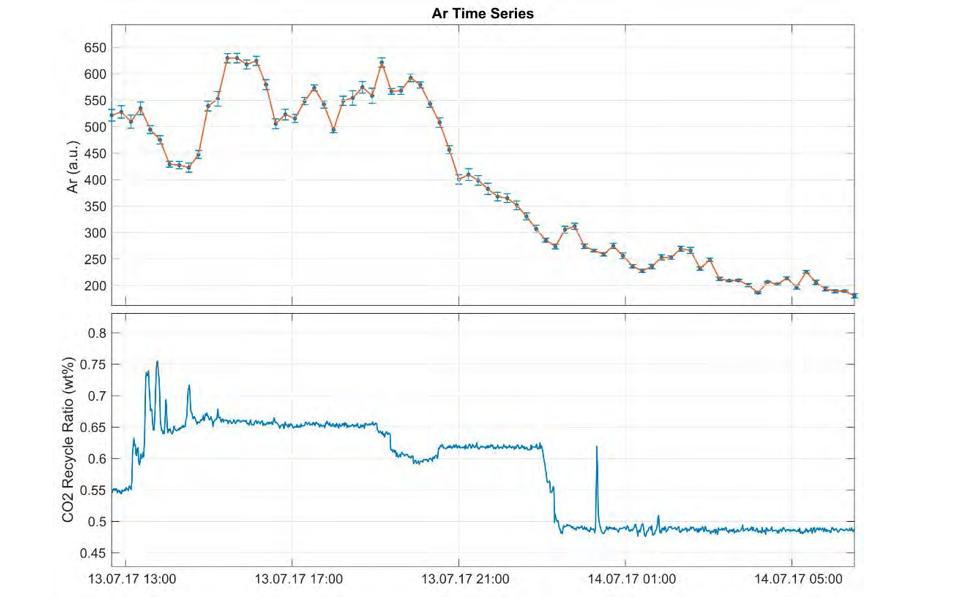
Fig. 4. Measured Ar concentrations (ppm) during the test period 10.07. – 15.07.2017 (upper) displaying co-variance with CO2 recycle ratio (wt %) at the TCM plant (lower).
The analytical error at sampling intervals of 10 – 15 minutes was acceptable, but the standard gas (air) used for calibration was found to be sub-optimal. Ar concentrations appear to be sensitive to changes in the recycle ratio and the capture process (Figs. 4, 5). Preliminary results from single samples confirm that He content is lower in the refinery gas compared to in the natural gas source, and concentrations are expected to vary according to relative contributions from natural gas versus refinery gas (Fig. 6). An observed decline in measured Ar concentrations coincided with reduction of CO2 recycling in the line, a scheduled process change (Fig. 4).
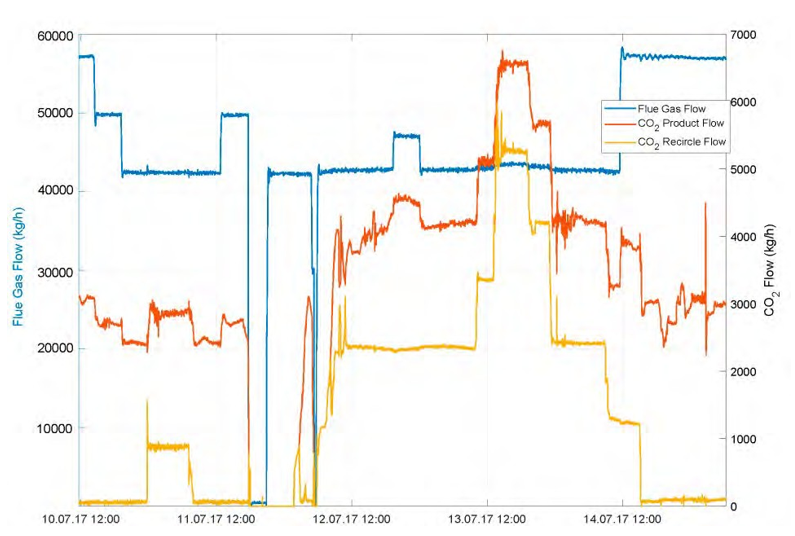
Fig 5. Gas flows (kg/h); flue gas, CO2 product and recycled CO2 .
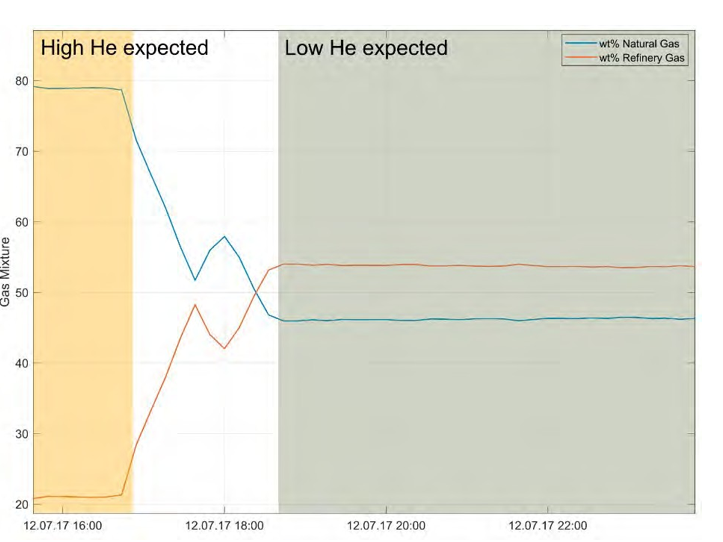
Fig. 6. Change in flue gas composition (wt % refinery gas vs. natural, detail from Fig. 5). Single samples indicate lower He concentrations in RFCC flue gas, such that concentration changes are expected for different mixing ratios.
5. Discussion
The test was successful in that the miniRuedi instrument ran continuously and variation in Ar concentrations were observed and documented. Up to 50 % change in relative concentrations of Ar was observed in the course of 5 days. This shows that there is noble gas variability, and that continuous monitoring is useful to ensure representative sampling in evaluation of inherent fingerprints and traceability. Preliminary single sample results show that concentrations are detectable with our technique before absorption (capture). During gas combustion and in the RFCC process, contamination with air occurs.
Assuming that tests during the capture processing (e.g. recycling) in a regularly working plant are not occurring, variations due to the capture process are expected to be minor. Thus noble gas variation related to source rather than process changes should be measured/detectable at the inlet. This is evaluated in ongoing studies.
The approach of in-line monitoring with a miniRuedi instrument is straightforward. However, optimization is necessary in order to reduce analytical uncertainty. Sample intervals of ~ 10 minutes were found suitable with respect to signal reading time and peak heights, and relative to sample resolution (gradual compositional changes). A customized calibration gas more similar to the CO2-product should be used in future tests for improved accuracy. Absolute variability in Ar concentrations was registered, and may be used to guide sampling for further chemical analysis and noble gas isotopic signature, ensuring a representative data set of single samples.
TCM is a test facility, and thus the CO2 product is not stored. There are, however, plans to establish a full value CCS chain in Norway, including storage of CO2 captured post-combustion [12]. Storage of CO2 from multiple sources (e.g. fossil fuels, cement, waste incineration) and different capture operations are challenging. Semi-continuous noble gas analysis may allow for pre-injection gas fingerprinting. With regards to detectability at potential leakage sites and separation of different anthropogenic type sources in reservoirs, however, some additional tracer gas may have to be added. At single-source sites (e.g. Snøhvit, Utsira), inherent fingerprints may suffice for source identification. For evaluation of source variability and the effect of the capture process on noble gas signatures, both the flue gas supply (inlet) and CO2 product (outlet) should be monitored simultaneously. Multiple capillary inlets to the miniRuedi instrument allows for semi-continous monitoring of several sample points. Total variation estimates are useful to guide sampling for isotopic analysis.
Ar concentrations displayed significant variation. During the Ar decrease shown in Fig. 4 the recycling rate was adjusted. Even though this is a change that may not occur in a running large-scale capture site, the dramatic decrease emphasizes that there is a response in the noble gas assembly related to capture process changes. During the test period measurements indicate that noble gases were heavily depleted after capture. He concentrations are significantly lower than atmospheric concentrations (~ 0.00524 hPa). Alternative sampling techniques, e.g. [13], may be tested in further research for gases currently below detection limit (i.e. He, Ne, Kr). Monitoring in-line variation and correlating with isotopic fractions from previous samples will reduce the need for costly and time-consuming lab analysis.
6. Conclusions
The utilization of noble gases as added and/or natural tracers in the context of CO2 storage monitoring is rather new. As CCS is being upscaled and put into practice, the need for combined and improved monitoring techniques is becoming evident. This feasibility study found that the miniRuedi [2] allows for frequent and accurate measurements of Ar abundance in captured CO2 from a post-combustion like facility (TCM). Preliminary single sample analyses indicate detectability also for continuous measurements of variation in He in the flue gas supply (source) before absorption and depletion during post-combustion capture. At pre-combustion sites, however, contamination with air is expected to be significantly lower, and considering He concentrations (enriched during radiogenic production) measured in North Sea gas fields [6], monitoring temporal variations for He in the CO2 product at pre-combustion capture sites is feasible. Documentation of variability in live gas streams and guided follow-up sampling for isotopic analysis in the lab will provide an important basis for consideration of noble gas fingerprints in monitoring schemes and leakage detection. This is a new approach that will provide knowledge of the uniqueness of noble gas fingerprints in the product stream from hydrocarbon production, CO2 capture operations and in the injection line for CO2 storage.
Acknowledgements
The ICO2P-project is funded by CLIMIT-Demo under grant #616220. The work was also partially funded by the SUCCES FME research center at the Department of Gesociences under grant 193825/S60 from the Research Council of Norway. The authors gratefully acknowledge the staff at TCM DA, Gassnova, Equinor, Shell and Total for their contribution and work at the TCM DA facility. The authors also gratefully acknowledge Gassnova, Equinor, Shell and Total as the owners of TCM DA for their financial support and contribution. We would also like to thank Eawag/ETH for making a miniRuedi instrument available. The project period is scheduled for 2016-2018. A continuation of this project is granted by RCN (#280551), and ICO2P-2 will continue until 2021, including a Ph.D. project. www.mn.uio.no/geo/english/research/projects/ico2p/
References
- CLIMIT-Demo feasibility study “ICO2P” under grant 193825/S60: www.mn.uio.no/geo/english/research/projects/ico2p/
- Brennwald, M. S., Schmidt, M., Oser, J., and Kipfer, R. (2016). A portable and autonomous mass spectrometric system for on-site environmental gas analysis. Env.Science & Technology, 50(24), 13455–13463.
- Gilfillan et al. (2008) The noble gas geochemistry of natural CO2 gas reservoirs from the Colorado Plateau and Rocky Mountain provinces, USA. Geochimica et Cosmologica Acta, 72(4), 1174-1198.
- Gilfillan et al. (2017) Using noble gas fingerprints at the Kerr Farm to assess CO2 leakage allegations linked to the Weyburn-Midale CO2 monitoring and storage project. Int. Jour. of Greenhouse Gas Control, 63, 215-225.
- Lollar, B. S., & Ballentine, C. J. (2009). Insights into deep carbon derived from noble gases. Nature Geosci. 2(8), 543.
- Ballentine, C. J., O’Nions, R. K., & Coleman, M. L. (1996). A Magnus opus: Helium, neon, and argon isotopes in a North Sea oilfield. Geochimica et Cosmochimica Acta, 60(5), 831-849.
- Györe et al. (2015). Tracing injected CO2 in the Cranfield enhanced oil recovery field (MS, USA) using He, Ne and Ar isotopes. Int. Jour. of Greenhouse Gas Control, 42, 554-561.
- Flude, S., Johnson, G., Gilfillan, S. M., & Haszeldine, R. S. (2016). Inherent tracers for CCS in sedimentary formations: composition and applications. Env. Science & Technology, 50(15), 7939-7955.
- de Koeijer, G., Enge, Y., Sanden, K., Graff, O. F., Falk-Pedersen, O., Amundsen, T., & Overå, S. (2011). CO2 Technology Centre Mongstad– Design, functionality and emissions of the amine plant. Energy Procedia, 4, 1207-1213.
- Thimsen et al. (2014). Results from MEA testing at the CO2 Post-Combstion CO2 capture testing. Part I: Post-Combstion CO2 capture testing methodology. Energy Procedia, 63, 5938-5958.
- Flø, N. E., Faramarzi, L., de Cazenove, T., Hvidsten, O. A., Morken, A. K., Hamborg, E. S., … & Fostås, B. F. (2017). Results from MEA degradation and reclaiming processes at the CO2 Technology Centre Mongstad. Energy Procedia, 114, 1307-1324.
- Gassnova (2018) Feasibility study for full scale CCS in Norway: www.gassnova.no/en/Documents/Feasibilitystudy…
- Manning, C. C., Stanley, R. H., & Lott III, D. E. (2016). Continuous measurements of dissolved Ne, Ar, Kr, and Xe ratios with a field- Deployable gas equilibration mass spectrometer. Analytical chemistry, 88(6), 3040-3048.
Dynamic Process Model Validation and Control of the Amine Plant at CO2 Technology Centre Mongstad (2016)
Rubén M. Montañés 1,* Nina E. Flø 2, and Lars O. Nord 1
1 Department of Energy and Process Engineering, NTNU—Norwegian University of Science and Technology, NO-7491 Trondheim, Norway; lars.nord@ntnu.no 2 CO2 Technology Center Mongstad, NO-5954 Mongstad, Norway; nflo@tcmda.no *Corresponding author
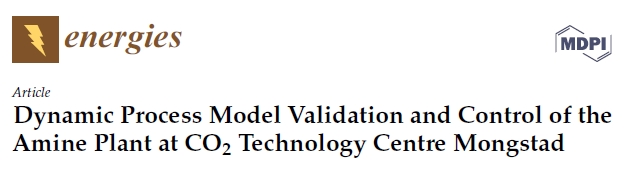
Abstract
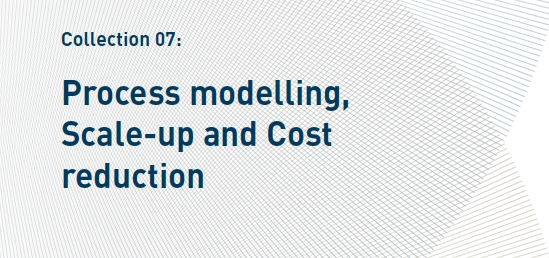
This paper presents a set of steady-state and transient data for dynamic process model validation of the chemical absorption process with monoethanolamine (MEA) for post-combustion CO2 capture of exhaust gas from a natural gas-fired power plant. The data selection includes a wide range of steady-state operating conditions and transient tests. A dynamic process model developed in the open physical modeling language Modelica is validated. The model is utilized to evaluate the open-loop transient performance at different loads of the plant, showing that pilot plant main process variables respond more slowly at lower operating loads of the plant, to step changes in main process inputs and disturbances. The performance of four decentralized control structures is evaluated, for fast load change transient events. Manipulation of reboiler duty to control CO2 capture ratio at the absorber’s inlet and rich solvent flow rate to control the stripper bottom solvent temperature showed the best performance.
1. Introduction
Carbon capture and storage (CCS) is a group of technologies that can significantly contribute to the reduction of anthropogenic CO2 emissions from thermal power generation and other carbon-intensive industries [1]. There are two commercial-scale coal-fired power plants with post-combustion CO2 capture (PCC) using amines being operated today, at Boundary Dam in Canada [2] and at Petra Nova project at the Parish Power Station in the US [3]. These projects prove the technical feasibility of the technology at commercial scale. Among the different options and technologies for CO2 capture in thermal power generation, post-combustion CO2 capture with chemical absorption is considered the more mature technology that can contribute to significantly reducing the carbon intensity (kgCO2/kWhel) of fossil-fueled thermal power plants. In future energy systems with a high penetration of renewable energy sources, the variability in demand and generation will introduce a change in the operating patterns of thermal power generation plants, which will have to change operating conditions [4–6]; there will also be a higher frequency of significant transient events including load changes, and start-up and shut-down events [7,8]. In this regard, Boot-Handford et al.’s carbon capture and storage update 2014 concludes that the financial case for CCS requires that it operates in a flexible manner and that load-following ability is extremely important to the long-term economics [9]. Among the different features of flexible operation of power plants with CCS, an important aspect is the transient behavior of the system when varying operating conditions. This means that efficient operation and emissions and the related operational costs during transient operation will gain importance. However, the operational experience from commercial-scale power plants with post combustion CO2 capture is scarce and the published transient pilot plant data from test campaigns is limited. Therefore, there is a need for the development of dynamic process models. Dynamic process models can contribute to developing the learning curve for flexible operation of PCC plants. These tools can assist in evaluating the feasibility of flexible operation strategies as well as design process configurations and operational strategies that can lead to the reduction of operational costs and increased revenue during power plant operation. The study of the transient performance with dynamic process models can contribute to identifying process bottlenecks and ease the process scale-up.
Dynamic process models allow the study of the open-loop transient performance of the plant [10], the evaluation of different process configurations and designs [11], the development and implementation of optimal control strategies [12–20], as well as the study of the plant behavior under different operational flexibility scenarios [21,22]. In addition, the power plant and the PCC unit can be treated as an integrated system and dynamic process models can be utilized to analyze the response of the capture unit to changes that occur upstream in the power plant [12,15,19,23–25]. Furthermore, the operational flexibility of the PCC plant can be improved with plant design or using control strategies [26–29]. The core purpose of dynamic process models is to capture the time-dependent behavior of the process under transient conditions. However, the validation of dynamic process models with experiments and pilot plant data is necessary in order to assess the reliability of simulation results. Kvamsdal et al. [30] developed a dynamic process model of a CO2 absorber column and used steady-state data from a pilot plant to validate liquid temperature profiles, capture ratio % and rich loading. That work highlighted the necessity of building up a dynamic process model of the integrated system (including stripper, lean/rich heat exchanger, mixing tank and main process equipment), to understand the complexities of dynamic operation of the plant. Gaspar and Cormos [31] developed a dynamic process model of the absorber/desorber process and validated with steady-state plant data. Several publications are available, in which the models were validated only with steady-state pilot plant data [11,32–35]. Biliyok et al. [36] presented a dynamic model validation study where transient data was driven by decrease in solvent flow rate to the absorber, fluctuating concentration of CO2 at absorber inlet and a varying absorber’s feed flue gas stream temperature to the absorber. A dynamic process model developed in Modelica language was validated with transient data from the Esbjerg pilot plant by Åkesson et al. [37]. That data consisted of the transient performance after one step-change in flue gas mass flow rate. An extensive review work by Bui et al. [38] concluded thatresearch efforts are required on producing transient pilot plant data.
More recent works have included validation of dynamic process models with transient plant data from pilot plants. A K-Spice model by Flø et al. was validated with pilot plant data from the Brindisi pilot plant [39]. Flø et al. [40] va lidated a dynamic process model of CO2 absorption process, developed in Matlab, with steady-state and transient pilot plant data from the Gløshaugen (Norwegian University of Science and Technology (NTNU)/SINTEF) pilot plant. Van de Haar et al. [41] conducted dynamic process model validation of a dynamic process model in Modelica with transient data from a pilot plant located at the site of the coal-fired Maasvlakte power plant in the Netherlands. Gaspar et al. [42] conducted model validation with transient data from two step changes in flue gas volumetric flow rate from the Esbjerg pilot plant. Other works include the validation of equilibrium-based models such as that of Dutta et al. [43]; or the work by Chinen et al. [44] which conducted dynamic process model validation of a process model in Aspen Plus® with transient plant data from the National Carbon Capture Center (NCCC) in the US. Manaf et al. [45] developed a data-driven black box mathematical model, based on transient pilot plant data, by means of system identification. In addition, dynamic process models have been developed to study the transient behavior of the chemical absorption CO2 capture process using piperazine (PZ) as chemical solvent [19,20]. It should be noted that the majority of work has been conducted for typical flue gas compositions from coal-based power plants with CO2 concentration around 12 vol % [38].
From the literature review it can be concluded that dynamic process model validation is a challenging process due to:
- The scarce availability of transient or dynamic pilot plant data.
- Most available data is found from small-scale pilot plants. That has implications for the reliability of simulation results when applying dynamic process models to scaled-up applications.
- The works involving transient data generally include the response of the plant to disturbances in a few process variables.
- Most of the validation work was done for flue gas with a typical CO2 content from coal-based power plants.
Flexible operation of PCC plants has been studied with pilot plant test facilities in test campaigns. Faber et al. [46] conducted open-loop step change responses at the Esbjerg pilot plant; this type of analysis helps in understanding the transient performance of the process. They concluded that the overall system acts as a buffer to perturbations at the plant inlet and that the coupled operation of the absorber/desorber unit led to fluctuations in the system when all parameters—flue gas and solvent mass flow rates and reboiler duty—are changed simultaneously. Bui et al. [47] presented a flexible operation campaign conducted at the Commonwealth Scientific and Industrial Research Organization (CSIRO)’s PCC pilot plant in Australian Gas Light Company (AGL) Loy Yang, a brown-coal-fired power station in Australia. The generated transient data included step changes in flue gas flow rate, solvent flow rate and steam pressure. The purpose of the study was to generate a set of data for validation of dynamic process models, and to gain insight into process behavior under varying operating conditions. A different approach was taken by Tait et al. [48] who conducted experiments that simulated flexible operation scenarios on a pilot plant to treat synthetic flue gas with a CO2 concentration of 4.3 vol%, typical of a natural gas combined cycle (NGCC) plant. Tests for transient operation have been conducted at the amine plant at CO2 Technology Center Mongstad (TCM DA). De Koeijer et al. presented two cases: a first case with controlled stop-restart of the plant, driven by a controlled stop of flue gas and steam sent to the PCC plant; and a second case with sudden stop of the blower upstream of the absorber [49]. Nevertheless, a limited amount of transient testing can be conducted during test campaigns. A thoroughly validated dynamic process model can help to study the transient performance, controllability, and flexible operation of the plant and process dynamics via dynamic process simulation.
In this work, a suitable set of steady-state and transient plant data, collected from a MEA campaign at CO2 Technology Center Mongstad, is selected for dynamic process model validation purposes. The plant was operated with flue gas from a natural gas fueled combined heat and power plant. The selected data is utilized to validate a dynamic process model of the amine-based CO2 absorption-desorption process at TCM DA. Then, the validated model is employed to carry out two case studies on the process dynamics of the TCM DA amine plant. In the first case study, the open-loop transient response of the pilot plant at different operating loads of the plant is analyzed. In the second case study, the performance of four decentralized control structures of TCM DA amine pilot plant is evaluated for fast disturbances in flue gas volumetric flow rate.
2. Materials and Methods
2.1 Plant Description
CO2 Technology Center Mongstad test site has a pilot-scale amine-based chemical absorption process plant. The amine plant can be configured to treat flue gas from a catalytic cracker from the Mongstad refinery, with CO2 content of around 13–14 vol%, typically found in flue gas from coal-fired power plants, and also to treat exhaust gas coming from a combined cycle gas turbine combined heat and power plant (CHP), with CO2 content of around 3.5 vol%. A fraction of the product CO2 mass flow rate can be re-circulated back upstream of the direct contact cooler (DCC) to increase the CO2 content, so CO2 concentrations of between 3.5 and 13–14 vol% could be fed to the plant to simulate the effects of exhaust gas recirculation [50]. Table 1 presents data of the main process equipment of TCM DA amine plant when configured to treat CHP flue gas, which has a total flue gas capacity of
60,000 Sm3/h and can capture around 80 ton CO2/day. Figure 1 shows a simplified process flow sheet of the amine plant at TCM DA when configured for CHP gas. A slipstream of exhaust gas is extracted from the CHP plant placed next to the TCM DA facility, and it consists of about 3% of the total exhaust gas. An induced draft blower is utilized to blow the flue gas flow. It has variable speed drives that allow the flue gas volumetric flow rate fed to the absorber column to be manipulated. Upstream the absorber column, a direct contact cooler cools down and saturates the flue gas with water, by means of a counter-current flow stream of water.
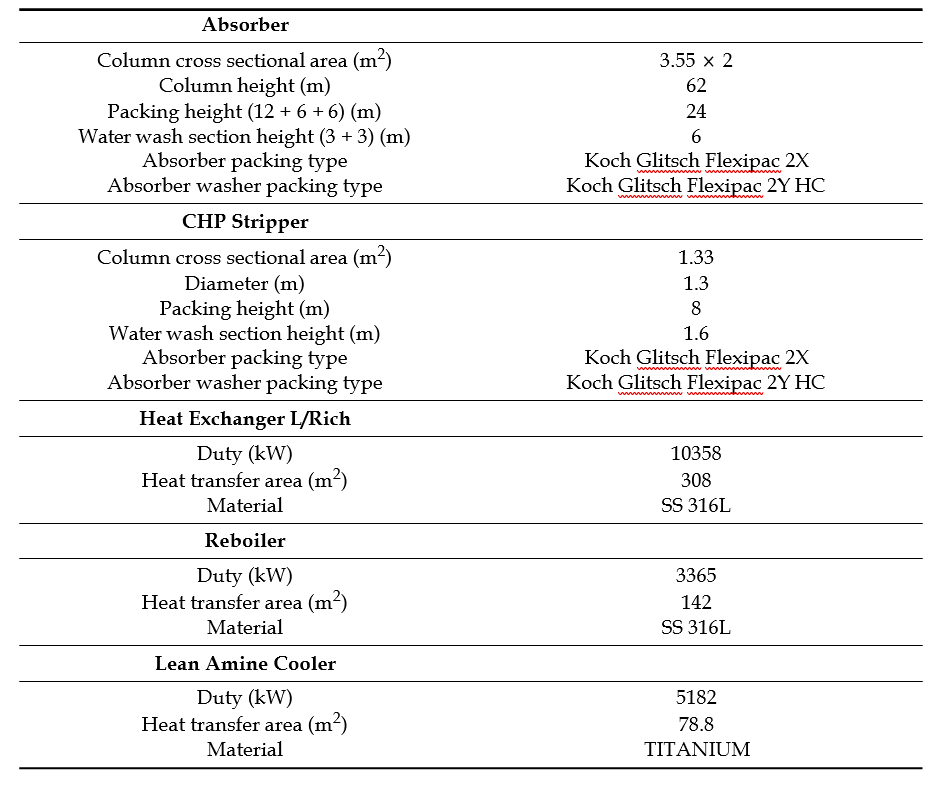
Table 1. Size and materials of main process equipment at the amine plant at TCM DA with CHP stripper configuration.
A chemical absorption process occurs in the absorber column, where the chemical solvent, flowing from top to bottom, meets the flue gas flowing in counter-current. The absorber column consists of a rectangular polypropylene-lined concrete column with a height of 62 m and a cross-section of 2 × 3.55 m. The absorber-packed sections consisting of Flexipac 2X (Koch-Glitsch Italia, Vimercate, Italy) structured stainless-steel packing are distributed from bottom to top in three sections of 12 m, 6 m and 6 m. Two water-wash systems are installed in the top of the absorption column, consisting of two sections of Flexipac 2Y HC (Koch-Glitsch Italia, Vimercate, Italy) structured stainless-steel packing. The water-wash sections limit emissions and are used to keep the water balance of the plant. The upper water-wash sections can be operated as acid wash [51]. In addition, the plant can be configured to use different packing heights in the absorber column resulting in 12, 18 or 24 m. This can be implemented at TCM plant by introducing all the lean solvent flow at 12 m of absorber packing, 18 m of absorber packing (12 + 6) m or 24 m of absorber packing (12 + 6 + 6) m.
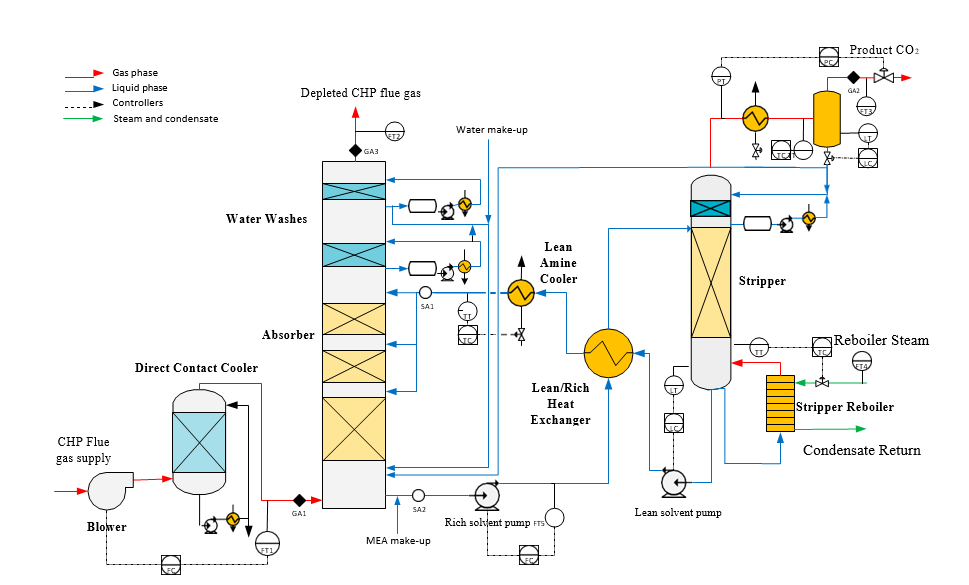
Figure 1. Simplified process flow sheet of the amine plant at CO2 Technology Center Mongstad, when configured to treat flue gas from a natural gas-fired power plant. The figure shows the location of some gas analyzers (GA), solvent analyzers (SA), flow transmitters (FT), pressure transmitters (PT), temperature transmitters (TT) and level transmitters (LT). The main process controllers of the regulatory control layer are shown, including flow controllers (FC), temperature controllers (TC), pressure controllers (PC) and level controllers (LC).
A 10.4 MW plate and frame heat exchanger is present at the plant where the cold rich amine solution coming from the absorber sump cools down the hot lean amine solution coming from the stripper. In addition, a 5.2 MW lean amine cooler is utilized to set the temperature of the lean solvent conducted to the top of the absorber packing sections, by using a stream of cooling water. The rich solvent is pumped to the top of the stripper column, where it meets the stripping vapors generated in the reboiler. The CHP stripper with overhead condenser system consists of an 8 m column of Koch Glitsch Flexipac 2X structured stainless-steel packing of 1.3-m-diameter, and a water-wash system with Koch Glitsch Flexipac 2Y HC structured stainless-steel packing of 1.6 m of height. The stripper reboiler consists of a 3.4 MW thermosiphon steam-driven system that supplies the heat required for the desorption process. The steam supplied to the reboiler comes from the refinery situated next to the TCM DA facility. Details on the steam supply system can be found in Faramarzi et al. [51].
2.2 Pilot Plant Configuration and Instrumentation
The TCM DA amine plant can be utilized to test various chemical solvents. In this work, the tests were conducted with 30 wt. % aqueous monoethanolamine (MEA). During the tests conducted in the test campaign, the responses and performance of the pilot plant were logged and extracted every 30 s. Gas composition was logged with gas analyzers at the inlet of the absorber, outlet of the absorber, and the product CO2. A gas chromatograph (GC) installed at TCM DA plant can measure concentrations of CO2, N2, H2O and O2 at the three locations in a nearly simultaneous manner, which is a desired feature for transient tests; refer to GA1, GA2 and GA3 in Figure 1. Details on gas analyzers and instrumentation at TCM DA plant can be found in [51].
Gas phase flow rates were measured at the plant during the tests. The flue gas volumetric flow rate fed to the absorber is measured with an ultra-sonic flow meter (FT1). As discussed by Faramarzi et al. in [51], the depleted flue gas flow meter (FT2) had a higher degree of variability than FT1, and some transients were observed on the FT2 measurement that were not explained by changes in process parameters at the plant. Therefore the depleted flue gas flow rate was calculated in the test campaign by considering that all O2 and N2 fed to the absorber goes out of the plant with the depleted flue gas. The cooled product CO2 discharge flow (FT3) was measured with a vortex flow meter. Other flow rates measured at the plant include the steam fed to the reboiler, the lean amine flow rate at the absorber inlet and the rich amine flow rate at the absorber outlet. For flue gas flow meters, the standard conditions are 15 ◦C and 101.3 kPa [51].
Pressures and pressure drops at different components of the plant were logged. In addition, main process temperatures were logged. For process model validation, it is common to assess the model prediction of the absorber and stripper temperature profiles. Within the absorber and stripper columns of TCM DA’s amine plant there are four temperature sensors distributed in the radial plane per meter of packing in the axial direction. Thus, there are 96 temperature sensors within packed segments of absorber column and 28 temperature sensors within the packed segment in the stripper column. These measurements allow the creation of clear temperature profiles of the absorber and stripper columns in the axial direction (at each column height, the resulting temperature value is the average of the four measurements distributed in the radial plane).
Online solvent analysis measurements (SA) were taken at the inlet (SA1) and outlet of the absorber (SA2); refer to Figure 1. The measurements include pH, density and conductivity. In addition, solvent samples were regularly taken manually and analyzed onsite. These analyses allow MEA concentration and CO2 loadings to be calculated at the sampling points on a periodic basis. The actual reboiler duty was estimated as suggested in Thimsen et al. [52]. Equation (1) shows the calculation of the actual reboiler duty, where Fsteam is the logged measurement data of steam mass flow rate (refer to FT4 in Figure 1), Tc is the condensate temperature, Tg is the superheated steam inlet temperature, pg is the steam pressure at inlet, and pc is the condensate pressure. Enthalpy was calculated with the use of accurate steam tables, with the condensate at the reboiler outlet assumed to be saturated liquid at Tc or pc. The specific reboiler duty (SRD) in kJ/kgCO2 is calculated as in Equation (2), where Fprod is the CO2 rich product mass flow rate; refer to FT3 in Figure 1.
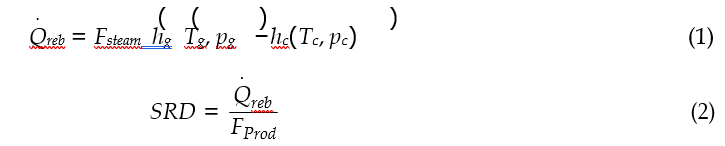
During the tests presented in this work, the averaged total inventory of aqueous MEA was around 38.2 m3. Averaged values of liquid hold-ups and its distribution at different components of the plant during the steady-state tests included in this work are presented in Table 2. Detailed data on solvent inventory distribution throughout the plant is of importance in order to obtain suitable dynamic process simulation results. The regulatory control layer of the plant was active during the tests conducted in the MEA campaign. The main control loops of the regulatory control layer are presented in Figure 1. Note that the actual regulatory control layer of the amine plant at TCM DA is more complex and includes more control loops for auxiliary equipment, stable and safe operation of the plant, and start-up and shut-down sequences. The control loops included here are those the authors found relevant for the purposes of dynamic process modeling and simulation of this plant during online operation, and considering the time scales of interest for process operation.
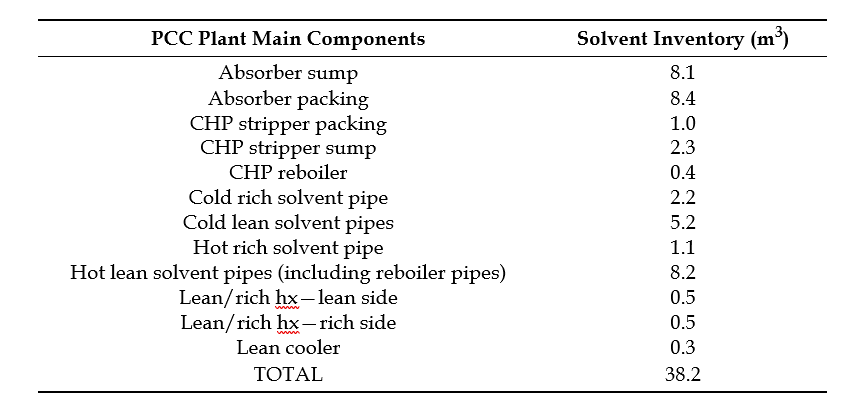
2.3 Dynamic Process Model
Dynamic process modeling was carried out by means of the physical modeling language Modelica [53]. Modelica allows development of systems of differential and algebraic equations that represent the physical phenomena occurring in the different components of the system. The process models of the equipment typically found in a chemical absorption plant were obtained from a Modelica library called Gas Liquid Contactors (Modelon AB, Lund, Sweden) [54], and the commercial tool Dymola (Dassault Systèmes, Vélizy-Villacoublay, France) [55] was utilized to develop the models and carry out the simulations. The component models include absorber and stripper columns, sumps, lean and rich heat exchanger, stripper reboiler, overhead condenser, condensers, pipe models, pumps, valves, measurements and controllers. The dynamic process model of the amine plant at TCM DA presented in Figure 1 was developed by parameterizing, modifying and connecting the different models. For this purpose, the main process equipment, size, geometry and materials were considered; refer to Table 1. A key aspect for obtaining suitable dynamic simulation results is the consideration of the distribution of solvent inventory at the different equipment of the plant. Therefore, solvent inventory distribution was implemented in the dynamic process model; refer to Table 2. Finally, the equivalent regulatory control layer of the plant was applied in the dynamic process model; discussed later in Section 5.2. The models contained in the library have been presented elsewhere [56,57]; therefore only an overview of the models is presented in the following. Numerical integration of the resulting system of differential and algebraic equations was carried out in Dymola with the differential algebraic system solver (DASSL) implemented in Dymola [55]. The main assumptions applied are [56]:
- All chemical reactions occur in the liquid phase and are assumed to be in equilibrium.
- The flue gas into the absorber contains only CO2, O2, H2O and N2.
- MEA is non-volatile and not present in the gas phase.
- The total amount of liquid in the column is defined as the packing hold-up and the sump liquid hold-up.
- The reboiler is modeled as an equilibrium flash stage.
- The liquid in the column sumps and other large volumes are assumed to be ideally mixed.
- Mass and heat transfer between liquid and gas phase is restricted to packed section.
- Negligible temperature difference between the liquid bulk and interface to gas phase.
- No storage of mass and energy in the gas phase.
- All liquid from the packing bottom in the stripper is fed to the reboiler with a constant liquid level.
- Constant target packing hold-up.
The models of the absorber and stripper columns are developed based on the two-film theory; therefore, at the gas and liquid interface thermodynamic equilibrium is assumed. Interface mass transfer phenomena is modeled in packed sections with a rate-based approach with enhancement factor E [30], which takes into account the enhanced mass transfer due to chemical reactions; refer to Equations (3) and (4), where ci,if and ci,b are molar concentrations at liquid bulk and interface, Aif is the contact area, ki are the mass transfer coefficients by Onda [58], T is the bulk phase temperature, and pi are the partial pressures of the species in the gas phase. The pseudo-first order enhancement factor E is calculated as in Equation (5), where kCO2 is the overall reaction constant for CO2 and CMEA the molar free MEA-concentration taken from [59], the diffusivity DCO2 of CO2 in aqueous MEA is calculated by the Stokes-Einstein relation and the diffusivity of CO2 in water from [60]. Cef is a pre-multiplying coefficient for calibration of enhancement factor. The packing characteristics of Koch Glitsch Flexipac 2X were considered for parameterizing the packing segments of the dynamic process model for absorber and stripper columns, with a surface area of 225 m2/m3 and a void fraction of 0.97.
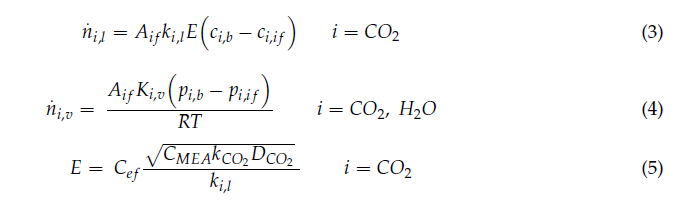
Phase equilibrium at the gas-liquid interface is calculated as in Equations (6) and (7), where the solubility of CO2 in water is considered by Henry’s law, with Hei from [61]; activity coefficients γi are implemented from [61]; chemical equilibrium is assumed at the interface and liquid bulk, and the chemical equilibrium constants Ki implemented in the process model are obtained from Böttinger [61]. The Van’t Hoff equation is utilized in order to infer the heats of reaction ∆Hr from the equilibrium constant; refer to Equation (8). The Chilton-Colburn analogy was employed to correlate sensible heat transfer between phases with the gas phase mass transfer coefficient. Latent heat connected to the transferred mass flow from one phase to the other is considered in the specific enthalpies of the individual species. The heat of evaporation and heat of solution are a function of temperature but are considered constant with solvent CO2 loading. The gas phase model assumes ideal gas law, and the pressure of the column p is determined by the gas phase pressure drop.
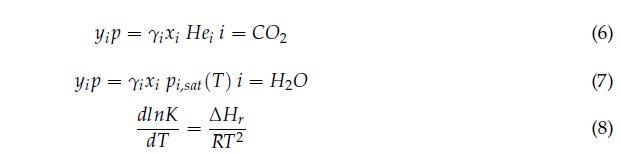
The lean-rich heat exchanger is modeled as a static heat exchanger model with the ε-NTU (effectiveness—number of thermal units), and pure transport delay models are used to account for dead times included by the solvent hold-up within piping’ volumes.
At the top of the absorber column a washer model is implemented, consisting of a volume model with phase separation that saturates the gas with water at the targeted temperature. A make-up stream of water is injected in the absorber sump to keep the H2O mass balance of the system. MEA is assumed non-volatile in the model and therefore it is only present in the liquid phase. However, in the actual plant make-up MEA is required for operation and it is injected upstream the rich amine pump; refer to Figure 1.
3. Steady-State Validation of Dynamic Process Model
3.1 Steady-State Operating Cases
A test campaign was conducted at the amine plant at TCM DA using MEA, operated from 6 July until 17 October 2015. Table 3 shows the steady-state cases generated during the test campaign that were used in this work for dynamic process model validation purposes. The plant was operated with 30 wt. % MEA for all cases. The objective was to select a set of steady-state cases from the MEA campaign that could represent a wide range of steady-state operating conditions, including data from full capacity of volumetric flow rate fed to the absorber column. The steady-state cases were generated by varying the set points of the main pilot plant inputs, namely solvent circulation flow rate Fsolv (refer to FT5 in Figure 1), reboiler duty (Qreb), and flue gas volumetric flow rate (Fgas).
The steady-state cases represent a variation in operating conditions of the plant, especially on the flue gas volumetric flow rate load of the absorber, CO2 capture rate, L/G ratio in the absorber and absorber packing height. Cases 1 to 5 are operated at absorber full flue gas capacity of around 60,000 Sm3/h. A similar mass-based L/G ratio, of around 0.89, is kept in the absorber column during the steady-state operating cases with full capacity, with the exception of Case 4, where it is changed to 0.8, by varying the rich solvent mass flow rate. The main process variability in these cases is the change in reboiler duty, with CO2 capture rate ranging from 85 to 68%. CO2 capture rate was calculated with the method 1 described by Thimsen et al. [52]; refer to Equation (9), where Fprod refers to the product CO2 flow rate (FT3 in Figure 1), and XCO2 is the mass fraction of CO2 in the absorber inlet (measured at GA1 in Figure 1). Note that here CO2 capture rate has been named Des as it defines the desorption ratio utilized in Section 5.2. In addition, Cases 2 to 5 were operated with 18 m absorber packing, i.e., the uppermost absorber-packing segment is kept dry. Cases 6 to 10 are operated with 24 m absorber packing and the absorber column at 80% volumetric flue gas flow rate capacity. The mass-based L/G ratios on the absorber range from 1.34 to 0.75 for Cases 6 to 10, by varying solvent circulation mass flow rate. The capture rate is kept constant at around 85% by varying the reboiler duty.
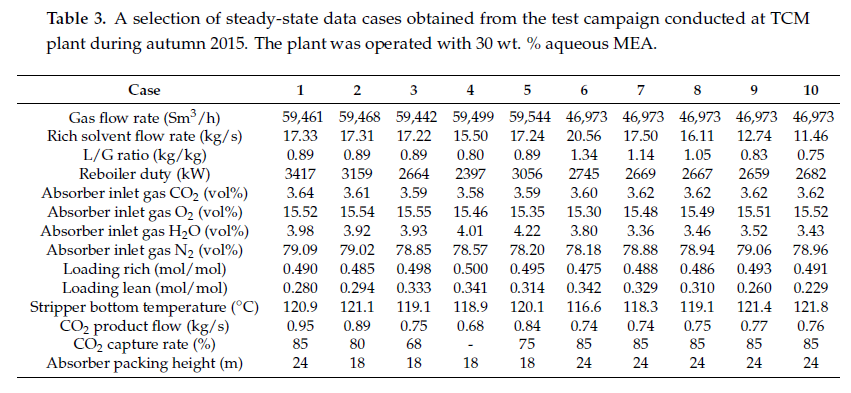
plant during autumn 2015. The plant was operated with 30 wt. % aqueous MEA.
The first series of tests during the MEA campaign were dedicated to verification of mass balances of the plant [50]. CO2 mass balance gives results close to 100%, and Gjernes et al. [50] conclude that CO2 mass balance based on gas phase can be maintained at a level better than 100 ± 5%. In this work, the suggested method in [50] was used during data selection in order to ensure that the steady-state data cases presented in Table 3 have acceptable CO2 mass balance.
In order to develop the overall dynamic process model of the plant, the steady-state data for Case 1, refer to Table 3, was used as a reference to calibrate the dynamic process model, and the main outputs from the model simulations were compared with the plant data. This data set was chosen since it represents the baseline operating conditions of the amine plant at TCM DA when using aqueous MEA as chemical solvent, as presented in Faramarzi et al. [51]. The models of the different subsystems of the plant consisting of (i) absorber column; (ii) lean/rich heat exchanger; and (iii) stripper column with overhead condenser and reboiler were calibrated separately, and then linked to form the overall dynamic process model. The model was calibrated by tuning a pre-multiplying coefficient Cef for the enhancement factor E. It was set to 0.28 in absorber packed segments and 0.01 in stripper packed segments. The validation section included in this work extends on work conducted previously [62].
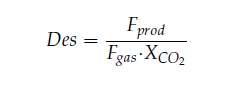
3.2 Validation Results of Dynamic Process Model with Steady-State Plant Data
The results from the simulated dynamic process model for the steady-state operating cases, described in Section 3.1, are displayed in Table 4. The results shown are for main process variables during pilot plant operation, namely CO2 lean (Ll) and rich (Lr) loadings, product CO2 flow rate (Fprod), specific reboiler duty (SRD) and stripper bottom temperature Tstr. Possible deviations in dynamic process model prediction arise from errors related to measurement uncertainty and to modeling uncertainty, the latter being related to the fact that a physical model is always a simplification of reality. This means that it is natural to observe some deviation in the prediction of the dynamic process model simulation. Therefore, it is of importance to quantify these errors so that they are kept within reasonable bounds. The absolute percentage errors (AP) and the mean absolute percentage errors (MAP) are calculated as in Equations (10) and (11), where xm is the value of the process variable predicted by the process model simulation, xp is the value of the process variable measured at the pilot plant at the given steady-state operation case, and n is the number of steady-state cases studied.
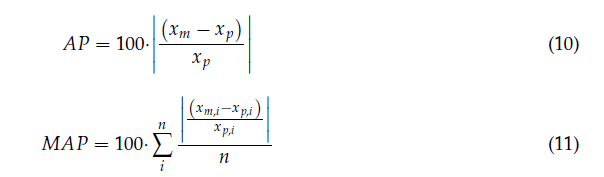
The results for lean CO2 loading are presented in Figure 2 with a parity plot, where ±5% and ±10% error lines are also shown. It is clear that the dynamic process model under-predicts lean loading for most of the cases, with a MAP < 6.6%. In addition, Figure 2 shows the parity plot for CO2 product flow rate; in this case, the CO2 product flow rate is also under-predicted by the dynamic process model, with a MAP < 5.3%. Figure 3 shows the parity plot for stripper bottom temperature, with the ±2% error lines plotted; stripper bottom temperature Tstr presented a MAP < 1%. From the parity plots, one can observe that, despite the errors found in the absolute values predicted by the dynamic process model with respect to the reference plant data, the dynamic process model can predict the variability in the main process variables for a wide range of steady-state operating conditions.
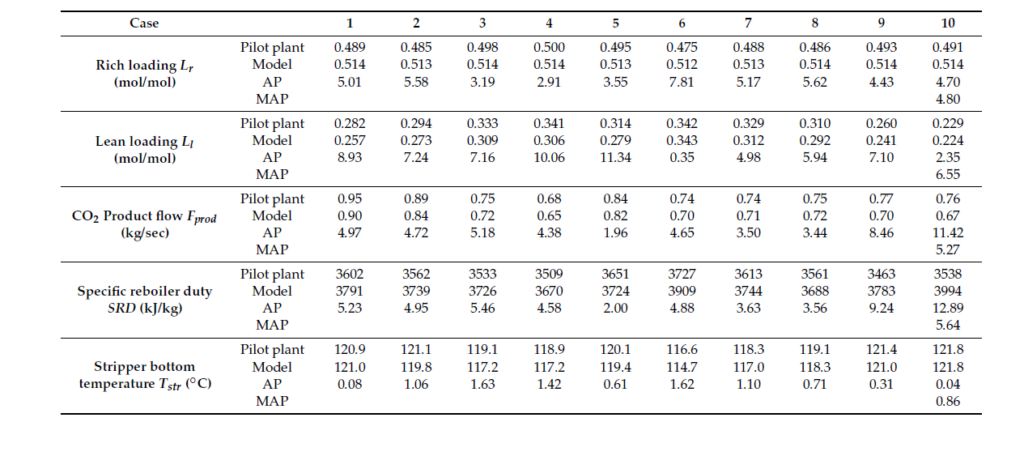
Table 4. Results from dynamic process simulation of the amine plant at TCM for the 10 steady-state operation cases. The pilot plant data for solvent CO2 lean loading (Ll), solvent CO2 rich loading (Lr), CO2 product flow rate (Fprod), specific reboiler duty (SRD) and stripper bottom temperature (Tstr) are shown. In addition, the model prediction during steady-state simulation for the same process variables is shown. Calculated absolute percentage errors (AP) and mean absolute percentage errors (MAP) between pilot plant measurements and simulated model predictions for the 10 steady-state operating cases are presented.
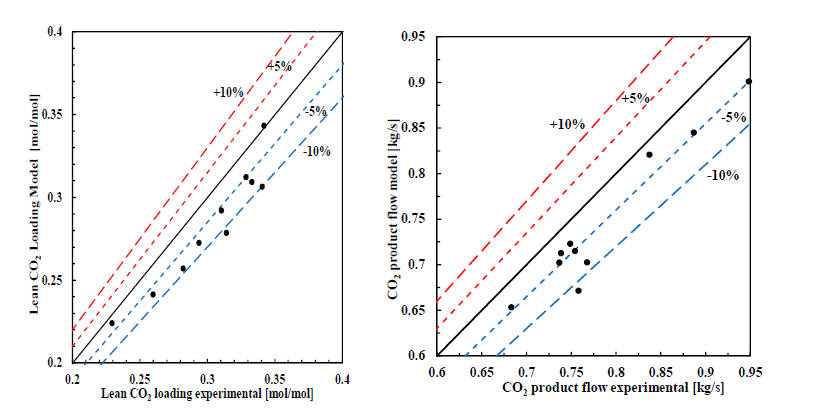
Figure 2. Parity plots of lean CO2 loading (left) and CO2 product flow rate (right). Lines for +10%,
+5%, −5% and −10% percentage error are shown. The mean percentage error is <6.6% for CO2 lean
Energileos a2d01in7,g 1a0,n xd F<O5R.3 P%EEfoRr RpErVoIdEuWc t CO2 flow rate (Fprod).
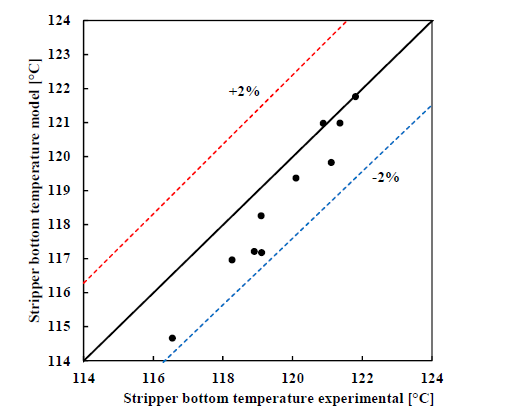
Figure 3. Parity plot for stripper bottom temperature for the 10 steady-state operation cases. Lines for +2% and −2% percentage errors are shown. The mean percentage error is 0.86 for stripper bottom temperature.
Temperature within absorber and stripper column is an important process variable since it affects phase equilibrium at liquid and gas-liquid interface. Some important model parameters and thermophysical properties depend on temperature, including heat capacity, water heat of condensation, heats of reaction, equilibrium constants and CO2 solubility. Therefore, it is desirable that the dynamic process model can predict with good accuracy absorber and stripper columns’ temperature profiles. Figure 4 shows the comparison between the pilot plant temperature profiles of the absorber and desorber columns with the predictions from the simulation of the dynamic process models. Two steady-state operating cases are presented: Case 1 (Table 3) with absorber flue gas volumetric capacity of 100%, mass-based L/G ratio of 0.89 and capture target of 85%; and Case 6 (refer to Table 3) with 80% flue gas volumetric capacity, mass-based L/G ratio of 1.34 and capture target of 85%. Both cases were operated with 24 m of wet absorber packing, and represent two operating cases with different flue gas capacities and L/G ratios.
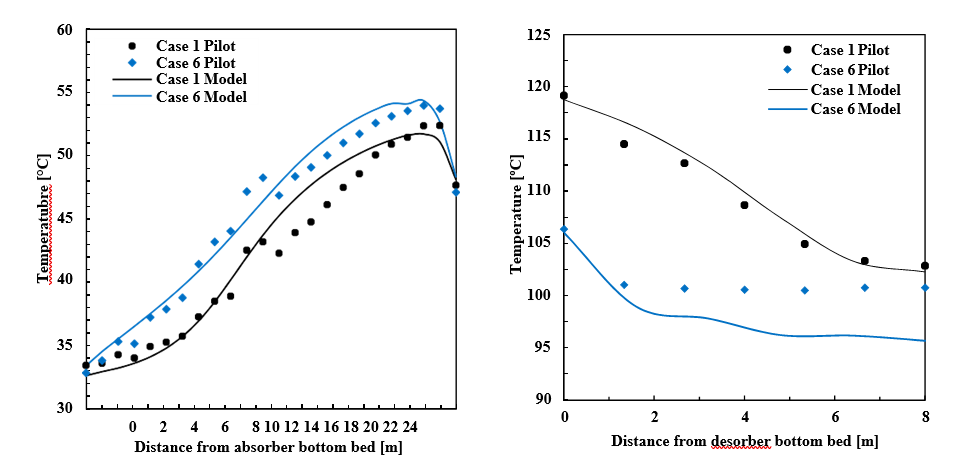
Validation of absorber and stripper temperature profiles is normally considered a challenging task for several reasons. At TCM DA the temperature profiles are the resulting averaged values of the 4 measurements distributed radially in a given axial position within the column; refer to Section 3. A given pilot plant temperature value presented in Figure 4 is the resulting average over time during one hour of steady-state operating conditions, of the averaged 4 temperature measurements radially distributed within the absorber or stripper column, at the given axial position of the column. The individual temperature measurements are considered reliable and the resulting temperature profiles are reasonable. However, some sensors are located closer to the center of the packing while others closer to the wall. This results in a maximum variation (<6 ◦C) which is observed between the measurements in the same radial plane, which depends on operating conditions and is different at different radial planes. Based on the results presented in Figure 4, the dynamic process model can properly predict absorber and stripper column temperature profiles with sufficient accuracy considering the purpose of application. Absorber temperature profiles predicted by the model show a good agreement with the experimental pilot plant data, and the model is capable of properly predicting the trends in temperature along the column. The absorber temperature profiles have a mean absolute percentage error (<2.5%) for Case 1 and (<2.1%) for Case 6, which is within the observed maximum variability of the temperature measurements in a given radial plane. In addition, desorber temperature profiles have a mean average error (<0.6%) for Case 1 and (<3.6%) for Case 6. It is the desorber temperature profile for Case 6 that presents the less accurate prediction. In addition, it can be concluded that the process model is capable of properly predicting the variation of temperature profiles for various steady-state operating conditions.
4. Validation of Dynamic Process Model with Transient Plant Data
For dynamic process model validation purposes transient tests are conducted by means of open-loop step changes in the main process inputs to the plant. The transient behavior occurs between the initial steady-state operating conditions until the new steady-state operating conditions are reached. In this work, the experiments consist of set-point changes in rich solvent flow rate, flue gas volumetric flow rate fed to the absorber and reboiler duty. The output trajectories of main process variables are observed and compared with the model output trajectories. In order to obtain good sets of data for validation, it is desired to apply the step changes in plant inputs in a non-simultaneous manner. However, this is not normally easy to implement in practice. In order to compare the pilot plant experimental output trajectories with the output trajectories predicted by the dynamic process models, input trajectories were utilized in the dynamic simulations. This means that the measured time series of the inputs applied to the pilot plant during the tests were applied as disturbances or inputs to the dynamic process model; refer to Figures 5a, 6a and 7a. During the three tests, the regulatory control layer of the plant was active. In Figures 5 and 6, the time t = 0 corresponds to the point from which the set point of flue gas volumetric flow rate was changed. In Figure 7 the time t = 0 is the point from when the set point of rich solvent flow rate was changed.
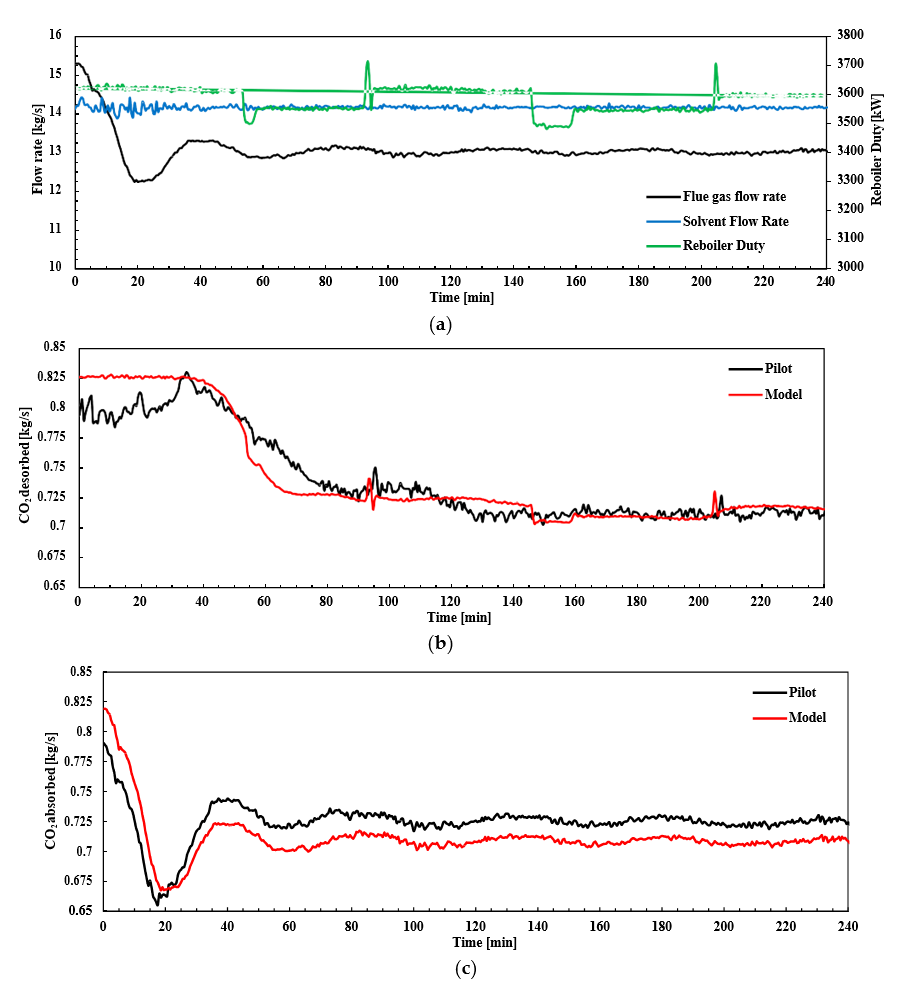
Figure 5. (a) Main inputs to the plant for test with flue gas flow rate set-point reduction (kg/s). Rich solvent flow rate from absorber (kg/s) and reboiler duty (kW); (b) Pilot plant transient response and model output trajectory for CO2 product flow rate Fprod or CO2 desorbed (refer to FT3 in Figure 1); (c) Pilot plant transient response and model output trajectory for CO2 absorbed in absorber column,
refer to Equation (11). The time t = 0 corresponds to the point from which the set point of flue gas volumetric flow rate was changed.
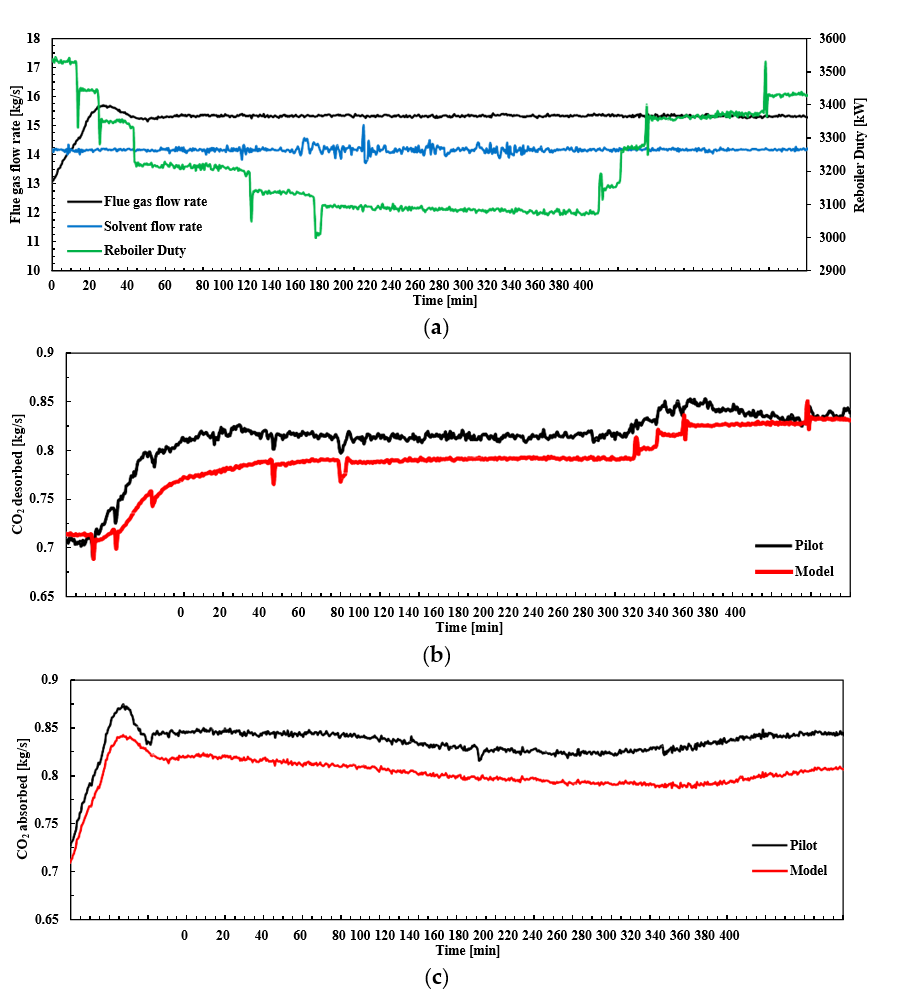
Figure 6. (a) Main inputs to the plant for test with flue gas flow rate set-point increase (kg/s). Rich solvent flow rate from absorber (kg/s) and reboiler duty (kW); (b) Pilot plant transient response and model output trajectory for CO2 product flow rate Fprod or CO2 desorbed (refer to FT3 in Figure 1); (c) Pilot plant transient response and model output trajectory for CO2 absorbed in absorber column,
refer to Equation (11). The time t = 0 corresponds to the point from which the set point of flue gas volumetric flow rate was changed.
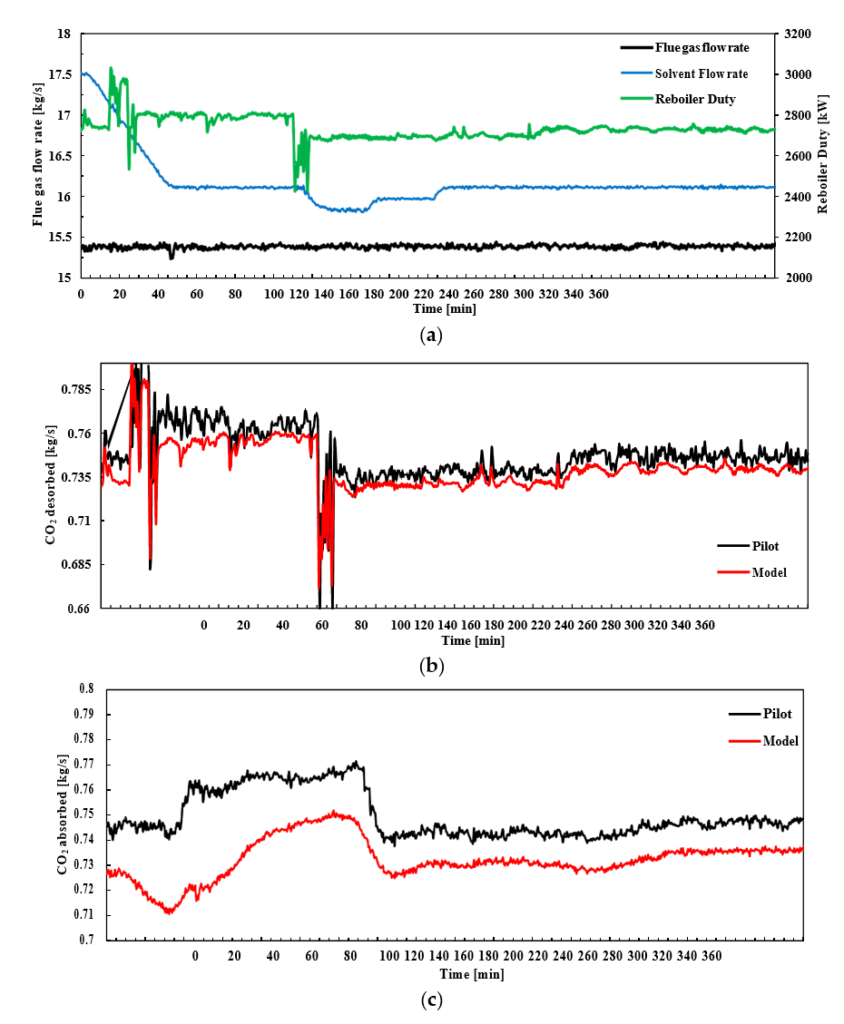
Figure 7. (a) Main inputs to the plant. Flue gas volumetric flow rate set-point change increase (kg/s). Rich solvent flow rate from absorber (kg/s) and steam flow to reboiler (kg/s); (b) Pilot plant transient response and model output trajectory for CO2 product flow rate Fprod or CO2 desorbed (refer to FT3 in Figure 1); (c) Pilot plant response in CO2 absorbed mass flow rate (kg/s). The time t = 0 corresponds to the point from which the set point of rich solvent flow rate was changed.
4.1 Flue Gas Flow Rate Ramp-Down
The main disturbance applied in this transient test consisted of a reduction in flue gas volumetric flow rate at the inlet of the absorber. It was implemented at TCM DA pilot plant by changing the set point of the blower cascade controller from 47,000 Sm3 to 40,000 Sm3; refer to FT1 in Figure 1. This corresponds with flue gas volumetric flow capacities in the absorber column of 80% and 67% respectively. Figure 5a shows the three main inputs of the plant for this test. During the test, reboiler duty was changed in steps around the value of 3550 kW; this might be due to the effects of the regulatory control layer on steam mass flow rate. The solvent mass flow rate had small amplitude oscillations around the set point.
CO2,abs = Fgas·XCO2 − Fdepleted·XCO2,out (12)
Figure 5b,c show the output trajectories of CO2 product flow rate (or CO2 desorbed) and CO2 absorbed to the disturbance applied in this test. CO2 absorbed is calculated as the difference between CO2 mass flow rate at the absorber inlet and the CO2 mass flow rate leaving the absorber with the depleted flue gas at the top of the absorber; refer to Equation (12). In Figure 5b, a dead time of around 40 min was observed, i.e., no significant changes are found in the CO2 desorbed until around 40 min after the disturbance was applied to the pilot plant. In addition, the plant did not reach steady-state operating conditions until around 4 h later. As shown in Figure 5c, there is not significant dead time in the response of CO2 absorbed. The difference observed between the output trajectories is characteristic of the coupled transient performance of the absorber and stripper columns. Figure 5b,c shows that the process model is capable of predicting the main process dynamics for CO2 product mass flow rate (CO2 desorbed), including an adequate prediction of dead times and stabilization time. In addition, the CO2 absorbed transient performance trends are predicted in a satisfactory manner.
4.2 Flue Gas Flow Rate Ramp-Up and Step Changes in Reboiler Duty
These tests consist of combined input changes to the plant in terms of flue gas volumetric flow rate and reboiler duty. A set-point increase of the flue gas volumetric flow rate fed to the absorber from 40,000 to 47,000 Sm3/h was applied. This corresponds with 67% and 80% of the absorber column capacity, respectively. In addition, step-changes in reboiler duty were applied during the transient test. Figure 6a shows the three main inputs of the plant during the test. Figure 6b,c show the CO2 product flow and CO2 absorbed for the model and the pilot plant data. In this test a dead time of around 20 min in the response of CO2 desorbed was observed. This confirms the buffering effect by the chemical process in terms of the response of CO2 desorbed when the flue gas volumetric flow rate is changed. There is evidence to support this observation in previous pilot plant studies [46–48]. The delay in the response is partly attributed to solvent circulation time and the redistribution of liquid. Despite the steady-state offset shown on CO2 absorbed in Figure 6b, a good prediction of the main transient response is seen. It is possible that the reduction in reboiler duty at around 10 min flattens out the response in CO2 product flow rate.
4.3 Solvent Flow Rate Ramp-Down
In this test, the plant is operated in steady-state until the rich solvent mass flow rate set point is ramped down from around 17.5 kg/s to around 16.1 kg/s; refer to FT5 in Figure 1. The reboiler duty and flue gas volumetric flow rate were intended to be kept constant. Figure 7a shows the three main inputs of the plant during this transient test. In addition, the pilot plant performance in terms of product CO2 mass flow Fprod (or CO2 desorbed) and absorbed CO2 flow rate are presented, together with the dynamic process model simulations for this test. Again, a satisfactory agreement is found between the plant trajectories and the output trajectories predicted by the dynamic process model.
From the three transient tests presented above, it can be concluded that the dynamic process model predicts the transient trends of the main output trajectories of the process for different inputs to the plant. In addition, the dead times and stabilization times of the process are properly predicted by the dynamic process models, despite the steady-state deviations observed and already quantified in Section 3.2. This means that the dynamic process model is suitable for simulation studies at the plant scale, including dynamic process simulations to analyze the plant transient performance, and for control tuning and advanced control layer design, including control structure studies.
5. Case Study: Open-Loop Performance and Decentralized Control Structures
5.1 Open-Loop Step Responses at Different Plant Flue Gas Capacities
A power plant operated in a power market with a high penetration of renewables will most likely be operated in load-following mode [7,63]. This means that the power plant with PCC will be operated during a significant amount of its lifetime at part loads. In the case of a natural gas combined cycle power plant with post-combustion CO2 capture it means that, at part-load operation, the gas turbine (GT) load will be reduced, generating a reduced mass flow rate of flue gas that would be conducted to the PCC unit. The purpose of this case study is to investigate the transient performance of the PCC pilot plant via dynamic process simulation by implementing open-loop step changes to the dynamic process model, and to compare the response of the plant at different part-load operating points, defined by different mass flow rates of flue gas to be treated. The analysis will assess the transient response of the plant to multiple and non-simultaneous step changes in three key inputs to the plant, namely
- flue gas flow rate Fgas (ii) solvent flow rate Fsolv; and (iii) reboiler duty Qreb, at different flue gas
mass flow rate capacities of the plant. In order to define the part-load operating points, a decentralized control structure was utilized, in which reboiler duty was the manipulated variable to control stripper bottom temperature Tstr to 120.9 ◦C, and the solvent flow rate was the manipulated variable to control CO2 capture ratio Cap to 0.85, as defined in Equation (13). When operating the plant at different flue gas mass flow rates, corresponding to 100%, 80% and 60% of nominal mass flow rate, this results in the three steady-state operating points presented in Tables 5 and 6. The control structure is defined as control structure A in Table 7.
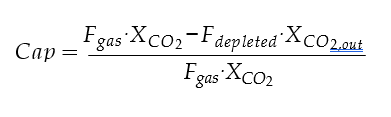
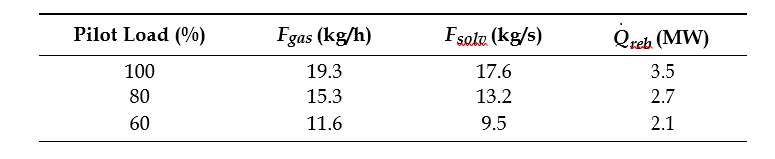
Table 5. Simulated pilot plant inputs’ set points for the three operating points to be studied, corresponding to 100%, 80% and 60% of flue gas mass flow rate capacity of the pilot plant. With Cap = 0.85 and Tstr = 120.9 ◦C for all cases.
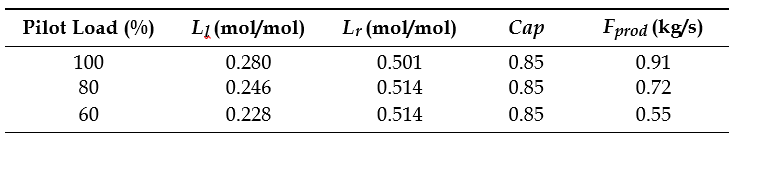
Table 6. Simulated pilot plant values for the process variables, lean CO2 loading Ll, rich CO2 loading Lr, CO2 capture ratio Cap and CO2 product flow rate, at three different operating points of the plant, corresponding to 100%, 80% and 60% of flue gas mass flow rate capacity of the pilot plant. With Cap = 0.85 and Tstr = 120.9 ◦C for all cases.
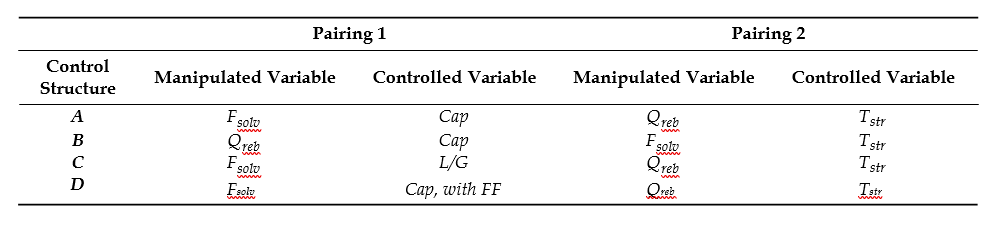
Table 7. Control structures for the supervisory control layer of the TCM amine plant. Key manipulated variables (MVs) are solvent flow rate Fsolv and reboiler duty Qreb. Controlled variables are CO2 capture ratio Cap to 85%, defined in Equation (12), and stripper bottom temperature Tstr to 120.9 ◦C. Control structure D controls Cap via a feed forward FF controller.
The open-loop response was studied for the process variables (i) CO2 absorbed CO2,abs, in Equation (11); (ii) CO2 desorbed CO2,abs (or Fprod); (iii) lean CO2 loading Ll at the inlet of the absorber; and (iv) rich CO2 loading Lr at the outlet of the absorber. To characterize the transient response, dead time θ, settling time ts, total stabilization time tt, and relative change (RC) were calculated:
- Dead time θ: it is the time that takes before a process variable starts to change from the initial steady-state conditions as a response to the disturbance or input.
- Settling time: The 10% settling time ts is the time taken from when the process variable begins to respond to the input change (dead time) until it remains within an error band described by 10% of the change in the process variable ∆y and the final steady-state value of the process variable y∞, i.e.: −0.1 ∆y+ y∞ < y∞ < 0.1 ∆y + y∞.
- Total stabilization time: the sum of the dead time θ and the settling time ts is the resulting total stabilization time tt.
- Relative change RC: Change in the observed process variable from initial steady-state conditions y0 to the final steady-state conditions; refer to Equation (14).
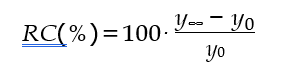
The detailed results of the process simulations are presented in Tables A1–A3 in Appendix A. Figure 8 shows the total stabilization times for the selected process variables at the three operating points, for step changes in solvent flow rate and reboiler duty. The responses for step changes in flue gas flow rate are not presented, since it is shown in Table A1 that the relative change RC in the output process variables is very small or negligible (RC ranges from −0.81% to 0.21%). This can be explained by the highly diluted nature of the CO2 in the flue gas (ca. 3.5 vol%). The results show the non-linear behavior of the plant, with different transient responses to step change set-point increase and decrease in key plant inputs, and at different loads of the plant.
Figure 8a shows the total stabilization time for lean CO2 loading Ll at the inlet of the absorber, which ranges from 25 to 45 min in all cases. The results show that the required time for total stabilization increases when the plant is operated at lower loads. As shown in Appendix A (Tables A1 and A2), a general trend was that the dead time θ in the response of Ll to step changes in reboiler duty and rich solvent mass flow rate increases at part-load points. This could be explained by the fact that at lower loads the solvent mass flow rate is smaller (refer to Fsolv in Table 6), resulting in longer residence times of the solvent through each equipment hold-up, piping, and recycle loop, this is, larger circulation time. This can also explain why dead times are generally larger when decreasing solvent flow rate than when increasing it; refer to Table A2 in Appendix A.
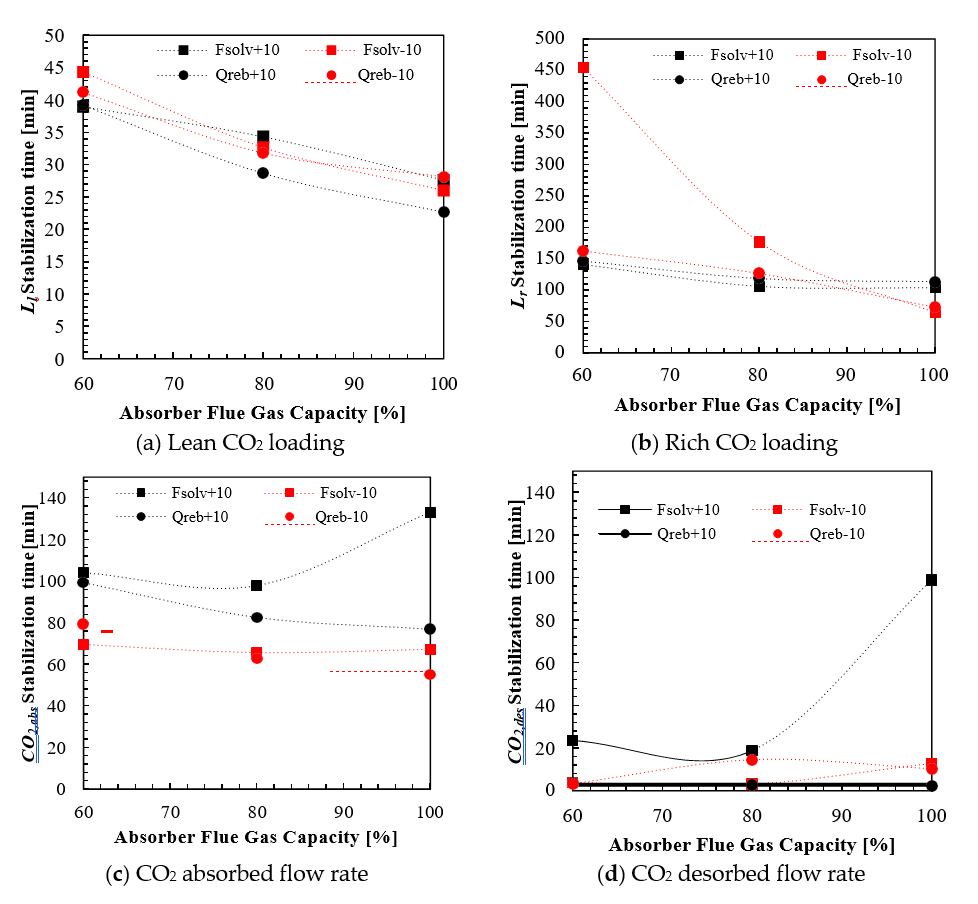
Figure 8. Simulation results. Total stabilization times ts for open-loop ± 10% step changes in solvent flow rate and reboiler duty for the process variables (a) Lean CO2 loading Ll; (b) rich CO2 loading Lr; (c) CO2 absorbed CO2 ,abs and (d) CO2 desorbed CO2 ,abs. Stabilization times are calculated for the response when the plant is operated at three different operating points in terms of flue gas mass flow rate, 100%, 80% and 60% of nominal capacity; refer to Tables 5 and 6.
Figure 8b shows the total stabilization times for rich CO2 loading Lr at the outlet of the absorber sump. In this case, the stabilization times range from 60 to 450 min. It should be mentioned that the relative change RC in rich CO2 loading is also small or negligible for the disturbances studied (see Appendix A), due to the fact that the solvent is operated close to its maximum loading capacity of 0.51 mol/mol CO2 loading. The total stabilization times for CO2 absorbed CO2,absresponse to disturbances in rich solvent mass flow rate Fsolv and reboiler duty Qreb are shown in Figure 8c. Total stabilization times range from 55 to 135 min. When the rich solvent mass flow rate is increased by 10%, this results in an increase in CO2 absorbed with a relative change RC of 0.35% to 4.18% (refer to Table A2), due to the increased
L/G ratio in the absorber column. However, since the reboiler duty is kept constant, the lean loading will increase (see RC values of Ll in Table A2). Due to the residence time in the hot solvent piping, lean/rich heat exchanger and lean amine cooler of the recycle loop, it takes time for the solvent to be distributed towards the inlet of the absorber. A dead time in CO2 lean loading Ll at the inlet of the absorber of 11 to 22 min is observed (see Table A2). This results in it taking a long time for the CO2,absto stabilize. When the rich solvent mass flow rate is decreased by 10%, it is observed that the CO2 absorbed CO2,absdecreases (relative change RC between −3.14% and −5.59% in Table A2). This is a result of the combination of the reduction in L/G ratio and the decrease in lean loading Ll is observed at the absorber inlet with a dead time of 13 to 23 min (due to circulation time of the solvent in the recycle loop), and the total stabilization time for CO2,abs requiring time for stabilization (stabilization time of 65 to 69 min). When reboiler duty Qreb is increased by 10%, the lean loading Ll is decreased significantly (RC ranging from 6.75 to 8.59%), which results in increase of CO2,abs (relative change RC of 4.0% to 6.07%). The change in lean loading Ll is observed at the absorber inlet with a dead time of 13 to 23 min (due to circulation time of the solvent in the recycle loop), and the total stabilization time for CO2,abs for increase in reboiler duty ranges from 76 to 99 min. When reboiler duty Qreb is decreased by 10%, the solvent lean loading increases (RC of 6.63% to 8.46%), resulting in less CO2 being absorbed. Relatively slower response in CO2,absto disturbances in solvent flow rate and reboiler duty were found when the PCC was operated at lower loads (55 to 99 min). An exception is found for the case when the solvent flow rate is increased at 100% mass flow rate operating conditions of the plant.
Figure 8d shows the stabilization times for CO2 desorbed CO2,abs. For disturbances in rich solvent flow rate and reboiler duty, the desorbed CO2 stabilizes slightly faster at lower loads (ranging from 2 to 100 min). In general, it was found that the desorption rate stabilized faster than the absorption rate CO2,abs for the disturbances in solvent flow rate and reboiler duty applied to the process. When solvent flow rate is decreased, this results in smaller L/G ratio in the absorber column and less CO2 being desorbed in the stripper column. Since the rich CO2 loading does not change significantly (RC in Lr from 0 to 0.08%), the CO2 desorbed CO2,desstabilizes faster than the CO2 absorbed (circulation time through the recycle loop is not affecting the stabilization of CO2,abs). When the reboiler duty Qreb is increased by 10%, the relative change in CO2 desorbed is large (4 to 6.07% in Table A3), and with fast total stabilization time (2 to 3 min in Table A3). A change in reboiler duty results in a fast response in the produced stripping vapors, which also results in a fast response in CO2 product flow rate (CO2 desorbed). The longest stabilization time for CO2 desorbed is found when the solvent flow rate is increased at 100% operating conditions. It is notable that there is a big difference in total stabilization times for solvent flow rate increase at different loads of the plant.
5.2 Decentralized Control Structures
In this section, four control structures for the TCM DA amine plant were tested via dynamic process model simulations. The scenario considers realistic load changes on the power plant, by changing flue gas flow rate feed to the absorber column. From a control analysis perspective, flue gas flow rate change can be considered as a disturbance applied to the PCC process. A load change event would result in a significant change in flue gas flow rate, at a ramp rate given by GT operation and controls. Fast ramp rates are the goal of power plant operators, since a fast power plant can respond to the variability in costs in a day-ahead power market [7,64]. For a NGCC power plant, a fast ramp rate is considered to be around 10%/min GT load [4,65]. Two tests were considered and simulated:
- Test 1: Ramping down flue gas flow rate from 100 to 70% in 3 min. The transient event starts at
t0 = 0 min, and sufficient simulation time is allowed for the plant to reach the new steady-state.
- Test 2: Flue gas flow rate is ramped up from 70 to 100% in 3 min. The transient event starts at
t0 = 0 min, and sufficient simulation time is allowed for the plant to reach the new steady-state.
The supervisory or advanced control layer of the TCM DA amine plant has three main degrees of freedom, consisting of set point of flue gas volumetric flow rate Fgas, set point of rich pump solvent flow rate Fsolv, and steam flow rate to feed the reboiler duty Qreb; refer to FT1, FT5 and FT4, respectively in Figure 1. Under normal and stable operation of the pilot plant at TCM DA, such degrees of freedom are changed manually by the operators to bring the plant to different operating conditions. If flue gas flow rate is considered to be a disturbance, there are two degrees of freedom left for operation. Note that here we do not consider the degrees of freedom available to the operators in the stabilizing or regulatory control layer, or for other auxiliary operations of the plant, or start-up procedures. Several studies in the literature suggest that keeping the capture ratio Cap and a temperature in the stripper column constant can lead to efficient operation of the process for varying loads of the PCC absorber-desorber process [13]. In this analysis, four control structures were tested, as presented in Table 7. All the feedback control loops are PI controllers, and were tuned with the simple internal model control (SIMC) tuning rules [66].
- Control structure A uses Fsolv to control capture ratio at the top of the absorber Cap defined by Equation (13) to the set point of 0.85, and reboiler duty Qreb to control the solvent temperature at the stripper bottom Tstr to the set point of 120.9 ◦C. This control structure has been previously proposed in the literature in different studies including [14,16], where it shows a fast response and the capability to reject disturbances.
- Control structure B uses Fsolv to control the solvent temperature at the stripper bottom Tstr to the set point of 120.9 ◦C, and reboiler duty Qreb to control capture ratio at the top of the absorber Cap to the set point of 0.85. Note that changes in reboiler duty result in a big change in solvent lean CO2 loading (large relative change RC; see Appendix A). A similar version was suggested by Panahi and Skogestad [14], where it was found that this control structure showed similar dynamic behavior, in response to disturbances in flue gas flow rate, compared with a model predictive control scheme (MPC).
- Control structure C utilizes solvent flow rate Fsolv to control the mass-based L/G ratio in the absorber column at the same value as that in the close-to-design-point operating conditions. This control structure has been studied previously in [12,15]. This control loop is implemented via ratio control. In addition, reboiler duty is manipulated to control Tstr to 120.9 ◦C. The control structure leads to different final steady-state operating conditions when ramping down the plant load than the other three alternatives.
- Control structure D is a modification of control structure A. In this control structure, the solvent flow rate set point is changed via a feed forward (FF) action to control the capture ratio Cap at 0.85; in addition, the stripper bottom temperature is controlled by manipulating the reboiler duty. The feed forward controller is implemented by a set-point ramp change in the solvent flow rate with the same total duration as the flue gas flow rate ramp change, to the final value that gives a Cap of 0.85 under final steady-state conditions.
Figure 9 shows the simulated time input trajectories during the test with flue gas flow rate reduction. The manipulated variables Fsolv and Qreb are shown for the different control structures evaluated. Figure 10 shows the output trajectories of CO2 capture ratio Cap, desorption ratio Des, CO2 absorbed and CO2 desorbed for the transient tests of flue gas flow rate reduction. Figure 11 shows the trajectories of lean loading Ll and stripper bottom solvent temperature Tstr for flue gas flow rate reduction. In addition, Figure 12 shows the simulated time input trajectories during the test with flue gas flow rate increase. Figure 13 shows the output trajectories of CO2 capture ratio Cap, desorption ratio Des, CO2 absorbed and CO2 desorbed for the transient tests of flue gas flow rate increase, and Figure 14 shows the trajectories of lean loading Ll and stripper bottom solvent temperature Tstr for flue gas flow rate increase. In order to compare the different control structure performances during transient load change, the total stabilization times of the selected process variables are shown in Table 8. These will indicate how fast the plant achieves stabilization of the different floating (not controlled) process variables when moving from one operating condition to the next one. In addition, three transient performance indicators have been considered and presented in Table 9. Note that, for this analysis auxiliary consumptions of the plant are not considered.
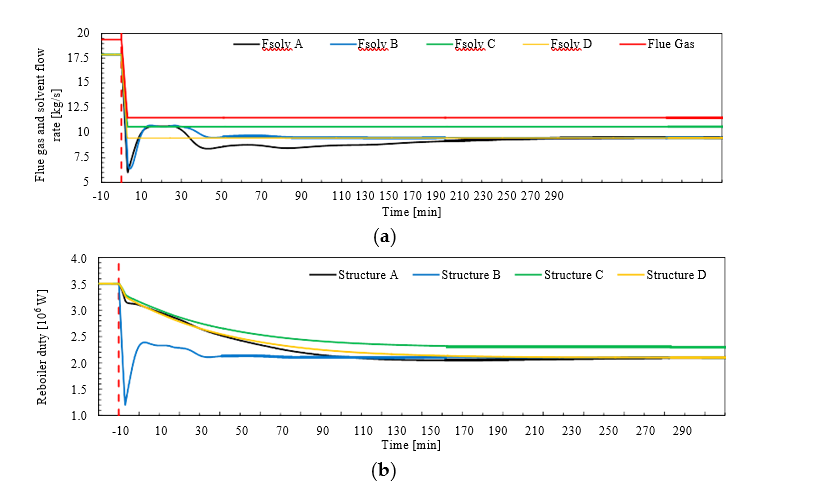
Figure 9. Inputs to the pilot plant during simulations for load change ramp-down (Test 1) from 100 to 70% with a ramp rate of 10%/min reduction in flue gas flow rate, for control structures A, B, C and D. (a) Flue gas flow rate (kg/s), as a disturbance, and solvent flow rates (kg/s) of the rich pump as manipulated variables (MVs); (b) Reboiler duty (W) as MV. The red vertical dotted line shows when the transient event starts at t0.
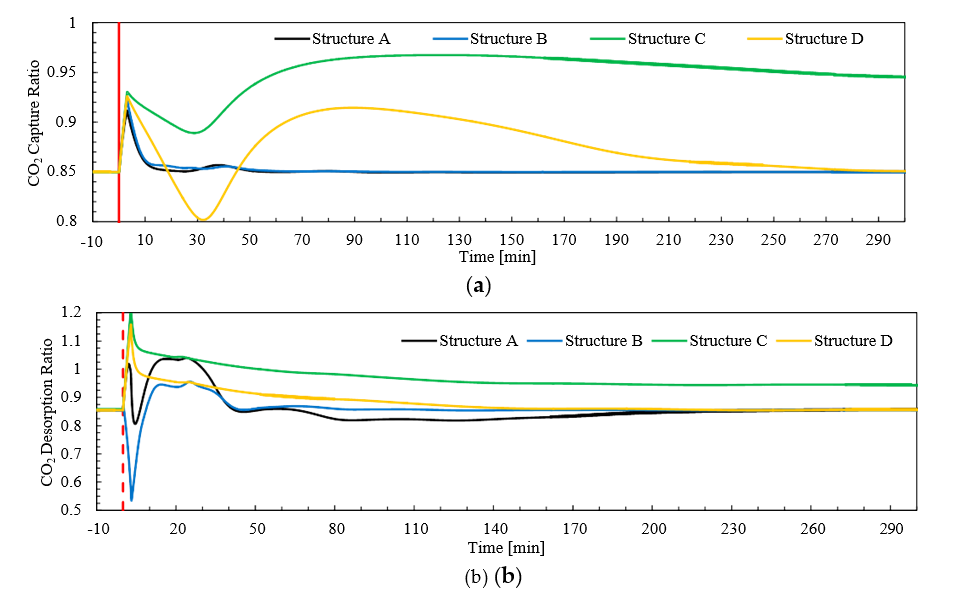
Figure 10. Cont.
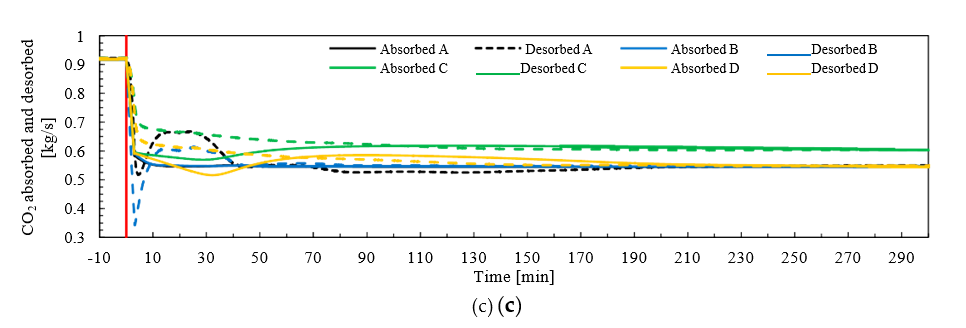
Figure 10. Outputs from pilot plant model during simulations for load change ramp-down (Test 1) from 100 to 70% with a ramp rate of 10%/min reduction in flue gas flow rate, for control structures A, B, C and D. (a) CO2 capture ratio Cap, as controlled variable (CV); (b) CO2 desorption ratio Des; (c) CO2 absorption and desorption rates (kg/s). The red vertical dotted line shows when the transient
event starts at t0.
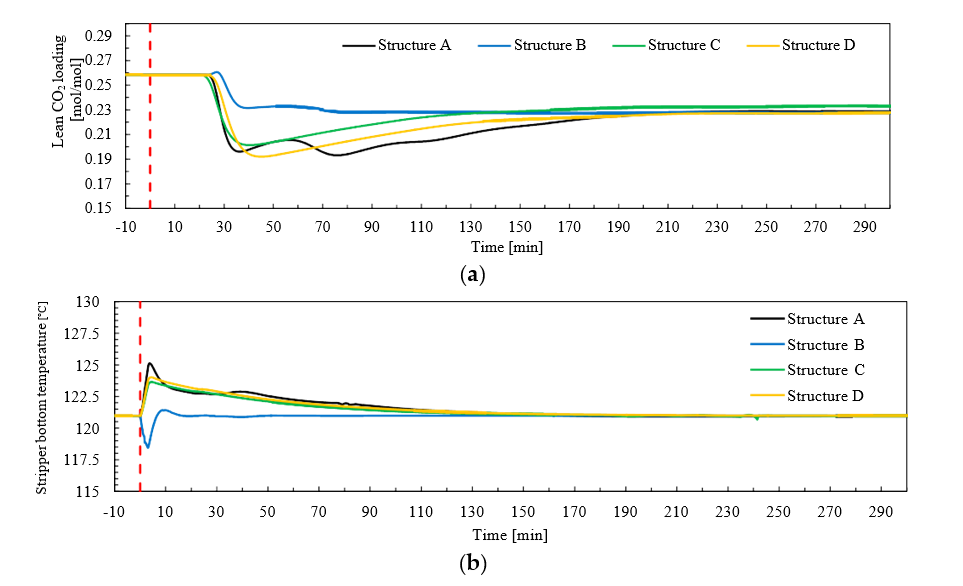
Figure 11. Outputs from pilot plant model during simulations for load change ramp-down (Test 1) from 100 to 70% with a ramp rate of 10%/min reduction in flue gas flow rate, for control structures A, B, C and D. (a) Lean CO2 loading at the inlet of the absorber; (b) Stripper bottom temperature as controlled variable (◦C). The red vertical dotted line shows when the transient event starts at t0.
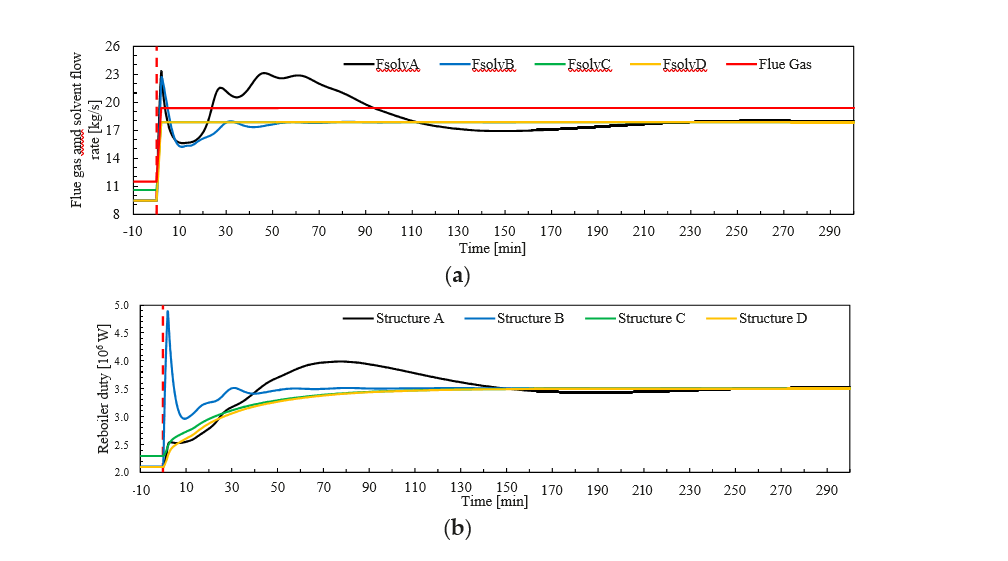
Figure 12. Inputs to the pilot plant during simulations for load change ramp-up (Test 2) from 70 to 100% with a ramp rate of 10%/min increase in flue gas flow rate, for control structures A, B, C and D. (a) Flue gas flow rate (kg/s), as a disturbance, and solvent flow rates (kg/s) of the rich pump as manipulated variables (MVs); (b) Reboiler duty (W) as MV. The red vertical dotted line shows when the transient event starts at t0.
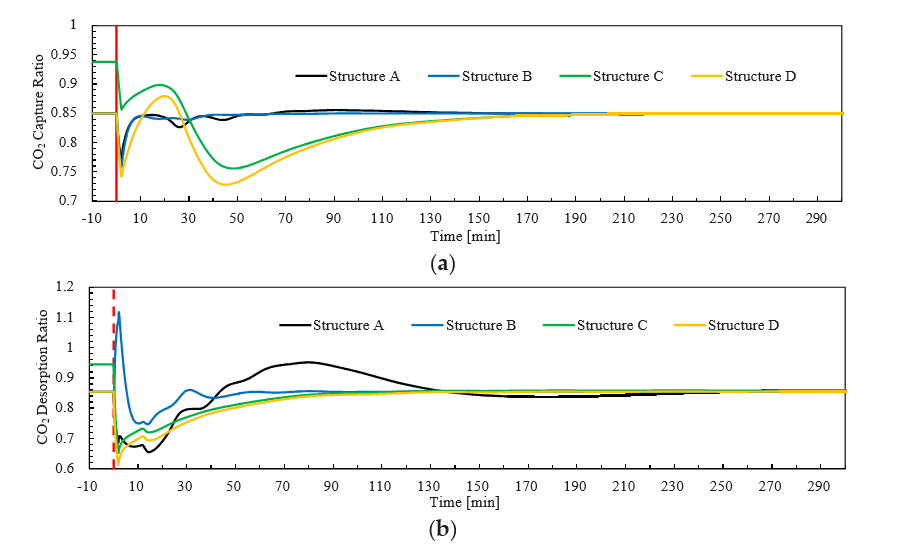
Figure 13. Cont.
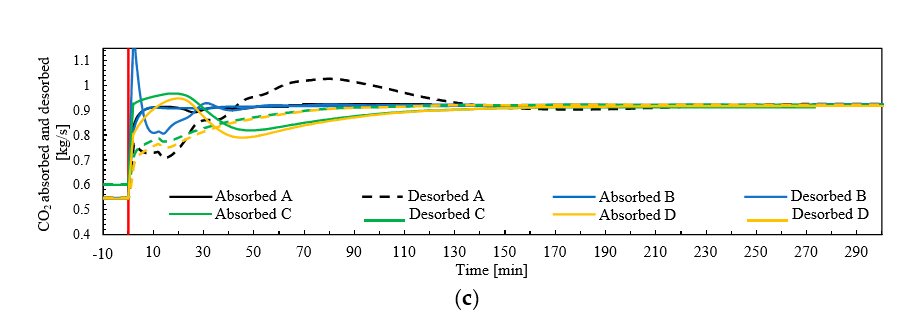
Figure 13. Outputs from pilot plant model during simulations for load change ramp-up (Test 2) from 70 to 100% with a ramp rate of 10%/min increase in flue gas flow rate, for control structures A, B, C and D. (a) CO2 capture ratio Cap, as controlled variable (CV); (b) CO2 desorption ratio Des; (c) CO2 absorption and desorption rates (kg/s). The red vertical dotted line shows when the transient event starts at t0.
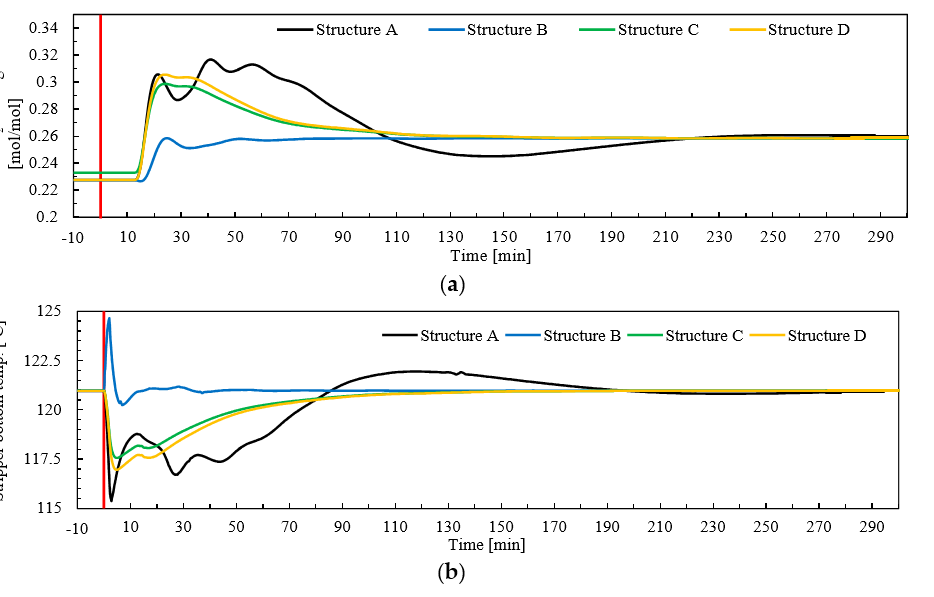
Figure 14. Outputs from pilot plant model during simulations for load change ramp-up (Test 2) from 70 to 100% with a ramp rate of 10%/min increase in flue gas flow rate, for control structures A, B, C and D. (a) Lean CO2 loading at the inlet of the absorber; (b) Stripper bottom temperature as controlled variable (◦C). The red vertical dotted line shows when the transient event starts at t0.
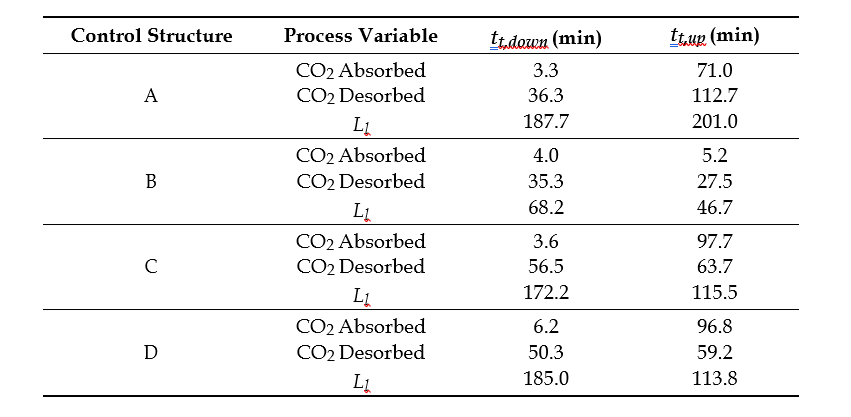
Table 8. Total stabilization times of the floating process variables for the different control structures, when ramping down the plant tt,down from 100% flue gas mass flow rate to 70%; and when ramping up the plant tt,up from 70% flue gas mass flow rate to 100%.
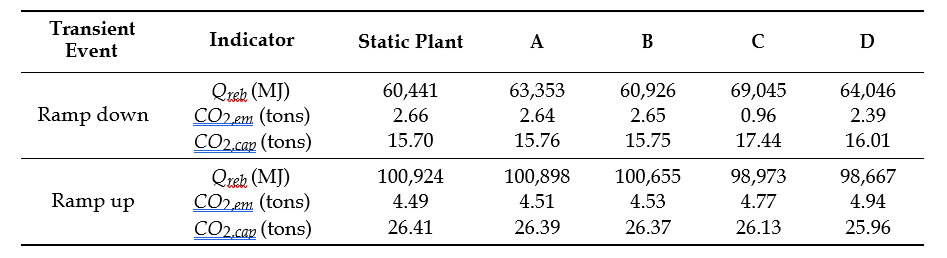
Table 9. Simulation results for accumulated reboiler energy consumption Qreb (MJ), accumulated CO2 emitted CO2 em and accumulated CO2 captured CO2 ,cap during the transient event (8 h) for the different control structures A,B, C and D (refer to Table 7), when ramping up and down the plant (between 100% and 70% of flue gas mass flow rate). Static plant refers to an ideal static plant that changes from the initial operating conditions to the final operating conditions instantaneously at time t = 0. An integration time of tf = 480 min was utilized to calculate the values for the ideal static plant
- Accumulated reboiler energy input Qreb (MJ): see Equation (15). This is calculated by integration of the Qreb trajectory under the transient event, from the initial time t0 = 0 min to the final time tf = 480 min (8 h). The final time was defined to ensure that the plant was already under steady-state conditions at the final operating point. This value Qreb represents the main energy consumption of the process during the transient event of load change. In addition, the consumption of an ideal static plant is included for comparison (see Table 9). The ideal static plant is assumed to change from initial to the final steady-state operating conditions instantaneously at time t0, and would operate until tf. The static plant value represents the minimum value when ramping down and a maximum value when ramping up.
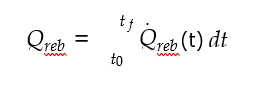
- Accumulated CO2 emitted CO2,em(tons): see Equation (16). This is calculated by integration of the m.CO trajectory under the transient event, from the initial time t0 = 0 min to the final time tf = 480 min; this represents the CO2 emitted at the absorber stack. The final time was defined to ensure that the plant was already under steady-state conditions at the final operating point. This measure represents the CO2 emitted during the transient event of load change. For comparison, the CO2 emitted by an ideal static plant is calculated (considered as the maximum value when ramping down and a minimum value when ramping up), shown in Table 9

- Accumulated CO2 captured CO2,cap (tons): see Equation (17). This is calculated by integration of the CO2 absorbed CO2,abstrajectory (Equation (12)) under the transient event, from the initial time t0 = 0 min to the final time tf = 480 min. The final time was defined to ensure that the plant was already under steady-state conditions at the final operating point. This measure represents the CO2 captured during the transient event of load change. For comparison, the CO2 captured by an ideal static plant is calculated (considered as the minimum value when ramping down and a maximum value when ramping up), shown in Table 9.

Figure 10 shows that the CO2 capture ratio Cap had similar trajectories for control structures A and B during Test 1 (flue gas ramp-down), and that Cap reached stabilization conditions faster (20–50 min) than control structures C and D (around 270 min). Cap had also larger excursions from the set point than when control structures A and B are utilized. The same trends are found for Test 2 with flue gas flow rate ramp-up (Figure 13). When ramping up, control structures C and D stabilize faster (around 160 min) than when ramping down. This showed that the utilization of close-loop feedback control (structures A and B) allows shorter stabilization times to be reached for the controlled variable CO2 capture ratio Cap. The desorption ratio Des trajectories in Figure 10 show that the plant requires the shortest stabilization time for this process variable when employing control structure B (around 60 min), followed by control structure A and C (around 200 min). This can be explained by the fact that for a change in reboiler duty the response of CO2 desorbed has a fast total stabilization time and a large static relative change RC (where RC ranges from 4 to 6.29% and total stabilization time range from 2.2 to 3.5 min for a +10% step in reboiler duty); refer to Table A3. When it comes to the stabilization time required for Des for Test 1, structures C and D presented a poorer performance as the trajectories for Cap and Des deviate from the set point significantly. For control structure A, Des showed slow performance for Test 2 (around 210 min total stabilization time) with significant oscillations around set point; refer to Figure 13.
When ramping down the plant, CO2 absorbed and CO2 desorbed require similar stabilization times for control structures A and B (around 3 min for CO2,abs and 36 min for CO2,abs), while the control structures C and D require longer stabilization times for CO2 desorbed (around 50 to 57 min); refer to Table 8. The trajectory of CO2 lean loading again shows shorter stabilization time for control structure B. This can be explained by the large static relative change RC of the response of CO2 lean loading to changes in reboiler duty (where RC ranges from −6.29% to −4.97% and total stabilization time range from 22.7 to 39.2 min for a +10% step in reboiler duty); refer to Table A3. This contributes to the tight control of CO2 capture ratio Cap achieved by control structure B, since the CO2 lean loading Ll is a key process variable that connects the operation of the stripper and the absorber columns via the recycle loop. In addition, control structure B shows the shortest stabilization times and smaller excursions of the stripper bottom temperature Tstr (around 15 to 30 min), in Figures 11 and 14.
When the plant load is ramped up from 70 to 100% (Test 2), the control structure B in general showed a faster dynamic performance with significantly shorter stabilization times required for the floating process variables considered (5.2 min for CO2,abs, 27.5 min for CO2,absand 46.7 min for Ll), see Table 9; followed by C, D and A. Note that control structure B presented a faster dynamic performance towards stabilization while ramping up (Ll stabilizes in 46.7 min) than when ramping down the process (Ll stabilizes in 68.2 min). Control structures A, C and D required shorter stabilization times for CO2 absorption and CO2 desorption when ramping down the process load, while CO2 lean loading stabilized faster when ramping up the plant load; refer to the stabilization time values in Table 9. When the plant is operated under control structure C, the optimum solvent flow rate Fsolv and lean loading Ll are not reached at the 70% absorber capacity steady-state operating conditions; refer to time >250 min in Figures 9a and 11a, and time <0 min in Figures 12a and 14a. This leads to a higher Cap than specified (refer time t > 290 min in Figure 10a and time t < 0 min in Figure 13a), and therefore higher reboiler duty (time t > 290 min in Figure 9b and time t < 0 min in Figure 12b), even though the stripper bottom temperature Tstr criterion is satisfied.
During the ramp-down transient event of the plant (i.e., period of 8 h from the time change was implemented), the least energy-intensive performance measured by Qreb in Table 9 was observed for control structure B. In addition, this structure shows the largest CO2 emissions during the transient event, albeit still lower than the ideal static plant. The fast stabilization time of the plant process variables achieved by control structure B provides a transient performance that is the closest to the ideal static plant. Control structures C and D showed the largest CO2 captured during the transient event. However, when ramping down the plant load, this means that the plant is emitting less CO2 during the transient event with control structures A, B, C and D than that established by the operational objective and represented by the ideal static plant case. Consequently, when ramping down the plant load, CO2 emissions will always be lower than those of the equivalent ideal static plant. In addition, the plant is capturing more CO2 than the ideal static plant. Figure 10a shows how there are periods of time in which the capture ratio Cap is above the target of 0.85, leading to more CO2 being captured than the ideal static plant during the transient event. Control structures A and B showed the largest CO2 emitted when compared with the ideal static case. Despite control structure A presenting a similar amount of CO2 emitted during the transient event, it requires a larger amount of energy input during this period than control structure B. Therefore, control structure B shows the best performance in terms of energy consumption and CO2 emissions during the transient load change event of ramping down the PCC plant load. When ramping up the plant load the most energy-intensive control structure is control structure B. However CO2 emissions are the lowest, being closer to the minimum established by the static plant. This means that, when ramping up the plant load, CO2 emissions will always be higher than those of the equivalent ideal static plant. While control structure D is the least energy-intensive process during the transient event of load change increase, it is the control structure with the largest CO2 emissions during this transient event.
6. Conclusions
The pilot plant data obtained in this work from an MEA campaign at TCM DA amine plant includes ten steady-state operating data sets. The data sets consist of a wide range of steady-state operating conditions of the chemical absorption process in terms of L/G ratio in the absorber column, different absorber packing heights, CO2 capture ratios, reboiler duty and flue gas flow rate fed to the absorber. The data is considered reliable and valid and can be used for process model validation purposes. In addition, the three transient data sets presented in this work represent transient operation of the pilot plant driven by set-point changes in flue gas flow rate, solvent circulation flow rate and reboiler duty. The transient data sets are considered reliable and suitable for dynamic process model validation purposes, provided that input trajectories can be applied to the dynamic process model.
The validation of the dynamic process model with the steady-state and transient data shows that the process model has a good capability of predicting the steady-state and transient behavior of the plant for a wide range of operating conditions. The validation included in this work proves the capacities of dynamic process modeling applied to large-scale experimental data. The model is considered suitable for studies including transient performance analysis and control structure evaluation studies at the plant scale. In addition, it provides confidence towards using the dynamic process model for analysis of larger-scale PCC plants.
The case study carried out in this work via dynamic process simulations with the validated model shows that, generally, the plant responds more slowly at lower operating loads (the load being defined by the flow rate fed to the absorber). A general trend is observed, in which it takes a longer time to stabilize the main process variables of the pilot plant under open-loop step changes in the main inputs of the process, namely solvent flow rate, flue gas flow rate and reboiler duty. From the process simulations, it is found that, in general, the desorption rate stabilizes faster than the absorption rate for set-point step changes in solvent flow rate and reboiler duty. In addition, ±10% step changes in flue gas flow rate around a given operating point do not cause a large relative change in the main process variables of the process (RC ranges from −0.81% to 0.21%).
The evaluation of the decentralized control structures shows that by adding closed-loop controllers on the two main degrees of freedom of the plant—solvent flow rate and reboiler duty—to control two other process variables, including CO2 capture ratio and stripper bottom solvent temperature, the plant can be stabilized faster and more efficiently under varying loads. The control structure that showed the best performance was control structure B, in which the reboiler duty is manipulated to control CO2 capture ratio at the inlet of the absorber and the rich solvent flow rate to control the stripper bottom solvent temperature. It was observed that control structure B provides the fastest stabilization times for the main process variables under scenarios when the plant load is ramped down and up, with ramp rates typically found in NGCC power plants with fast-cycling capabilities. When reducing the PCC process load, this control structure is the least energy-intensive of those evaluated in this work. When increasing the plant load, this control structure is the one with the lowest accumulated CO2 emissions imposed by the process inertia during load-change transient operation.
Acknowledgments
The authors acknowledge the Department of Energy and Process Engineering at NTNU-Norwegian University of Science and Technology and TCM DA owners Gassnova, Shell, Statoil and Sasol, for funding this project. The funds for covering the costs to publish in open access were provided by the Norwegian University of Science and Technology—NTNU.
Author Contributions
Rubén M. Montañés contributed to the selection of experimental data; processed the experimental data; developed the models; carried out the calibration, validation and simulation of the dynamic process models; defined and carried out the case studies; analyzed the results; and wrote the manuscript. Nina E. Flø contributed to the experimental data selection; contributed to the critical analysis of the results; and reviewed the manuscript. Lars O. Nord contributed to the critical analysis of the results; reviewed the manuscript; and supervised the work.
Conflicts of Interest
The authors declare no conflict of interest.
Abbreviations and Symbols
Aif Contact area
AP Absolute percentage error
Cap CO2 capture ratio
CHP Combined heat and power
CCS Carbon capture and storage
CO2 Carbon dioxide
CO2,em CO2 emitted (kg/s)
ci Molar concentration
Cef Pre-multiplying coefficient
DCC Direct contact cooler
Des Desorption ratio
DCO2 Diffusivity of CO2 in aqueous monoethanolamine
E Enhancement factor
F Mass flow rate (kg/s)
FB Feedback
FC Flow controller
FF Feed-forward
FT Flow transmitter
GA Gas analyzer
GC Gas chromatograph
GT Gas turbine
Hei Henry’s constant
H2O Water
HX Heat exchanger
ki Mass transfer coefficient
Ki Equilibrium constant
LC Level controller
Ll Lean CO2 loading
Lr Rich CO2 loading
L/G Mass-based liquid to gas ratio (kg/kg)
LT Level transmitter
MAP Mean absolute percentage error
MEA Monoethanolamine
MPC Model predictive control
N2 Nitrogen
NCCC National carbon capture center
NGCC Natural gas combined cycle
O2 Oxygen
p Pressure (Pa)
PC Pressure controller
PCC Post-combustion CO2 capture
PT Pressure transmitter
PZ Piperazine
Qreb Reboiler duty (W)
Qreb Reboiler energy input (J)
RC Relative change
SA Solvent analyzer
SIMC Simplified internal model control
SRD Specific reboiler duty (kJ/kgCO2)
T Temperature (K)
TC Temperature controller
TCM DA Technology Centre Mongstad
ts Settling time
tt Total stabilization time
TT Temperature transmitter
X Mass fraction
xp Value measured at pilot plant
xm Value simulated model
y∞ Steady-state final value
θ Dead time
γi Activity coefficient
∆Hr Heat of reaction
∆y Change in process variable
ε-NTU Effectiveness number of thermal units
Appendix A
Tables A1–A3 show the simulation results in terms of the dead time θ, 10% settling time ts, total stabilization time tt and relative change RC %, for the open-loop response to step-changes in the main inputs to the plant. The step changes are applied to the plant when it is operated at three different steady-state operating conditions defined by three different mass flow rate capacities of the absorber column. The inputs are:
- Flue gas mass flow rate ±10% step-change.
- Solvent mass flow rate ±10% step-change.
- Reboiler duty ±10% step-change.
The output process variables studied are:
- CO2 lean loading Ll (mol/mol).
- CO2 rich loading Lr (mol/mol).
- CO2 absorbed CO2,abs(kg/s).
- CO2 desorbed CO2,abs(kg/s).
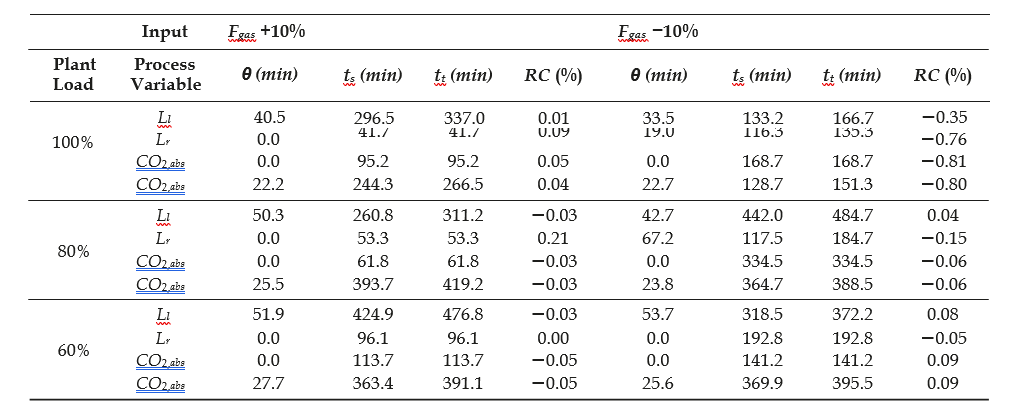
Table A1. Open-loop response to ±10% step-changes in flue gas mass flow rate for three different operating points of the pilot plant. Responses in CO2 lean loading Ll, CO2 rich loading Lr, CO2 absorbed, and CO2 desorbed.
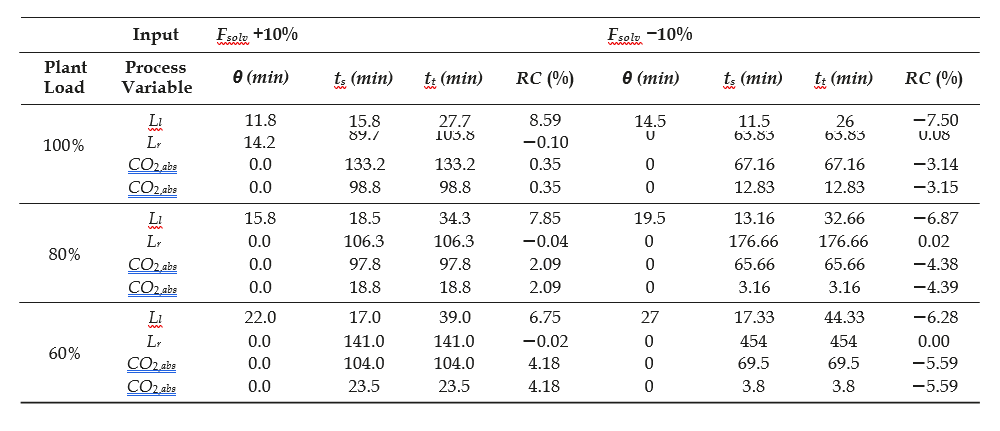
Table A2. Open-loop response to ±10% step-changes in solvent mass flow rate for three different operating points of the pilot plant. Responses in CO2 lean loading Ll, CO2 rich loading Lr, CO2 absorbed, and CO2 desorbed.
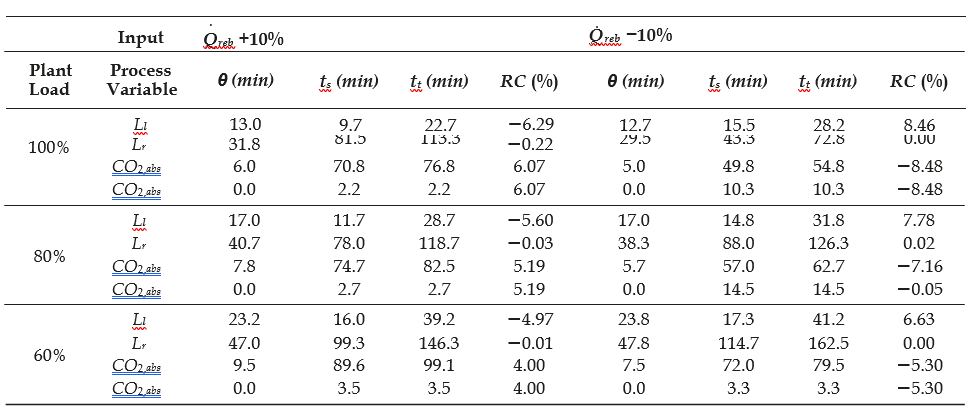
Table A3. Open-loop response to ±10% step-changes in reboiler duty for three different operating points of the pilot plant. Responses in CO2 lean loading Ll, CO2 rich loading Lr, CO2 absorbed, and CO2 desorbed
References
- The International Energy Agency (IEA). CO2 Capture and Storage: A Key Carbon Abatement Option; International Energy Agency: Paris, France, 2008.
- Singh, A.; Stéphenne, K. Shell Cansolv CO2 capture technology: Achievement from first commercial plant. Energy Procedia 2014, 63, 1678–1685. [CrossRef]
- Laboratory, N.E.T. Petra Nova Parish Holdings. W.A. Parish Post-Combustion CO2 Capture and Sequestration Project. Available online: https://www.netl.doe.gov/research/coal/project-information/ fe0003311-ppp (accessed on 28 September 2017).
- Hentschel, J.; Babic´, U.A.; Spliethoff, H. A parametric approach for the valuation of power plant flexibility options. Energy Rep. 2016, 2, 40–47. [CrossRef]
- Mac Dowell, N.; Staffell, I. The role of flexible CCS in the UK’s future energy system. Int. J. Greenh. Gas Control 2016, 48, 327–344. [CrossRef]
- Gaspar, J.; Jorgensen, J.B.; Fosbol, P.L. Control of a post-combustion CO2 capture plant during process start-up and load variations. IFAC-PapersOnLine 2015, 48, 580–585. [CrossRef]
- Montañés, R.M.; Korpås, M.; Nord, L.O.; Jaehnert, S. Identifying operational requirements for flexible CCS power plant in future energy systems. Energy Procedia 2016, 86, 22–31. [CrossRef]
- Johnsson, F.; Odenberger, M.; Göransson, L. Challenges to integrate CCS into low carbon electricity markets. Energy Procedia 2014, 63, 7485–7493. [CrossRef]
- Boot-Handford, M.E.; Abanades, J.C.; Anthony, E.J.; Blunt, M.J.; Brandani, S.; Mac Dowell, N.; Fernandez, J.R.; Ferrari, M.-C.; Gross, R.; Hallett, J.P.; et al. Carbon capture and storage update. Energy Environ. Sci. 2014, 7, 130–189. [CrossRef]
- Flø, N.E.; Kvamsdal, H.M.; Hillestad, M.; Mejdell, T. Dominating dynamics of the post-combustion CO2 absorption process. Comput. Chem. Eng. 2016, 86, 171–183. [CrossRef]
- Karimi, M.; Hillestad, M.; Svendsen, H.F. Investigation of the dynamic behavior of different stripper configurations for post-combustion CO2 capture. Int. J. Greenh. Gas Control 2012, 7, 230–239. [CrossRef]
- Garđarsdóttir, S.Ó.; Montañés, R.M.; Normann, F.; Nord, L.O.; Johnsson, F. Effects of CO2-absorption control strategies on the dynamic performance of a supercritical pulverized-coal-fired power plant. Ind. Eng. Chem. Res. 2017, 56, 4415–4430. [CrossRef]
- Panahi, M.; Skogestad, S. Economically efficient operation of CO2 capturing process part i: Self-optimizing procedure for selecting the best controlled variables. Chem. Eng. Process. Process Intensif. 2011, 50, 247–253. [CrossRef]
- Panahi, M.; Skogestad, S. Economically efficient operation of CO2 capturing process. Part II. Design of control layer. Chem. Eng. Process. Process Intensif. 2012, 52, 112–124. [CrossRef] Energies 2017, 10,
- Montañés, R.M.; GarÐarsdóttir, S.Ó.; Normann, F.; Johnsson, F.; Nord, L.O. Demonstrating load-change transient performance of a commercial-scale natural gas combined cycle power plant with post-combustion CO2 capture. Int. J. Greenh. Gas Control 2017, 63, 158–174. [CrossRef]
- Nittaya, T.; Douglas, P.L.; Croiset, E.; Ricardez-Sandoval, L.A. Dynamic modelling and control of MEA absorption processes for CO2 capture from power plants. Fuel 2014, 116, 672–691. [CrossRef]
- Zhang, Q.; Turton, R.; Bhattacharyya, D. Development of model and model-predictive control of an MEA-based postcombustion CO2 capture process. Ind. Eng. Chem. Res. 2016, 55, 1292–1308. [CrossRef]
- International Energy Agency Greenhouse Gas R&D Programme (IEAGHG). Evaluation of Process Control Strategies for Normal, Flexible, and Upset Operation Conditions of CO2 Post Combustion Capture Processes; July 2016; IEAGHG: Cheltenham, UK, September 2016.
- Walters, M.S.; Edgar, T.F.; Rochelle, G.T. Regulatory control of amine scrubbing for CO2 capture from power plants. Ind. Eng. Chem. Res. 2016, 55, 4646–4657. [CrossRef]
- Gaspar, J.; Ricardez-Sandoval, L.; Jørgensen, J.B.; Fosbøl, P.L. Controllability and flexibility analysis of CO2 post-combustion capture using piperazine and MEA. Int. J. Greenh. Gas Control 2016, 51, 276–289. [CrossRef]
- Mac Dowell, N.; Shah, N. The multi-period optimisation of an amine-based CO2 capture process integrated with a super-critical coal-fired power station for flexible operation. Comput. Chem. Eng. 2015, 74, 169–183. [CrossRef]
- Flø, N.E.; Kvamsdal, H.M.; Hillestad, M. Dynamic simulation of post-combustion CO2 capture for flexible operation of the brindisi pilot plant. Int. J. Greenh. Gas Control 2016, 48 Pt 2, 204–215. [CrossRef]
- Ceccarelli, N.; van Leeuwen, M.; Wolf, T.; van Leeuwen, P.; van der Vaart, R.; Maas, W.; Ramos, A. Flexibility of low-CO2 gas power plants: Integration of the CO2 capture unit with CCGT operation. Energy Procedia 2014, 63, 1703–1726. [CrossRef]
- Wellner, K.; Marx-Schubach, T.; Schmitz, G. Dynamic behavior of coal-fired power plants with postcombustion CO2 capture. Ind. Eng. Chem. Res. 2016, 55, 12038–12045. [CrossRef]
- Olaleye, A.K.; Oko, E.; Wang, M.; Kelsall, G. Dynamic modelling and analysis of supercritical coal-fired power plant integrated with post-combustion CO2 capture. In Clean Coal Technology and Sustainable Development, Proceedings of the 8th International Symposium on Coal Combustion, Beijing, China, 19-22 July 2015; Yue, G., Li, S., Eds.; Springer: Beijing, China, 2016; pp. 359–363.
- Mechleri, E.; Lawal, A.; Ramos, A.; Davison, J.; Dowell, N.M. Process control strategies for flexible operation of post-combustion CO2 capture plants. Int. J. Greenh. Gas Control 2017, 57, 14–25. [CrossRef]
- Mechleri, E.; Fennell, P.S.; Dowell, N.M. Optimisation and evaluation of flexible operation strategies for coal-and gas-CCS power stations with a multi-period design approach. Int. J. Greenh. Gas Control 2017, 59, 24–39. [CrossRef]
- Sanchez Fernandez, E.; Sanchez del Rio, M.; Chalmers, H.; Khakharia, P.; Goetheer, E.L.V.; Gibbins, J.; Lucquiaud, M. Operational flexibility options in power plants with integrated post-combustion capture. Int. J. Greenh. Gas Control 2016, 48, 275–289. [CrossRef]
- Dutta, R.; Nord, L.O.; Bolland, O. Selection and design of post-combustion CO2 capture process for 600 MW natural gas fueled thermal power plant based on operability. Energy 2017, 121, 643–656. [CrossRef]
- Kvamsdal, H.M.; Jakobsen, J.P.; Hoff, K.A. Dynamic modeling and simulation of a CO2 absorber column for post-combustion CO2 capture. Chem. Eng. Process. Process Intensif. 2009, 48, 135–144. [CrossRef]
- Gaspar, J.; Cormos, A.-M. Dynamic modeling and absorption capacity assessment of CO2 capture process. Int. J. Greenh. Gas Control 2012, 8, 45–55. [CrossRef]
- Harun, N.; Nittaya, T.; Douglas, P.L.; Croiset, E.; Ricardez-Sandoval, L.A. Dynamic simulation of MEA absorption process for CO2 capture from power plants. Int. J. Greenh. Gas Control 2012, 10, 295–309. [CrossRef]
- Jayarathna, S.A.; Lie, B.; Melaaen, M.C. Amine based CO2 capture plant: Dynamic modeling and simulations. Int. J. Greenh. Gas Control 2013, 14, 282–290. [CrossRef]
- He, Z.; Sahraei, M.H.; Ricardez-Sandoval, L.A. Flexible operation and simultaneous scheduling and control of a CO2 capture plant using model predictive control. Int. J. Greenh. Gas Control 2016, 48, 300–311. [CrossRef]
- Luu, M.T.; Abdul Manaf, N.; Abbas, A. Dynamic modelling and control strategies for flexible operation of amine-based post-combustion CO2 capture systems. Int. J. Greenh. Gas Control 2015, 39, 377–389. [CrossRef]
- Biliyok, C.; Lawal, A.; Wang, M.; Seibert, F. Dynamic modelling, validation and analysis of post-combustion chemical absorption CO2 capture plant. Int. J. Greenh. Gas Control 2012, 9, 428–445. [CrossRef] Energies
- Åkesson, J.; Laird, C.D.; Lavedan, G.; Prölß, K.; Tummescheit, H.; Velut, S.; Zhu, Y. Nonlinear model predictive control of a CO2 post-combustion absorption unit. Chem. Eng. Technol. 2012, 35, 445–454. [CrossRef]
- Bui, M.; Gunawan, I.; Verheyen, V.; Feron, P.; Meuleman, E.; Adeloju, S. Dynamic modelling and optimisation of flexible operation in post-combustion CO2 capture plants—A review. Comput. Chem. Eng. 2014, 61, 245–265. [CrossRef]
- Enaasen, N.; Zangrilli, L.; Mangiaracina, A.; Mejdell, T.; Kvamsdal, H.M.; Hillestad, M. Validation of a dynamic model of the brindisi pilot plant. Energy Procedia 2014, 63, 1040–1054. [CrossRef]
- Enaasen Flø, N.; Knuutila, H.; Kvamsdal, H.M.; Hillestad, M. Dynamic model validation of the post-combustion CO2 absorption process. Int. J. Greenh. Gas Control 2015, 41, 127–141. [CrossRef]
- Van De Haar, A.; Trapp, C.; Wellner, K.; De Kler, R.; Schmitz, G.; Colonna, P. Dynamics of postcombustion CO2 capture plants: Modeling, validation, and case study. Ind. Eng. Chem. Res. 2017, 56, 1810–1822. [CrossRef] [PubMed]
- Gaspar, J.; Gladis, A.; Jørgensen, J.B.; Thomsen, K.; von Solms, N.; Fosbøl, P.L. Dynamic operation and simulation of post-combustion CO2 capture. Energy Procedia 2016, 86, 205–214. [CrossRef]
- Dutta, R.; Nord, L.O.; Bolland, O. Prospects of using equilibrium-based column models in dynamic process simulation of post-combustion CO2 capture for coal-fired power plant. Fuel 2017, 202, 85–97. [CrossRef]
- Chinen, A.S.; Morgan, J.C.; Omell, B.P.; Bhattacharyya, D.; Miller, D.C. Dynamic data reconciliation and model validation of a MEA-based CO2 capture system using pilot plant data. In Proceedings of the 11th IFAC Symposium on Dynamics and Control of Process Systems, Including Biosystems, Trondheim, Norway, 6–8 June 2016.
- Abdul Manaf, N.; Cousins, A.; Feron, P.; Abbas, A. Dynamic modelling, identification and preliminary control analysis of an amine-based post-combustion CO2 capture pilot plant. J. Clean. Prod. 2016, 113, 635–653. [CrossRef]
- Faber, R.; Köpcke, M.; Biede, O.; Knudsen, J.N.; Andersen, J. Open-loop step responses for the MEA post-combustion capture process: Experimental results from the esbjerg pilot plant. Energy Procedia 2011, 4, 1427–1434. [CrossRef]
- Bui, M.; Gunawan, I.; Verheyen, V.; Feron, P.; Meuleman, E. Flexible operation of CSIRO’s post-combustion CO2 capture pilot plant at the AGL Loy Yang power station. Int. J. Greenh. Gas Control 2016, 48, 188–203. [CrossRef]
- Tait, P.; Buschle, B.; Ausner, I.; Valluri, P.; Wehrli, M.; Lucquiaud, M. A pilot-scale study of dynamic response scenarios for the flexible operation of post-combustion CO2 capture. Int. J. Greenh. Gas Control 2016, 48, 216–233. [CrossRef]
- De Koeijer, G.M.; Aasen, K.I.; Steinseth Hamborg, E. Scale-Up and Transient Operation of CO2 Capture Plants at CO2 Technology Centre Mongstad; Society of Petroleum Engineers: Abu Dhabi, UAE, 2014.
- Gjernes, E.; Pedersen, S.; Cents, T.; Watson, G.; Fostås, B.F.; Shah, M.I.; Lombardo, G.; Desvignes, C.; Flø, N.E.; Morken, A.K.; et al. Results from 30 wt % MEA performance testing at the CO2 Technology Centre Mongstad. Energy Procedia 2017, 114, 1146–1157. [CrossRef]
- Faramarzi, L.; Thimsen, D.; Hume, S.; Maxon, A.; Watson, G.; Pedersen, S.; Gjernes, E.; Fostås, B.F.; Lombardo, G.; Cents, T.; et al. Results from MEA testing at the CO2 Technology Centre Mongstad: Verification of baseline results in 2015. Energy Procedia 2017, 114, 1128–1145. [CrossRef]
- Thimsen, D.; Maxson, A.; Smith, V.; Cents, T.; Falk-Pedersen, O.; Gorset, O.; Hamborg, E.S. Results from MEA testing at the CO2 Technology Centre Mongstad. Part I: Post-combustion CO2 capture testing methodology. Energy Procedia 2014, 63, 5938–5958. [CrossRef]
- Modelica Association. Available online: https://www.Modelica.Org/ (accessed on 28 September 2017).
- Modelon. Post-Combustion Capture with Amine Solutions. Available online: http://www.Modelon.Com/ industries/energy-process/carbon-capture-and-sequestration/ (accessed on 28 September 2017).
- Dassault Systems, Dymola. Available online: http://www.3ds.Com/products-services/catia/products/ dymola (accessed on 28 September 2017).
- Prölß, K.; Tummescheit, H.; Velut, S.; Åkesson, J. Dynamic model of a post-combustion absorption unit for use in a non-linear model predictive control scheme. Energy Procedia 2011, 4, 2620–2627. [CrossRef]
- Garðarsdóttir, S.Ó.; Normann, F.; Andersson, K.; Prölß, K.; Emilsdóttir, S.; Johnsson, F. Post-combustion CO2 capture applied to a state-of-the-art coal-fired power plant—The influence of dynamic process conditions. Int. J. Greenh. Gas Control 2015, 33, 51–62. [CrossRef]
- Onda, K.; Takeuchi, H.; Okumoto, Y. Mass transfer coefficients between gas and liquid phases in packed columns. J. Chem. Eng. Jpn. 1968, 1, 56–62. [CrossRef]
- Versteeg, G.F.; Van Dijck, L.A.J.; Van Swaaij, W.P.M. On the kinetics between CO2 and alkanolamines both in aqueous and non-aqueous solutions. An overview. Chem. Eng. Commun. 1996, 144, 113–158. [CrossRef]
- Holst, J.V.; Versteeg, G.F.; Brilman, D.W.F.; Hogendoorn, J.A. Kinetic study of CO2 with various amino acid salts in aqueous solution. Chem. Eng. Sci. 2009, 64, 59–68. [CrossRef]
- Böttinger, W. Nmr-Spektroskopische Untersuchung der Reaktivabsorption von Kohlendioxid in Wässrigen Aminlösungen; VDI-Verlag: Düsseldorf, Germany, 2006.
- Montañés, R.M.; Flø, N.E.; Dutta, R.; Nord, L.O.; Bolland, O. Dynamic process model development and validation with transient plant data collected from an mea test campaign at the CO2 technology center mongstad. Energy Procedia 2017, 114, 1538–1550. [CrossRef]
- International Energy Agency Greenhouse Gas R&D Programme (IEAGHG). Operating Flexibility of Power Plants with CCS; IEAGHG: Cheltenham, UK, June 2012.
- Christopher, H.J.; James, K. How to Determine a Unit Ramp Rate (MW/min) for Lowest Total Production Cost. Available online: http://www.heatrate.com/docs/Value-of-Ramp-Rate-1987.pdf (accessed on 28 September 2017).
- Genrup, M.; Thern, M. Ny Gasturbinteknik 2012–2014: Gas Turbine Developments; Report 2012; ELFORSK: Stockholm, Sweden, 2013.
- Skogestad, S.; Grimholt, C. The SIMC method for smooth PID controller tuning. In Pid Control in the Third Millennium: Lessons Learned and New Approaches; Vilanova, R., Visioli, A., Eds.; Springer: London, UK, 2012; pp. 147–175.
© 2017 by the authors. Licensee MDPI, Basel, Switzerland. This article is an open access article distributed under the terms and conditions of the Creative Commons Attribution (CC BY) license (http://creativecommons.org/licenses/by/4.0/).
Dynamic process model development and validation with transient plant data collected from an MEA test campaign at the CO2 Technology Center Mongstad (2017)
Rubén M. Montañésa*, Nina E. Fløb, Rohan Duttaa, Lars O. Norda, Olav Bollanda
aDepartment of Energy and Process Engineering, NTNU – Norwegian University of Science and Technology, NO-7491 Trondheim, Norway bCO2 Technology Center Mongstad (TCM DA), 5954 Mongstad, Norway. *Corresponding author
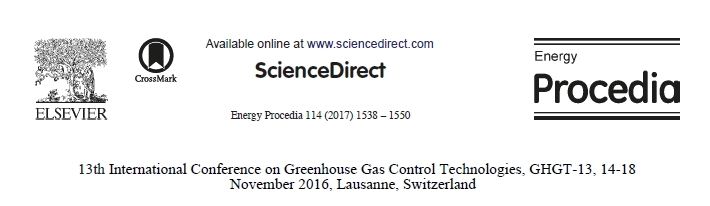
(http://creativecommons.org/licenses/by-nc-nd/4.0/).
Peer-review under responsibility of the organizing committee of GHGT-13.
doi: 10.1016/j.egypro.2017.03.1284
Abstract
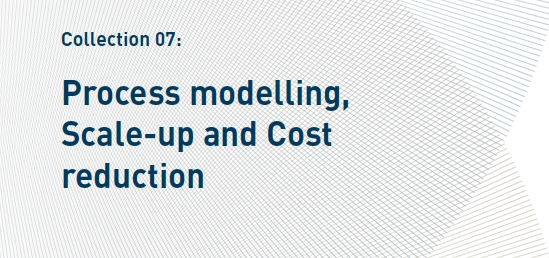
This work focuses on the development and validation of a dynamic process model of the post-combustion CO2 chemical absorption process with temperature swing absorption (TSA) using aqueous monoethanolamine (MEA) as solvent. A new set of steady-state and transient cases were generated during an MEA test campaign at the amine pilot plant at CO2 Technology Center Mongstad (TCM DA). Nine steady-state cases comprising a wide range of operating conditions of the plant and two transient tests consisting of flue gas volumetric flow rate step-changes were utilized for the purpose of dynamic process model validation of the overall pilot plant process model. It is concluded that the dynamic process model is capable of estimating the absorber and stripper columns temperature profiles with good accuracy after tuning of model parameters. An over-prediction of the model for lean and rich CO2 loadings has been reported, being mean percentage errors <1.5% for lean loading and <6.7% for rich loading. In addition, an under prediction of CO2 product flow rate has been observed (<5%). The process model is capable of predicting the variability of lean and rich loadings for the range of steady-state operating conditions. The main process dynamics of the pilot plant under flue gas volumetric flow rate set-point step changes is captured by the process model.
1. Introduction
Post-combustion CO2 capture (PCC) with amines is considered one of the more mature technologies that can contribute to reduce anthropogenic CO2 emissions to the atmosphere from fossil-fueled thermal power plants. It is considered that thermal power plants with CO2 capture and storage (CCS) might be operated as load-following units in future energy systems with higher integration of variable renewable energy sources [1]. The Carbon Capture and Storage update 2014 concludes that the financial case for CCS requires that it operates in a flexible manner, and considers load-following ability as extremely important to the long-term economics [2]. Therefore, interest has grown in the field of operational flexibility of thermal power plants with CCS. A key aspect of operational flexibility of power plants with post-combustion CO2 capture using amines is the transient behavior of the capture process, i.e. the time dependent behavior of the PCC plant when varying operating conditions. Pilot plant testing allows analyzing flexible operation of the process [3-5]. Nevertheless, pilot plant testing requires expensive resources and normally a limited amount of transient testing can be conducted during test campaigns.
The scarcity of published transient performance data from pilot plants together with the limited operational experience from commercial-scale post-combustion capture plants, claims for an interest within the research community for the development of dynamic process simulation models. Dynamic process models are considered as invaluable tools that can help studying different aspects of the transient behavior of PCC plants. The models allow studying various transient events, as well as developing and implementing optimal control strategies. In addition, computational tools and process models can contribute to identify process bottlenecks and develop useful knowledge that will contribute to technology development and ease process scale-up. However, the reliability of results from dynamic simulations might be questioned if the dynamic process models have not been validated against experiments or pilot plant transient data. Thus, validation of dynamic process models is necessary [6-9]. According to Bui et al. [10], further research must focus on producing transient pilot plant data for increasing knowledge on real plant transient performance and for dynamic process model validation in order to ensure reliability of simulation results.
The objectives of this work were:
- Generate a set of steady-state and transient plant data that can be used for dynamic process model validation.
- Develop and validate a dynamic process model of the amine-based TSA plant at CO2 Technology Center Mongstad for flue gas from a natural gas fueled power plant.
Steady-state and dynamic experiments were conducted by TCM DA during an MEA test campaign at the post- combustion amine pilot plant at TCM DA treating flue gas from a natural gas fueled power plant. The steady-state data sets reflect a wide range of operating conditions while the dynamic experiments consist of set-point changes in exhaust gas volumetric flow rate fed to the absorber. In this work, a dynamic process model of the amine-based plant at TCM DA was built with the open physical modeling language Modelica [11], by means of the commercial tool Dymola [12]. After processing the pilot plant data, validation of the overall process model has been conducted with the steady-state and transient data by comparing the prediction of the overall process model of the PCC plant with the pilot plant data. In this paper, the validation with nine steady-state cases and two transient events is presented.
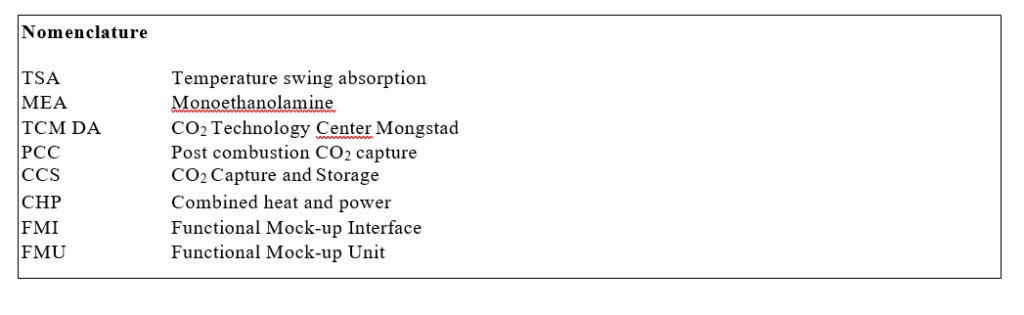
2. TCM DA amine pilot plant configured for CHP flue gas treatment
CO2 Technology Centre Mongstad has an installed pilot-scale amine-based temperature swing absorption (TSA) process plant next to the Statoil refinery in Mongstad, Norway. TCM DA has recently conducted a test campaign with 30% aqueous monoethanolamine (MEA), operated from 6 July until 17 October 2015. The work is part of the continuous effort of TCM DA on generating better understanding of the performance of the non-proprietary aqueous MEA solvent system. From TCM DA’s perspective, one of the objectives of MEA test campaigns is to provide understanding of the transient operations of the amine plant [13]. A detailed description of the flexible and fully instrumented TCM DA plant can be found in Hamborg et al. [14]. In the following it is presented a brief description of the TCM DA PCC pilot plant configured for flue gas cleaning from natural gas fueled power plant.
The exhaust gas, with a CO2 content of about 3.5 vol%, comes from the natural gas combined heat and power plant (CHP) placed next to the TCM DA facility. The amine pilot plant treats a fraction of about 3% of the total exhaust gas originating from the two GE 9001E gas turbines operating at design load at the CHP plant. The total capacity of the pilot plant for CHP flue gas is 60000 Sm3/hr and it is capable of capturing around 80 ton CO2/day. Figure 1 shows a simplified process flowsheet for TCM DA amine plant operated with CHP flue gas. An induced draft blower is present at the plant to overcome pressure drops and blow the flue gas flow. It has variable speed drives that allow manipulating the flue gas volumetric flow rate fed to the absorber. The flue gas flows through a direct contact cooler that cools down and saturates the flue gas by a counter-current water flow.
The absorber column consists of a rectangular polypropylene-lined concrete column with a cross-section of
3.55 x 2 m and a total height of 62 m. It has three absorber packed sections consisting of Koch Glitsch Flexipac 2X structured stainless-steel packing of 12 m, 6 m and 6 m. Two water-wash systems are operated in the upper part of the absorption tower, consisting of two sections of Koch Glitsch Flexipac 2Y HC structured stainless-steel packing. The absorber in TCM DA has the flexibility option to use different packing heights (12, 18 or 24 m). During the tests presented in this paper, 24 m of absorber packing were utilized (12 bottom + 6 middle + 6 top). There are 4 temperature sensors radially distributed in the absorber column per meter of absorber packing in the axial direction. This makes a total of 96 temperature sensors within packed segments.
The CHP stripper with overhead condenser system consists of a 1.3 m diameter column of Koch Glitsch Flexipac 2X structured stainless-steel packing of 8 m, and a rectifying water-wash region with Koch Glitsch Flexipac 2Y HC structured stainless-steel packing of 1.6 m of height. There are 4 temperature sensors radially distributed in the
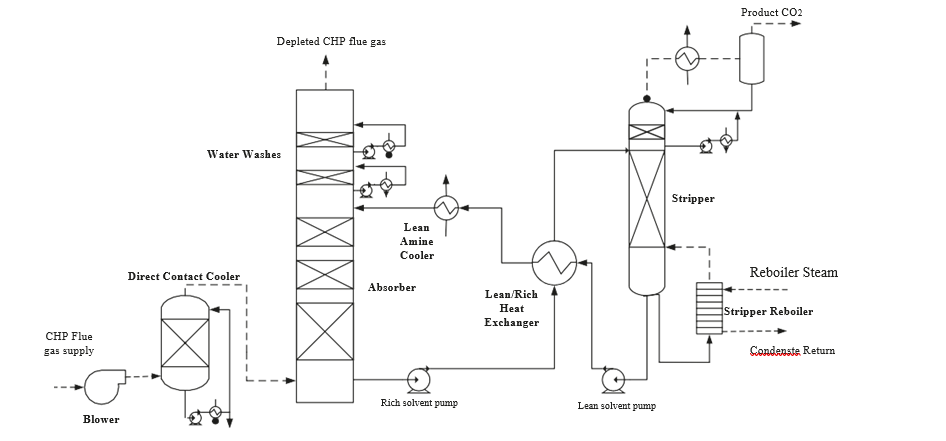
Figure 1. Simplified flowsheet of the TCM amine plant for CHP flue gas.
absorber column per meter of absorber packing in the axial direction. The total number of temperature sensors within packed segments is therefore 28. The stripper reboiler consists of a thermosiphon steam-driven system that provides the required heat for the stripping process. A plate and frame heat exchanger allows heat integration between the absorber and stripper columns, where the hot lean amine solution coming from the stripper heats up the rich amine solution. In addition, a lean amine cooler is utilized to control the lean solution temperature introduced at the top of the absorber packing sections.
3. Steady-state and transient operating cases from the MEA test campaign
With the purpose of dynamic model validation under steady-state operating conditions, a set of nine steady-state plant operation cases from the MEA test campaign were utilized. The tests were conducted with 30 wt% aqueous MEA, and comprise a wide range of operating conditions with various combinations of rich solvent flow rate and reboiler duty. Table 1 shows the steady-state cases generated during the test campaign that are used in this work. Cases 1 to 5 were obtained by varying rich solvent mass flow rate when operating the absorber at 80% volumetric flue gas flow rate capacity with a CO2 capture target of 85%. The mass based L/G ratios on the absorber range from 1.34 to 0.75 for cases 1 to 5. Cases 6 to 9 were obtained from the steady-state operation of the plant achieved in between the four transient tests (refer to Table 2).
A transient event happens when the plant is brought from one operating point to another. During transient testing key manipulated variables (inputs) of the plant are changed to observe how the process variables evolve over time from one steady-state operating point until a new steady-state operating point is reached. The purposes of these experiments are to increase knowledge of the process under transient conditions and to generate a set of data for assessing the validity of dynamic process models at the plant scale. It is desirable that the transient data represents the main dynamics of the plant. Table 2 includes the test matrix for the set-point change experiments conducted during the autumn 2015 MEA test campaign at TCM DA. The experiments consist of set-point changes in main inputs to the pilot plant, i.e., rich solvent flow rate, flue gas volumetric flow rate into the absorber and steam flow rate to reboiler. In this paper, tests 2 and 3 are presented for the purpose of dynamic process model validation. These two tests represent set point step-changes in flue gas volumetric flow rate fed to the absorber.
The responses and performance of the pilot plant was logged. The data was extracted every 30 seconds in order to reduce the data load. Logged data includes:
- Gas analyzers at the inlet of the absorber, outlet of the absorber, and CO2 rich to stack.
- Main liquid and gas flow rates.
- Main process temperatures, including absorber and stripper temperatures.
- Pressures and pressure drops at different components of the plant.
- Online solvent analysis measurements include pH, density and conductivity, at the inlet and outlet of the absorber (lean and rich solvent).
- Liquid hold-ups distribution at different components of the plant.
- Main active controller set-points and tuning parameters.
Solvent samples were taken during steady-state conditions at the inlet and outlet of the absorber for posterior analysis in the lab, in order to obtain the CO2 lean and rich solvent loadings. Actual reboiler duty was estimated based on logged measurement data of steam temperatures, pressures and mass flow rate as indicated in Thimsen et al. [15]. In order to assess the validity of the process model, temperature profiles of the absorber and stripper columns were utilized. Each of the measured temperature points included in the steady-state absorber temperature profiles is the average over time during steady-state conditions, of the averaged 4 temperature measurements of the sensors radially distributed within the absorber column, at the given axial position of the column.
The tests were run with a total inventory of aqueous MEA of about 38.2 m3. For process simulations, it is of importance to understand how the solvent inventory is distributed within the different components of the plant. Therefore, liquid hold-ups at different parts of the plant were registered for the steady-state operating cases.
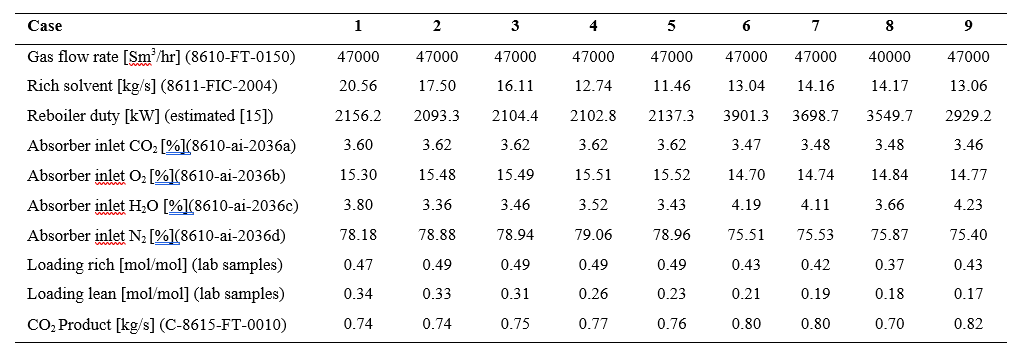
Table 1. Steady-state data for the nine operating cases selected from the MEA test campaign. The plant was operated with 30 wt% aqueous MEA and 24 meters of absorber packing. Note that standard conditions are 15 ºC and 1 atm. The tag IDs for the instrumentation utilized are included.
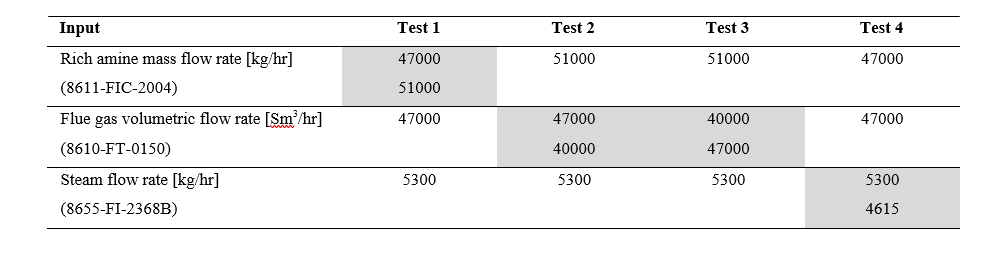
Table 2. Test matrix for the set-point change experiments conducted during the Autumn 2015 MEA test campaign at TCM DA. The tag IDs for the instrumentation used is included.
4. Dynamic process models of the CO2 chemical absorption process with aqueous MEA
A dynamic process model of the amine-based TSA plant at TCM DA was built with the open physical modeling language Modelica [11], by means of the commercial modeling and simulation tool Dymola [12]. Modelica allows for component-based modeling, and the component models consist of systems of differential and algebraic equations. The overall plant model consists of models for the absorber and stripper columns, sumps, internal heat exchanger, reboiler, condensers, flow resistances, pumps, valves, measurements and controllers. The process models were obtained from a Modelica library from Modelon AB [16] and have been presented elsewhere [17, 18]. In this work, the component models were configured, parameterized and modified in order to obtain a dynamic process model of the TSA plant at TCM DA considering the main process equipment, size, geometry, material and solvent inventory during the experiments. In addition, the regulatory control layer of the plant was implemented in the process model, considering the control structure at the PCC pilot plant.
Absorption and desorption columns are modeled considering the two-film theory approach, thus thermodynamic equilibrium is assumed at the liquid and gas interface. Packed sections consider rate-based approach for modeling interface mass transfer, with mass transfer coefficients for CO2 and H2O by Onda et al. [19], and enhanced mass transfer due to chemical reactions is implemented via a pseudo-first order enhancement factor [7]. Chemical equilibrium is considered in all model parts, both at interface and liquid bulk, with chemical equilibrium constants obtained empirically from Bötinger [20].
Heats of reaction are inferred from the equilibrium constant via the van’t Hoff equation. Sensible heat transfer between phases is correlated to gas-phase mass transfer coefficient (Cohilton-Colburn analogy), while heat of solution and evaporation is calculated as a function of temperature but is constant with solvent loading. Ideal gas law applies to the gas phase, which is only composed of CO2 , O2, H2O and N2. The pressure of the system p is determined by gas phase pressure drop from a known operating point and a quadratic correlation with gas velocity.
A simplified washer component is included in the head of the column. It is modelled as a simple volume with phase separation. Its purpose is to cool down the gas flow to a temperature given as an input signal and condense as much vapor as required to reach saturation in the gas phase. Water balance is ensured by a make-up water source in the absorber sump that controls the H2O mass balance of the plant. Note that in this model MEA is considered non- volatile, which means that it is only present in the liquid phase. This implies that MEA make-up source is not required in the overall dynamic process model. This is not the case for the real plant, where MEA make-up is required for operation.
The numerical solver DASSL was selected in Dymola for solution of the resulting system of differential and algebraic equations. The process model was exported as a co-simulation Functional Mock-up Unit (FMU) via FMI technology (Functional Mockup Interface) [21]. Simulations and validation were carried out in Microsoft Excel® environment via a FMI-add-in for Excel® [22].
5. Dynamic model validation results
5.1 Dynamic model validation using steady-state operational data.
The approach to overall PCC plant model development and validation followed in this work was to initially separate the plant in three main parts: absorber, lean/rich cross heat exchanger and stripper with reboiler. Proper boundary conditions were specified for each part of the process. Steady-state data measured at the pilot plant were used as inputs to the boundary conditions of each section of the process, and the main outputs from the model were compared with the plant data. This involves checking absorption and desorption rates, temperature profiles in the absorber and stripper, and lean and rich CO2 loadings. The task required tuning of uncertain model parameters (tuners) in order to obtain a better agreement between measured plant performance and behavior predicted by the model. Uncertain parameters include enhancement factors and pre-multiplying factors for adjustment of effective interface area correlations. Then, the overall PCC plant process model was closed by connecting the different sections of the process and implementing the suitable regulatory control layer. The main model outputs were compared with measured plant data in steady-state for the overall plant. In the following, the results from the overall plant process model validation are presented.
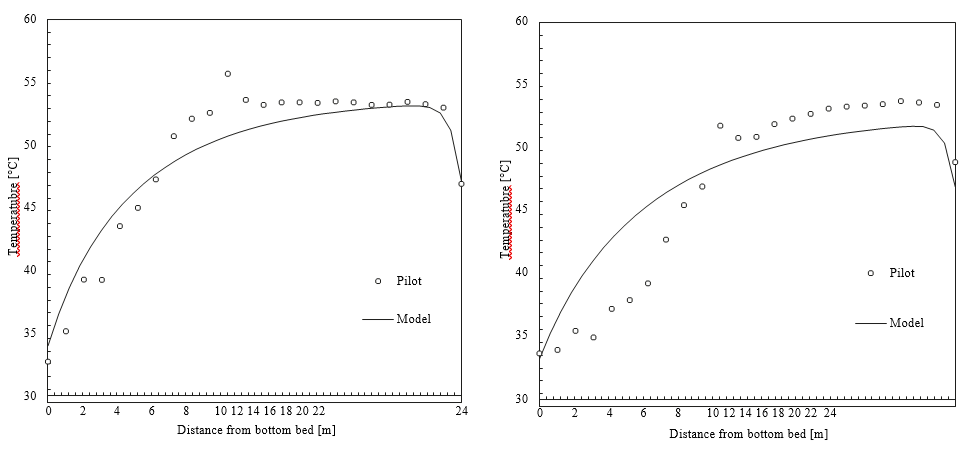
Figure 2. Examples of temperature profiles in absorber and stripper columns during steady-state operating conditions. Left: Case 8. Right: Case 9.
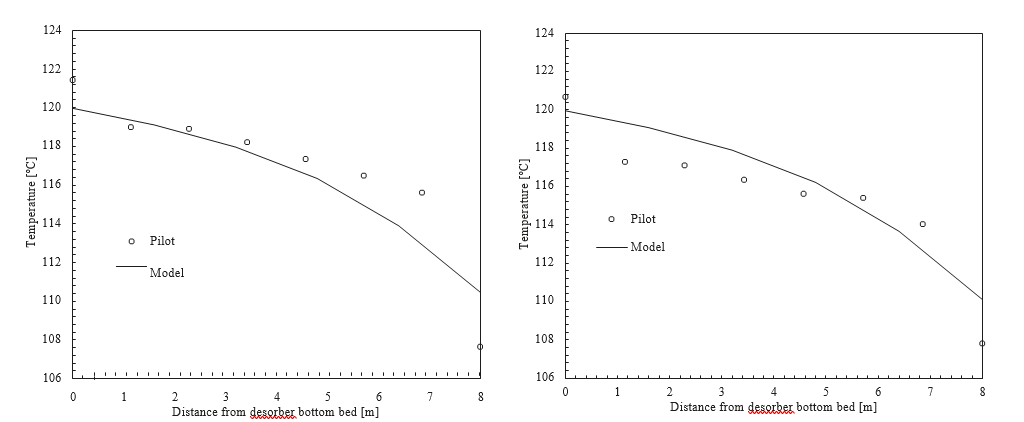
Figure 3. Examples of stripper temperature profiles for two steady-state operating conditions of the PCC pilot plant. Left: Case 8. Right: Case 9 (refer to Table 1).
Examples of temperature profiles within the absorber are shown in Figure 2. Figure 2 (left) shows the absorber temperature profile for the case 8, whereas Figure 2 (right) shows the absorber temperature profile for case 9. Figure 3 (left) shows temperature profiles in the stripper for case 8, whereas Figure 3 (right) shows temperature profiles for stripper in case 9. Note that for cases 8 and 9 the PCC plant is operated with 67 and 80 % flue gas volumetric flow rate capacity in the absorber respectively. Absorber temperature profiles predicted by the model show a good agreement with plant data, especially for case 8. The model is capable to predict properly the trends in temperature along the column. An over prediction is observed in case 9, at the bottom packing below the temperature bulge, while an under prediction is observed from the temperature bulge, within the middle and upper packing. The stripper temperature profile predicted by the process model shows also good agreement with plant data, as illustrated with steady-state cases in Figure 3.
Figure 4 shows the parity plot for lean and rich solvent loadings for the nine steady-state operating cases. It can be observed that the model over predicts the lean and rich loading when compared with the experimental data. The mean percentage error for lean loading is 1.4% and for rich loading 6.7%. There are two steady-state cases where the model shows an under prediction of lean solvent loading. This could be explained by the fact that these two steady-state cases are obtained prior to the injection of anti-foam solution in the plant (cases 6 and 7). Anti-foam is periodically used during MEA test campaigns at TCM to tackle the unideal phenomena in the stripper, and has a direct impact in the performance of the stripper [13]. From the results shown in Figure 4 it can be concluded that the dynamic process model is capable to predict the variability in solvent loading for the steady-state operating cases. The CO2 product flow is under predicted with an average percentage error of 5% for the simulated cases.
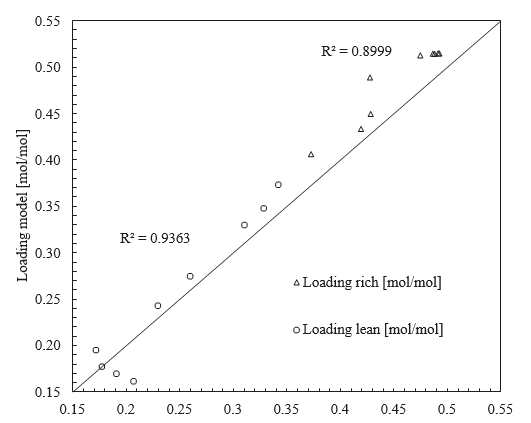
Figure 4. Lean and rich amine CO2 loading parity plots. Model results from overall pilot plant model for nine steady-state operating cases. The mean percentage error for lean loading is 1.4% and for rich loading is 6.7%.
5.2 Dynamic model validation using transient operational data.
Once the steady-state estimation performance of the dynamic process model is validated for the full plant model, the dynamic process model is validated with transient plant data. Transient performance of this process is characterized by long dead times and large lag times in main process variables, resulting in relatively large total stabilization times. This means that this process is considered slow, when it is compared with a change in load in the steam cycle of a power plant. During the test campaign four transient tests were conducted, here two of them involving flue gas volumetric flow rate ramp-down and ramp-up will be presented.
5.2.1 Flue gas volumetric flow rate reduction
The test consisted of set-point reduction of the exhaust gas volumetric flow rate fed to the absorber, from 80% to 67% of the plant capacity, i.e. 47000 Sm3/hr to 40000 Sm3/hr. The purpose was to change the flue gas volumetric flow rate while keeping the rest of the plant process variables constant. Figure 5 shows the three main inputs of the plant for this test. The main controlled drifting variables of the plant during the test were kept constant by the action of the controllers of the regulatory control layer of the plant.
The plant was disturbed by manipulating the speed of the induced draft blower located upstream the direct contact cooler. The blower speed was changed in order to set the flue gas volumetric flow rate at the inlet of the absorber. Step set-point reduction in flue gas volumetric flow rate was applied. As shown in Figure 5, this has resulted in an oscillatory flue gas volumetric flow rate as disturbance to the plant, due to the fact that the blower speed/volumetric flue gas flow rate controller is a PI controller. Steam mass flow rate was maintained constant, while the solvent mass flow rate had small amplitude oscillations around the set-point. In order to compare the transient plant data with the actual plant data, the measured flue gas volumetric flow rate was introduced as an input trajectory to the dynamic process model. This means that the same disturbance applied to the plant during the test campaign, was applied to the dynamic process model for simulation. In addition, averaged value of the time series of the measured rich solvent mass flow rate and the estimated reboiler duty was applied as input to the dynamic process model.
Figure 6 shows the response on CO2 product flow rate to the plant input. It was observed an input/output dead time of 40 minutes between flue gas volumetric flow rate and CO2 product mass flow rate. This means that for a change in the flue gas flow rate input to the plant, no changes are observed in the product CO2 flow until around 40 minutes later. Therefore, the system acts as a buffer to load change driven by flue gas volumetric flow rate change at absorber inlet. In addition, it takes around 4 hours to reach the new steady-state operating point. In addition, a significant lag time was found in stabilization of temperature profiles in the absorber (1 hour) and stripper columns (3-4 hours), not shown. It can be observed in Figures 6 and 7 that the process model is capable of predicting the main process dynamics for CO2 product mass flow rate and rich and lean solvent CO2 loadings.
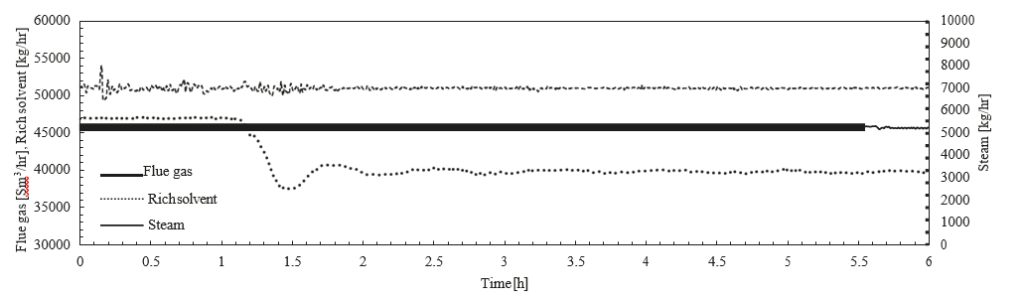
Figure 5. Main inputs to the plant for test with flue gas volumetric flow rate set-point reduction from 47000 [Sm3/hr] to 40000 Sm3/hr. Rich solvent flow rate from absorber kg/hr and steam flow to reboiler kg/hr.
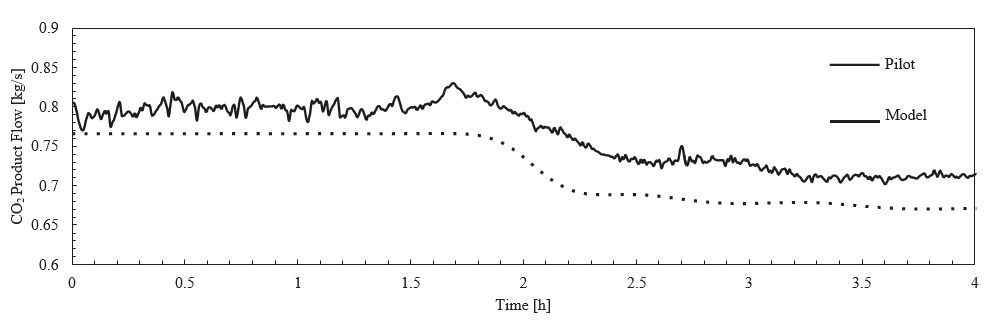
Figure 6. CO2 Product flow rate kg/s.
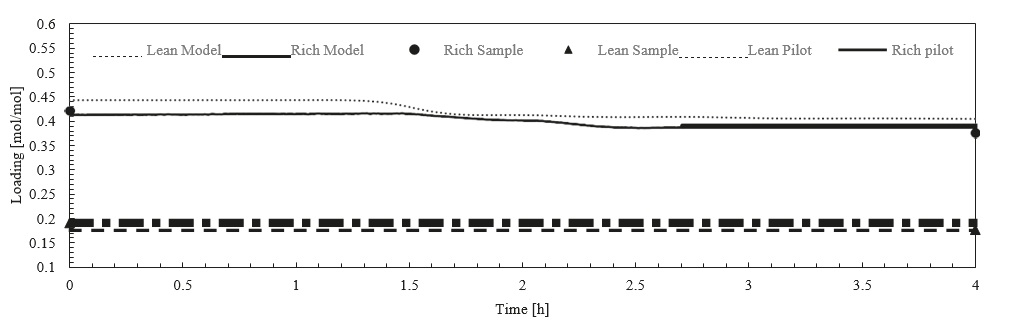
Figure 7. CO2 lean and rich solvent loadings during test 2 (refer to table 2). Lean and rich pilot curves are based on a correlation for total alkalinity, density and temperature of the solvent, measured online at the plant. Lab samples were taken before and after the test.
5.2.2 Flue gas volumetric flow rate increase.
This test consist of set-point increase of the flue gas volumetric flow rate fed to the absorber, from 67% to 80% of the plant capacity, i.e. 40000 Sm3/hr to 47000 Sm3/hr. Figure 8 shows the three main inputs of the plant during the test. As in the previous test, an oscillatory behavior of the flue gas volumetric flow rate around the new set point is observed. The same approach with the measured input to the plant as input trajectory to the dynamic process model was applied. The plant acts as a buffer for flue gas volumetric flow rate changes as shown in Figure 9. Around 20 minutes dead time input/output from flue gas volumetric flow rate to CO2 product mass flow rate was observed. Figure 9 shows the CO2 product flow for the model and the pilot plant data and Figure 10 shows the plant and model response for this disturbance in terms of CO2 lean and rich solvent loadings. A mismatch of 15 min for CO2 product flow rate predicted by the process model is observed. A similar offset as in the previous test is observed, with a steady- state under prediction of CO2 product flow rate. Despite of the steady-state offset shown on solvent CO2 loadings prediction, it is observed a good prediction of the main dynamics, refer to Figure 10. It can be concluded that the process dynamics are well captured by the process model.
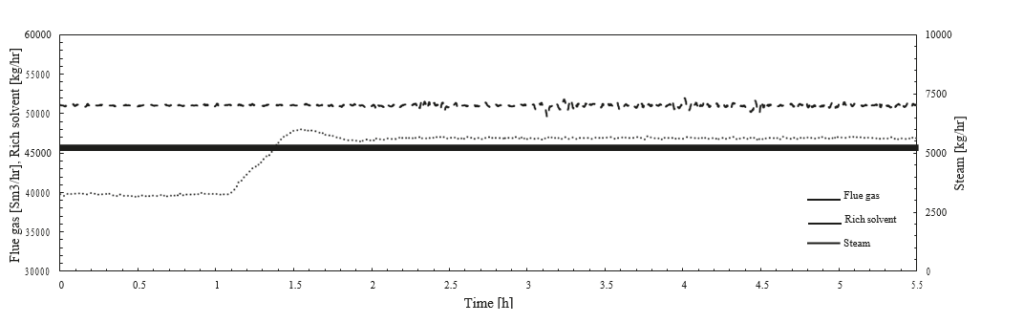
Figure 8. Main inputs to the plant. Flue gas volumetric flow rate set-point change from 47000 [Sm3/hr] to 40000 Sm3/hr. Rich solvent flow rate from absorber kg/hr and steam flow to reboiler kg/hr.
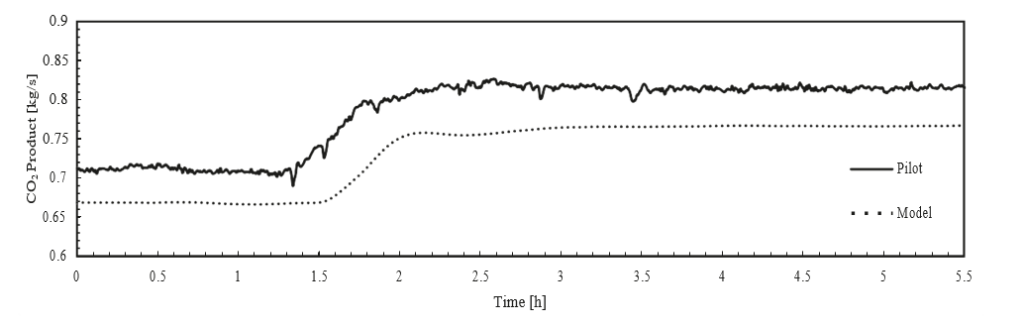
Figure 9. CO2 product kg/hr. Results from test 3 on flue gas volumetric flow rate set-point increase (refer to Table 2).
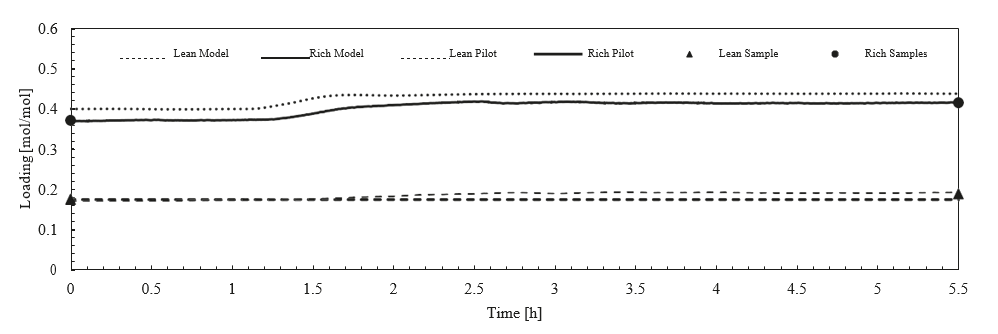
Figure 10. CO2 lean and rich solvent loadings during test 3 (refer to Table 2). Loading pilot curves are based on a correlation for total alkalinity, density and temperature of the solvent, measured at the plant. Lab samples were taken before and after the test.
6. Discussion
The task of dynamic process model validation of the post-combustion CO2 absorption with aqueous MEA requires the generation of suitable data sets including both steady-state and transient data. Ideally, the steady-state data should reflect a wide range of operating conditions of the PCC plant. The steady-state data utilized in this work consists of a wide range of operating conditions achieved by changing rich solvent mass flow rate and reboiler heat duty. As shown in Table 1, nine steady-state cases were gathered from the MEA test campaign. The cases include operation of the PCC plant with mass based L/G ratios on the absorber ranging from 1.34 to 0.75, when operating the absorber at 80% capacity and with a capture rate of 85% (cases 1 to 5).
During campaigns at TCM with 30% aqueous MEA, unideal behaviour occurs in the stripper bed and it is handled by addition of anti-foam solution. As shown in literature [13], the addition of anti-foam solution has a significant effect on stripper temperature profile at TCM DA pilot plant for CHP, and especially on specific reboiler duty at low lean amine loadings. Cases 6 and 7 were run before the addition of the anti-foam solution and it has been shown in Figure 4 that the model prediction under estimates lean loading only for these two specific cases. It is advised to check if anti-foam solution was used during the tests, if the data is to be used for process model validation. Anti-foam was introduced in the plant between the transient tests presented in this paper. If required, sufficient time between the tests should be allowed so that steady-state conditions are reached before and after adding anti-foam solution.
The post-combustion TSA process design with solvent recirculation from the stripper to the absorber in a closed- loop makes modeling and validation of the full plant challenging. Modeling errors and inaccuracies in one component of the plant will easily propagate towards other parts of the process. Therefore, a systematic approach is recommended beginning with validation of the separate models of absorber, stripper with reboiler, and heat exchanger sections. In this work, the overall process model is finally developed by joining the different sections and validated with the steady- state and transient pilot data. The intended application of the process model is for transient estimation and plantwide control studies.
Column temperature profiles accurate prediction is of importance since temperature affects phase equilibrium calculation at the gas-liquid interface and liquid phase. In addition, several model parameters and thermophysical properties depend on temperature. These include heat capacity, CO2 solubility, water heat of condensation, heats of reaction and equilibrium constants. The pilot plant absorber and stripper columns temperature profiles are calculated as an averaged value of the temperature measurements from the sensors distributed in the radial plane at the given axial position of the column. The individual temperature measurements are considered reliable, and the resulting temperature profiles are clear and reasonable. Nevertheless, it should be mentioned that some sensors are closer to the wall while some are closer to the center of the packing, thus a small maximum variation (<6 ºC) is observed between the measurements at a given radial position. The variation is different for different operating conditions of the columns and radial planes. The aggregated effect of above-mentioned aspects makes validation of the absorber temperature profiles challenging. Based on the results presented in Figures 2 and 3, it is considered that the dynamic process model is capable of predicting temperature profiles of both absorber and stripper columns with good accuracy for the purpose considered in this work. Tuning of the pre-multiplying factor of the mass transfer enhancement factor has been required (0.2 in absorber and 0.09 in stripper).
Lean and rich CO2 loadings are over-predicted by the dynamic process model. Lean and rich loadings are dependent of each other, and modeling errors will easily propagate. In addition, actual reboiler heat duty has been estimated from steam measurements in the plant as suggested by Thimsen et al. [15]. Nevertheless, that value is not truly representative of regeneration energy due to external factors such as changes in ambient conditions and heat loses through non-insulated pipes and equipment [5]. An under-prediction of lean loading is found on cases 6 and 7. It is believed that this is because the plant was operated before addition of anti-foam solution during these cases as well as due to small deviations on MEA concentration from 30 wt% during that period. The mean percentage error for lean loading is 1.4 % and for rich loading 6.7%. It can be concluded that the process model is capable of predicting the variability in lean and rich loading for the range of operating conditions of the PCC plant. The process model under- predicts CO2 product mass flow rate within <5% for all steady-state cases, being the precision uncertainty of the product CO2 flow measurement 1% (Vortex FT-0010) [13]. This under prediction is illustrated in the transient cases (Figures 6 and 9).
Dynamic process validation with the two tests involving volumetric flue gas flow rate reduction and increase has been presented in this paper. The experiment shows that the system acts as a buffer to load change driven by flue gas volumetric flow rate change at absorber inlet, and long dead times input/output in terms of CO2 product flow are observed (around 20-40 minutes). The results from the model show that the model development has been successful to predict the main process dynamics. This includes CO2 lean and rich loadings and CO2 product flow response to the disturbances.
7. Conclusions
A dynamic process model of the overall amine-based TSA plant at TCM DA was built for the purpose of model validation with a new set of steady-state and transient plant data. It is concluded that the dynamic process model is capable of estimating the temperature profiles of absorber and stripper columns with good accuracy for the purpose of application. Tuning of the pre-multiplying factor for calibration of the enhancement factor has been required. An over prediction of the model for lean and rich CO2 loadings has been reported, being mean percentage errors <1.5% and <6.7%. The process model is capable of predicting the variability of lean and rich loadings for a wide range of steady-state operating conditions. In addition, an under prediction of CO2 product flow rate has been observed (<5%). The main process dynamics of the pilot plant under flue gas volumetric flow changes is captured by the process model. The validated process model developed in this work will be used to analyze the TCM plant transient performance and expanded to a full-scale plant model to predict transient performance of a natural gas combined cycle power plant integrated with post-combustion CO2 capture.
Acknowledgements
The authors acknowledge TCM DA and the Department of Energy and Process Engineering at NTNU-Norwegian University of Science and Technology for funding this project. The Faculty of Engineering Science NTNU-Statoil Publication Grant 2016 is also acknowledged. The authors want to acknowledge Espen S. Hamborg from TCM DA for his contribution to establish the ongoing collaboration between TCM DA and the Thermal Energy Research Group at the Department of Energy and Process Engineering at NTNU.
References
- R.M. Montañés, M. Korpås, L.O. Nord, S. Jaehnert, Identifying Operational Requirements for Flexible CCS Power Plant in Future Energy Systems, Energy Procedia, 86 (2016) 22-31.
- M.E. Boot-Handford, J.C. Abanades, E.J. Anthony, M.J. Blunt, S. Brandani, N. Mac Dowell, J.R. Fernandez, M.-C. Ferrari, R. Gross, J.P. Hallett, R.S. Haszeldine, P. Heptonstall, A. Lyngfelt, Z. Makuch, E. Mangano, R.T.J. Porter, M. Pourkashanian, G.T. Rochelle, N. Shah, J.G. Yao, P.S. Fennell, Carbon capture and storage update, Energy & Environmental Science, 7 (2014) 130-189.
- R. Faber, M. Köpcke, O. Biede, J.N. Knudsen, J. Andersen, Open-loop step responses for the MEA post-combustion capture process: Experimental results from the Esbjerg pilot plant, Energy Procedia, 4 (2011) 1427-1434.
- P. Tait, B. Buschle, I. Ausner, P. Valluri, M. Wehrli, M. Lucquiaud, A pilot-scale study of dynamic response scenarios for the flexible operation of post-combustion CO2 capture, International Journal of Greenhouse Gas Control.
- M. Bui, I. Gunawan, V. Verheyen, P. Feron, E. Meuleman, Flexible operation of CSIRO’s post-combustion CO2 capture pilot plant at the AGL Loy Yang power station, International Journal of Greenhouse Gas Control.
- N. Enaasen Flø, H. Knuutila, H.M. Kvamsdal, M. Hillestad, Dynamic model validation of the post-combustion CO2 absorption process, International Journal of Greenhouse Gas Control, 41 (2015) 127-141.
- H.M. Kvamsdal, J.P. Jakobsen, K.A. Hoff, Dynamic modeling and simulation of a CO2 absorber column for post-combustion CO2 capture, Chemical Engineering and Processing: Process Intensification, 48 (2009) 135-144.
- C. Biliyok, A. Lawal, M. Wang, F. Seibert, Dynamic modelling, validation and analysis of post-combustion chemical absorption CO2 capture plant, International Journal of Greenhouse Gas Control, 9 (2012) 428-445.
- J. Gaspar, A.-M. Cormos, Dynamic modeling and absorption capacity assessment of CO2 capture process, International Journal of Greenhouse Gas Control, 8 (2012) 45-55.
- M. Bui, I. Gunawan, V. Verheyen, P. Feron, E. Meuleman, S. Adeloju, Dynamic modelling and optimisation of flexible operation in post- combustion CO2 capture plants—A review, Computers & Chemical Engineering, 61 (2014) 245-265.
- Modelica Association, https://www.modelica.org/.
- Dassault Systems, Dymola, http://www.3ds.com/products-services/catia/products/dymola.
- N. Brigman, M.I. Shah, O. Falk-Pedersen, T. Cents, V. Smith, T. De Cazenove, A.K. Morken, O.A. Hvidsten, M. Chhaganlal, J.K. Feste, G. Lombardo, O.M. Bade, J. Knudsen, S.C. Subramoney, B.F. Fostås, G. de Koeijer, E.S. Hamborg, Results of Amine Plant Operations from 30 wt% and 40 wt% Aqueous MEA Testing at the CO2 Technology Centre Mongstad, Energy Procedia, 63 (2014) 6012-6022.
- E.S. Hamborg, V. Smith, T. Cents, N. Brigman, O.F. Pedersen, T. De Cazenove, M. Chhaganlal, J.K. Feste, Ø. Ullestad, H. Ulvatn, O. Gorset, I. Askestad, L.K. Gram, B.F. Fostås, M.I. Shah, A. Maxson, D. Thimsen, Results from MEA testing at the CO2 Technology Centre Mongstad. Part II: Verification of baseline results, Energy Procedia, 63 (2014) 5994-6011.
- D. Thimsen, A. Maxson, V. Smith, T. Cents, O. Falk-Pedersen, O. Gorset, E.S. Hamborg, Results from MEA testing at the CO2 Technology Centre Mongstad. Part I: Post-Combustion CO2 capture testing methodology, Energy Procedia, 63 (2014) 5938-5958.
- Modelon, Post-combustion capture with amine solutions. http://www.modelon.com/industries/energy-process/carbon-capture-and- sequestration/.
- K. Prölß, H. Tummescheit, S. Velut, J. Åkesson, Dynamic model of a post-combustion absorption unit for use in a non-linear model predictive control scheme, Energy Procedia, 4 (2011) 2620-2627.
- S.Ó. Garðarsdóttir, F. Normann, K. Andersson, K. Prölß, S. Emilsdóttir, F. Johnsson, Post-combustion CO2 capture applied to a state-of-the- art coal-fired power plant—The influence of dynamic process conditions, International Journal of Greenhouse Gas Control, 33 (2015) 51-62.
- K. Onda, H. Takeuchi, Y. Okumoto, Mass transfer coefficients between gas and liquid phases in packed columns, Journal of Chemical Engineering of Japan, 1 (1968) 56-62.
- W. Böttinger, NMR-spektroskopische Untersuchung der Reaktivabsorption von Kohlendioxid in wässrigen Aminlösungen, VDI-Verlag, 2006.
- Functional Mock-up Interface. https://www.fmi-standard.org/.
- Modelon, FMI-Add-In for Excel. http://www.modelon.com/products/fmi-tools/fmi-add-in-for-excel/.