9. Dynamic process model development and validation with transient plant data collected from an MEA test campaign at the CO2 Technology Center Mongstad (2017)
Rubén M. Montañésa*, Nina E. Fløb, Rohan Duttaa, Lars O. Norda, Olav Bollanda
aDepartment of Energy and Process Engineering, NTNU – Norwegian University of Science and Technology, NO-7491 Trondheim, Norway bCO2 Technology Center Mongstad (TCM DA), 5954 Mongstad, Norway. *Corresponding author
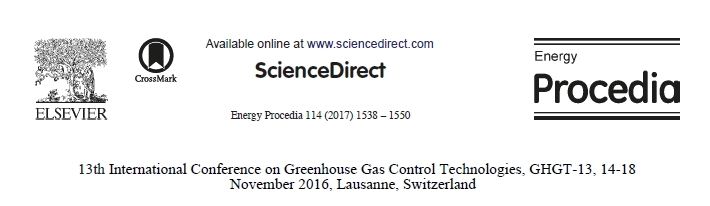
(http://creativecommons.org/licenses/by-nc-nd/4.0/).
Peer-review under responsibility of the organizing committee of GHGT-13.
doi: 10.1016/j.egypro.2017.03.1284
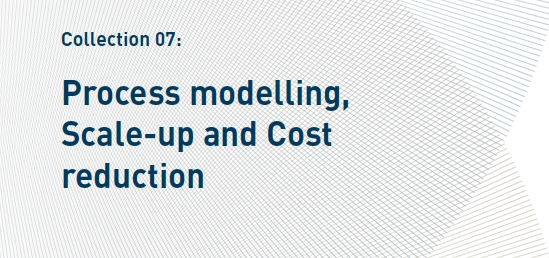
This work focuses on the development and validation of a dynamic process model of the post-combustion CO2 chemical absorption process with temperature swing absorption (TSA) using aqueous monoethanolamine (MEA) as solvent. A new set of steady-state and transient cases were generated during an MEA test campaign at the amine pilot plant at CO2 Technology Center Mongstad (TCM DA). Nine steady-state cases comprising a wide range of operating conditions of the plant and two transient tests consisting of flue gas volumetric flow rate step-changes were utilized for the purpose of dynamic process model validation of the overall pilot plant process model. It is concluded that the dynamic process model is capable of estimating the absorber and stripper columns temperature profiles with good accuracy after tuning of model parameters. An over-prediction of the model for lean and rich CO2 loadings has been reported, being mean percentage errors <1.5% for lean loading and <6.7% for rich loading. In addition, an under prediction of CO2 product flow rate has been observed (<5%). The process model is capable of predicting the variability of lean and rich loadings for the range of steady-state operating conditions. The main process dynamics of the pilot plant under flue gas volumetric flow rate set-point step changes is captured by the process model.
Post-combustion CO2 capture (PCC) with amines is considered one of the more mature technologies that can contribute to reduce anthropogenic CO2 emissions to the atmosphere from fossil-fueled thermal power plants. It is considered that thermal power plants with CO2 capture and storage (CCS) might be operated as load-following units in future energy systems with higher integration of variable renewable energy sources [1]. The Carbon Capture and Storage update 2014 concludes that the financial case for CCS requires that it operates in a flexible manner, and considers load-following ability as extremely important to the long-term economics [2]. Therefore, interest has grown in the field of operational flexibility of thermal power plants with CCS. A key aspect of operational flexibility of power plants with post-combustion CO2 capture using amines is the transient behavior of the capture process, i.e. the time dependent behavior of the PCC plant when varying operating conditions. Pilot plant testing allows analyzing flexible operation of the process [3-5]. Nevertheless, pilot plant testing requires expensive resources and normally a limited amount of transient testing can be conducted during test campaigns.
The scarcity of published transient performance data from pilot plants together with the limited operational experience from commercial-scale post-combustion capture plants, claims for an interest within the research community for the development of dynamic process simulation models. Dynamic process models are considered as invaluable tools that can help studying different aspects of the transient behavior of PCC plants. The models allow studying various transient events, as well as developing and implementing optimal control strategies. In addition, computational tools and process models can contribute to identify process bottlenecks and develop useful knowledge that will contribute to technology development and ease process scale-up. However, the reliability of results from dynamic simulations might be questioned if the dynamic process models have not been validated against experiments or pilot plant transient data. Thus, validation of dynamic process models is necessary [6-9]. According to Bui et al. [10], further research must focus on producing transient pilot plant data for increasing knowledge on real plant transient performance and for dynamic process model validation in order to ensure reliability of simulation results.
The objectives of this work were:
- Generate a set of steady-state and transient plant data that can be used for dynamic process model validation.
- Develop and validate a dynamic process model of the amine-based TSA plant at CO2 Technology Center Mongstad for flue gas from a natural gas fueled power plant.
Steady-state and dynamic experiments were conducted by TCM DA during an MEA test campaign at the post- combustion amine pilot plant at TCM DA treating flue gas from a natural gas fueled power plant. The steady-state data sets reflect a wide range of operating conditions while the dynamic experiments consist of set-point changes in exhaust gas volumetric flow rate fed to the absorber. In this work, a dynamic process model of the amine-based plant at TCM DA was built with the open physical modeling language Modelica [11], by means of the commercial tool Dymola [12]. After processing the pilot plant data, validation of the overall process model has been conducted with the steady-state and transient data by comparing the prediction of the overall process model of the PCC plant with the pilot plant data. In this paper, the validation with nine steady-state cases and two transient events is presented.
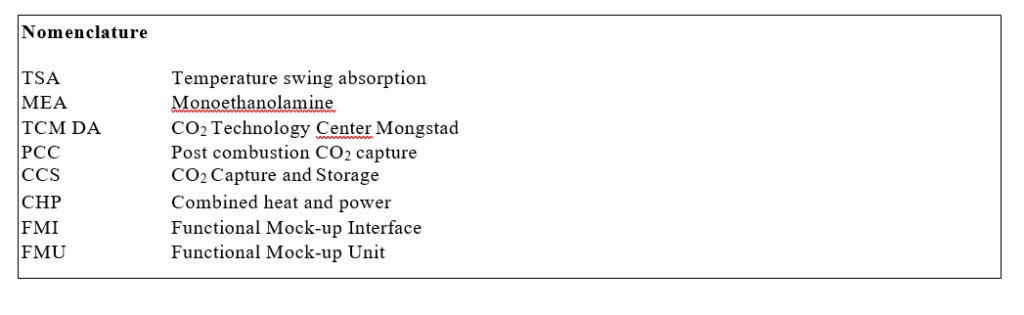
CO2 Technology Centre Mongstad has an installed pilot-scale amine-based temperature swing absorption (TSA) process plant next to the Statoil refinery in Mongstad, Norway. TCM DA has recently conducted a test campaign with 30% aqueous monoethanolamine (MEA), operated from 6 July until 17 October 2015. The work is part of the continuous effort of TCM DA on generating better understanding of the performance of the non-proprietary aqueous MEA solvent system. From TCM DA’s perspective, one of the objectives of MEA test campaigns is to provide understanding of the transient operations of the amine plant [13]. A detailed description of the flexible and fully instrumented TCM DA plant can be found in Hamborg et al. [14]. In the following it is presented a brief description of the TCM DA PCC pilot plant configured for flue gas cleaning from natural gas fueled power plant.
The exhaust gas, with a CO2 content of about 3.5 vol%, comes from the natural gas combined heat and power plant (CHP) placed next to the TCM DA facility. The amine pilot plant treats a fraction of about 3% of the total exhaust gas originating from the two GE 9001E gas turbines operating at design load at the CHP plant. The total capacity of the pilot plant for CHP flue gas is 60000 Sm3/hr and it is capable of capturing around 80 ton CO2/day. Figure 1 shows a simplified process flowsheet for TCM DA amine plant operated with CHP flue gas. An induced draft blower is present at the plant to overcome pressure drops and blow the flue gas flow. It has variable speed drives that allow manipulating the flue gas volumetric flow rate fed to the absorber. The flue gas flows through a direct contact cooler that cools down and saturates the flue gas by a counter-current water flow.
The absorber column consists of a rectangular polypropylene-lined concrete column with a cross-section of
3.55 x 2 m and a total height of 62 m. It has three absorber packed sections consisting of Koch Glitsch Flexipac 2X structured stainless-steel packing of 12 m, 6 m and 6 m. Two water-wash systems are operated in the upper part of the absorption tower, consisting of two sections of Koch Glitsch Flexipac 2Y HC structured stainless-steel packing. The absorber in TCM DA has the flexibility option to use different packing heights (12, 18 or 24 m). During the tests presented in this paper, 24 m of absorber packing were utilized (12 bottom + 6 middle + 6 top). There are 4 temperature sensors radially distributed in the absorber column per meter of absorber packing in the axial direction. This makes a total of 96 temperature sensors within packed segments.
The CHP stripper with overhead condenser system consists of a 1.3 m diameter column of Koch Glitsch Flexipac 2X structured stainless-steel packing of 8 m, and a rectifying water-wash region with Koch Glitsch Flexipac 2Y HC structured stainless-steel packing of 1.6 m of height. There are 4 temperature sensors radially distributed in the
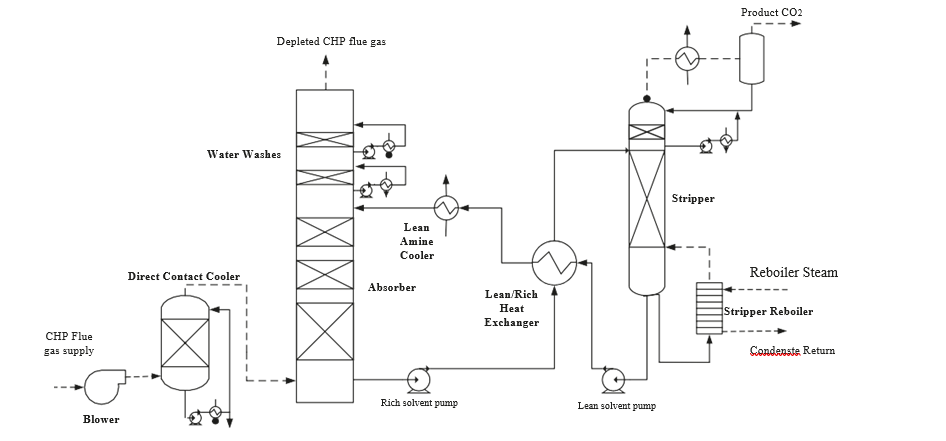
Figure 1. Simplified flowsheet of the TCM amine plant for CHP flue gas.
absorber column per meter of absorber packing in the axial direction. The total number of temperature sensors within packed segments is therefore 28. The stripper reboiler consists of a thermosiphon steam-driven system that provides the required heat for the stripping process. A plate and frame heat exchanger allows heat integration between the absorber and stripper columns, where the hot lean amine solution coming from the stripper heats up the rich amine solution. In addition, a lean amine cooler is utilized to control the lean solution temperature introduced at the top of the absorber packing sections.
With the purpose of dynamic model validation under steady-state operating conditions, a set of nine steady-state plant operation cases from the MEA test campaign were utilized. The tests were conducted with 30 wt% aqueous MEA, and comprise a wide range of operating conditions with various combinations of rich solvent flow rate and reboiler duty. Table 1 shows the steady-state cases generated during the test campaign that are used in this work. Cases 1 to 5 were obtained by varying rich solvent mass flow rate when operating the absorber at 80% volumetric flue gas flow rate capacity with a CO2 capture target of 85%. The mass based L/G ratios on the absorber range from 1.34 to 0.75 for cases 1 to 5. Cases 6 to 9 were obtained from the steady-state operation of the plant achieved in between the four transient tests (refer to Table 2).
A transient event happens when the plant is brought from one operating point to another. During transient testing key manipulated variables (inputs) of the plant are changed to observe how the process variables evolve over time from one steady-state operating point until a new steady-state operating point is reached. The purposes of these experiments are to increase knowledge of the process under transient conditions and to generate a set of data for assessing the validity of dynamic process models at the plant scale. It is desirable that the transient data represents the main dynamics of the plant. Table 2 includes the test matrix for the set-point change experiments conducted during the autumn 2015 MEA test campaign at TCM DA. The experiments consist of set-point changes in main inputs to the pilot plant, i.e., rich solvent flow rate, flue gas volumetric flow rate into the absorber and steam flow rate to reboiler. In this paper, tests 2 and 3 are presented for the purpose of dynamic process model validation. These two tests represent set point step-changes in flue gas volumetric flow rate fed to the absorber.
The responses and performance of the pilot plant was logged. The data was extracted every 30 seconds in order to reduce the data load. Logged data includes:
- Gas analyzers at the inlet of the absorber, outlet of the absorber, and CO2 rich to stack.
- Main liquid and gas flow rates.
- Main process temperatures, including absorber and stripper temperatures.
- Pressures and pressure drops at different components of the plant.
- Online solvent analysis measurements include pH, density and conductivity, at the inlet and outlet of the absorber (lean and rich solvent).
- Liquid hold-ups distribution at different components of the plant.
- Main active controller set-points and tuning parameters.
Solvent samples were taken during steady-state conditions at the inlet and outlet of the absorber for posterior analysis in the lab, in order to obtain the CO2 lean and rich solvent loadings. Actual reboiler duty was estimated based on logged measurement data of steam temperatures, pressures and mass flow rate as indicated in Thimsen et al. [15]. In order to assess the validity of the process model, temperature profiles of the absorber and stripper columns were utilized. Each of the measured temperature points included in the steady-state absorber temperature profiles is the average over time during steady-state conditions, of the averaged 4 temperature measurements of the sensors radially distributed within the absorber column, at the given axial position of the column.
The tests were run with a total inventory of aqueous MEA of about 38.2 m3. For process simulations, it is of importance to understand how the solvent inventory is distributed within the different components of the plant. Therefore, liquid hold-ups at different parts of the plant were registered for the steady-state operating cases.
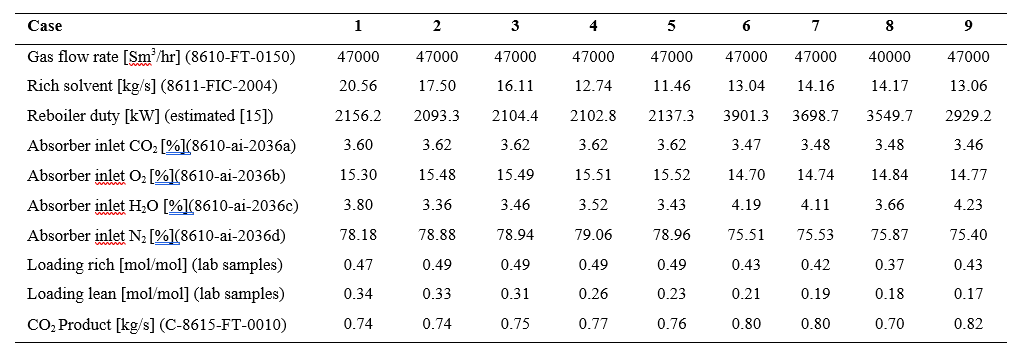
Table 1. Steady-state data for the nine operating cases selected from the MEA test campaign. The plant was operated with 30 wt% aqueous MEA and 24 meters of absorber packing. Note that standard conditions are 15 ºC and 1 atm. The tag IDs for the instrumentation utilized are included.
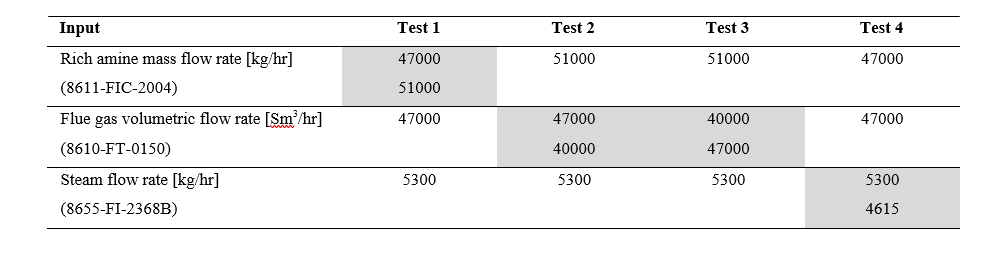
Table 2. Test matrix for the set-point change experiments conducted during the Autumn 2015 MEA test campaign at TCM DA. The tag IDs for the instrumentation used is included.
A dynamic process model of the amine-based TSA plant at TCM DA was built with the open physical modeling language Modelica [11], by means of the commercial modeling and simulation tool Dymola [12]. Modelica allows for component-based modeling, and the component models consist of systems of differential and algebraic equations. The overall plant model consists of models for the absorber and stripper columns, sumps, internal heat exchanger, reboiler, condensers, flow resistances, pumps, valves, measurements and controllers. The process models were obtained from a Modelica library from Modelon AB [16] and have been presented elsewhere [17, 18]. In this work, the component models were configured, parameterized and modified in order to obtain a dynamic process model of the TSA plant at TCM DA considering the main process equipment, size, geometry, material and solvent inventory during the experiments. In addition, the regulatory control layer of the plant was implemented in the process model, considering the control structure at the PCC pilot plant.
Absorption and desorption columns are modeled considering the two-film theory approach, thus thermodynamic equilibrium is assumed at the liquid and gas interface. Packed sections consider rate-based approach for modeling interface mass transfer, with mass transfer coefficients for CO2 and H2O by Onda et al. [19], and enhanced mass transfer due to chemical reactions is implemented via a pseudo-first order enhancement factor [7]. Chemical equilibrium is considered in all model parts, both at interface and liquid bulk, with chemical equilibrium constants obtained empirically from Bötinger [20].
Heats of reaction are inferred from the equilibrium constant via the van’t Hoff equation. Sensible heat transfer between phases is correlated to gas-phase mass transfer coefficient (Cohilton-Colburn analogy), while heat of solution and evaporation is calculated as a function of temperature but is constant with solvent loading. Ideal gas law applies to the gas phase, which is only composed of CO2 , O2, H2O and N2. The pressure of the system p is determined by gas phase pressure drop from a known operating point and a quadratic correlation with gas velocity.
A simplified washer component is included in the head of the column. It is modelled as a simple volume with phase separation. Its purpose is to cool down the gas flow to a temperature given as an input signal and condense as much vapor as required to reach saturation in the gas phase. Water balance is ensured by a make-up water source in the absorber sump that controls the H2O mass balance of the plant. Note that in this model MEA is considered non- volatile, which means that it is only present in the liquid phase. This implies that MEA make-up source is not required in the overall dynamic process model. This is not the case for the real plant, where MEA make-up is required for operation.
The numerical solver DASSL was selected in Dymola for solution of the resulting system of differential and algebraic equations. The process model was exported as a co-simulation Functional Mock-up Unit (FMU) via FMI technology (Functional Mockup Interface) [21]. Simulations and validation were carried out in Microsoft Excel® environment via a FMI-add-in for Excel® [22].
The approach to overall PCC plant model development and validation followed in this work was to initially separate the plant in three main parts: absorber, lean/rich cross heat exchanger and stripper with reboiler. Proper boundary conditions were specified for each part of the process. Steady-state data measured at the pilot plant were used as inputs to the boundary conditions of each section of the process, and the main outputs from the model were compared with the plant data. This involves checking absorption and desorption rates, temperature profiles in the absorber and stripper, and lean and rich CO2 loadings. The task required tuning of uncertain model parameters (tuners) in order to obtain a better agreement between measured plant performance and behavior predicted by the model. Uncertain parameters include enhancement factors and pre-multiplying factors for adjustment of effective interface area correlations. Then, the overall PCC plant process model was closed by connecting the different sections of the process and implementing the suitable regulatory control layer. The main model outputs were compared with measured plant data in steady-state for the overall plant. In the following, the results from the overall plant process model validation are presented.
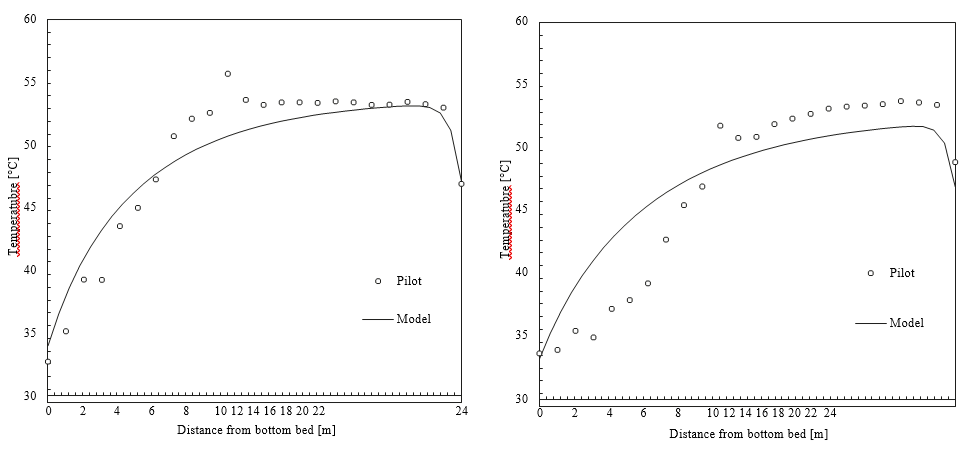
Figure 2. Examples of temperature profiles in absorber and stripper columns during steady-state operating conditions. Left: Case 8. Right: Case 9.
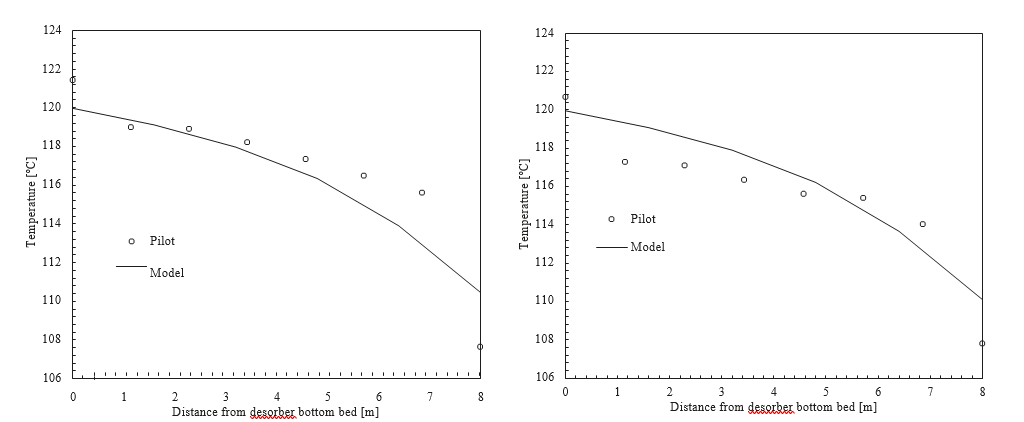
Figure 3. Examples of stripper temperature profiles for two steady-state operating conditions of the PCC pilot plant. Left: Case 8. Right: Case 9 (refer to Table 1).
Examples of temperature profiles within the absorber are shown in Figure 2. Figure 2 (left) shows the absorber temperature profile for the case 8, whereas Figure 2 (right) shows the absorber temperature profile for case 9. Figure 3 (left) shows temperature profiles in the stripper for case 8, whereas Figure 3 (right) shows temperature profiles for stripper in case 9. Note that for cases 8 and 9 the PCC plant is operated with 67 and 80 % flue gas volumetric flow rate capacity in the absorber respectively. Absorber temperature profiles predicted by the model show a good agreement with plant data, especially for case 8. The model is capable to predict properly the trends in temperature along the column. An over prediction is observed in case 9, at the bottom packing below the temperature bulge, while an under prediction is observed from the temperature bulge, within the middle and upper packing. The stripper temperature profile predicted by the process model shows also good agreement with plant data, as illustrated with steady-state cases in Figure 3.
Figure 4 shows the parity plot for lean and rich solvent loadings for the nine steady-state operating cases. It can be observed that the model over predicts the lean and rich loading when compared with the experimental data. The mean percentage error for lean loading is 1.4% and for rich loading 6.7%. There are two steady-state cases where the model shows an under prediction of lean solvent loading. This could be explained by the fact that these two steady-state cases are obtained prior to the injection of anti-foam solution in the plant (cases 6 and 7). Anti-foam is periodically used during MEA test campaigns at TCM to tackle the unideal phenomena in the stripper, and has a direct impact in the performance of the stripper [13]. From the results shown in Figure 4 it can be concluded that the dynamic process model is capable to predict the variability in solvent loading for the steady-state operating cases. The CO2 product flow is under predicted with an average percentage error of 5% for the simulated cases.
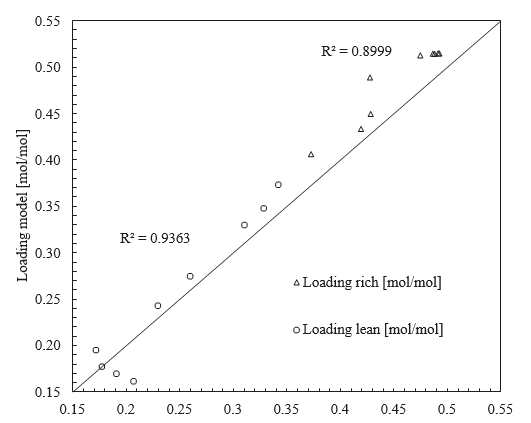
Figure 4. Lean and rich amine CO2 loading parity plots. Model results from overall pilot plant model for nine steady-state operating cases. The mean percentage error for lean loading is 1.4% and for rich loading is 6.7%.
Once the steady-state estimation performance of the dynamic process model is validated for the full plant model, the dynamic process model is validated with transient plant data. Transient performance of this process is characterized by long dead times and large lag times in main process variables, resulting in relatively large total stabilization times. This means that this process is considered slow, when it is compared with a change in load in the steam cycle of a power plant. During the test campaign four transient tests were conducted, here two of them involving flue gas volumetric flow rate ramp-down and ramp-up will be presented.
The test consisted of set-point reduction of the exhaust gas volumetric flow rate fed to the absorber, from 80% to 67% of the plant capacity, i.e. 47000 Sm3/hr to 40000 Sm3/hr. The purpose was to change the flue gas volumetric flow rate while keeping the rest of the plant process variables constant. Figure 5 shows the three main inputs of the plant for this test. The main controlled drifting variables of the plant during the test were kept constant by the action of the controllers of the regulatory control layer of the plant.
The plant was disturbed by manipulating the speed of the induced draft blower located upstream the direct contact cooler. The blower speed was changed in order to set the flue gas volumetric flow rate at the inlet of the absorber. Step set-point reduction in flue gas volumetric flow rate was applied. As shown in Figure 5, this has resulted in an oscillatory flue gas volumetric flow rate as disturbance to the plant, due to the fact that the blower speed/volumetric flue gas flow rate controller is a PI controller. Steam mass flow rate was maintained constant, while the solvent mass flow rate had small amplitude oscillations around the set-point. In order to compare the transient plant data with the actual plant data, the measured flue gas volumetric flow rate was introduced as an input trajectory to the dynamic process model. This means that the same disturbance applied to the plant during the test campaign, was applied to the dynamic process model for simulation. In addition, averaged value of the time series of the measured rich solvent mass flow rate and the estimated reboiler duty was applied as input to the dynamic process model.
Figure 6 shows the response on CO2 product flow rate to the plant input. It was observed an input/output dead time of 40 minutes between flue gas volumetric flow rate and CO2 product mass flow rate. This means that for a change in the flue gas flow rate input to the plant, no changes are observed in the product CO2 flow until around 40 minutes later. Therefore, the system acts as a buffer to load change driven by flue gas volumetric flow rate change at absorber inlet. In addition, it takes around 4 hours to reach the new steady-state operating point. In addition, a significant lag time was found in stabilization of temperature profiles in the absorber (1 hour) and stripper columns (3-4 hours), not shown. It can be observed in Figures 6 and 7 that the process model is capable of predicting the main process dynamics for CO2 product mass flow rate and rich and lean solvent CO2 loadings.
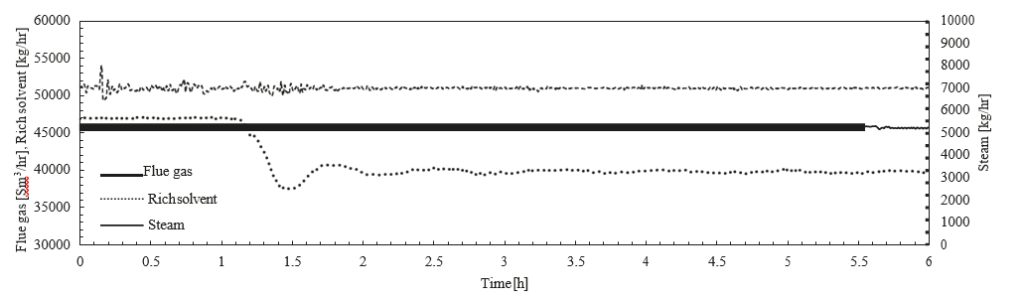
Figure 5. Main inputs to the plant for test with flue gas volumetric flow rate set-point reduction from 47000 [Sm3/hr] to 40000 Sm3/hr. Rich solvent flow rate from absorber kg/hr and steam flow to reboiler kg/hr.
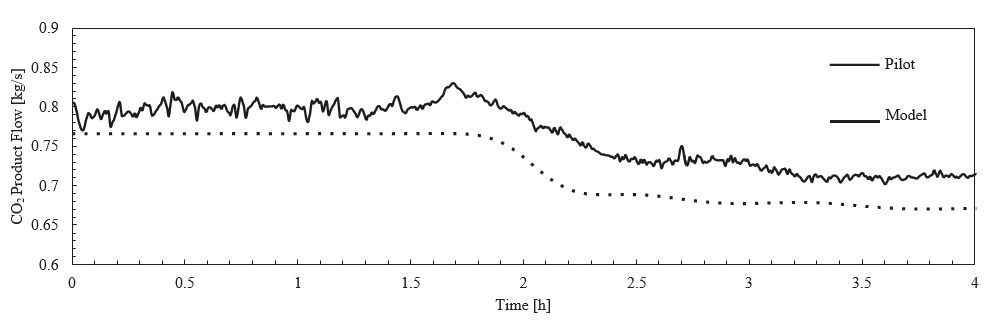
Figure 6. CO2 Product flow rate kg/s.
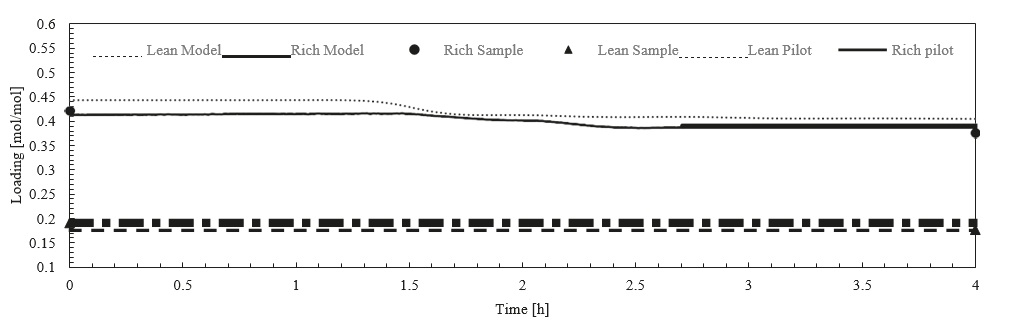
Figure 7. CO2 lean and rich solvent loadings during test 2 (refer to table 2). Lean and rich pilot curves are based on a correlation for total alkalinity, density and temperature of the solvent, measured online at the plant. Lab samples were taken before and after the test.
This test consist of set-point increase of the flue gas volumetric flow rate fed to the absorber, from 67% to 80% of the plant capacity, i.e. 40000 Sm3/hr to 47000 Sm3/hr. Figure 8 shows the three main inputs of the plant during the test. As in the previous test, an oscillatory behavior of the flue gas volumetric flow rate around the new set point is observed. The same approach with the measured input to the plant as input trajectory to the dynamic process model was applied. The plant acts as a buffer for flue gas volumetric flow rate changes as shown in Figure 9. Around 20 minutes dead time input/output from flue gas volumetric flow rate to CO2 product mass flow rate was observed. Figure 9 shows the CO2 product flow for the model and the pilot plant data and Figure 10 shows the plant and model response for this disturbance in terms of CO2 lean and rich solvent loadings. A mismatch of 15 min for CO2 product flow rate predicted by the process model is observed. A similar offset as in the previous test is observed, with a steady- state under prediction of CO2 product flow rate. Despite of the steady-state offset shown on solvent CO2 loadings prediction, it is observed a good prediction of the main dynamics, refer to Figure 10. It can be concluded that the process dynamics are well captured by the process model.
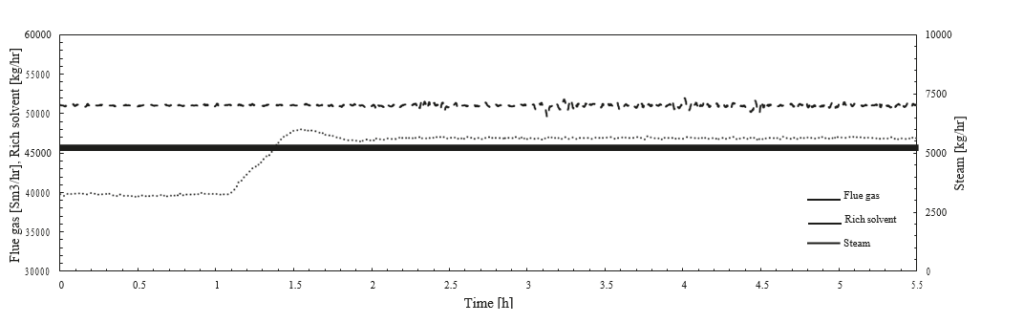
Figure 8. Main inputs to the plant. Flue gas volumetric flow rate set-point change from 47000 [Sm3/hr] to 40000 Sm3/hr. Rich solvent flow rate from absorber kg/hr and steam flow to reboiler kg/hr.
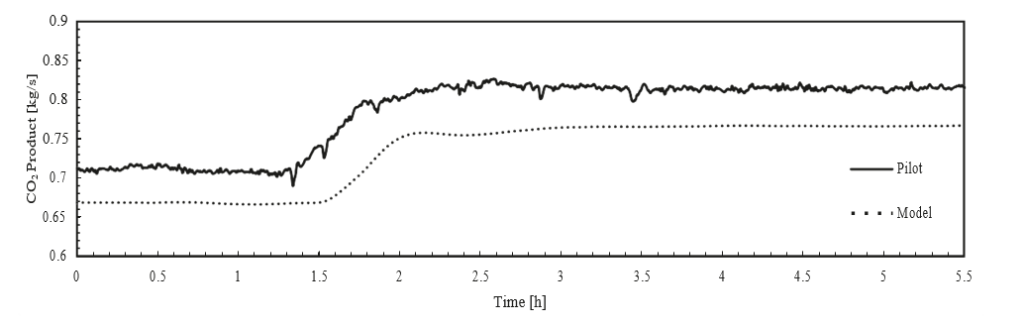
Figure 9. CO2 product kg/hr. Results from test 3 on flue gas volumetric flow rate set-point increase (refer to Table 2).
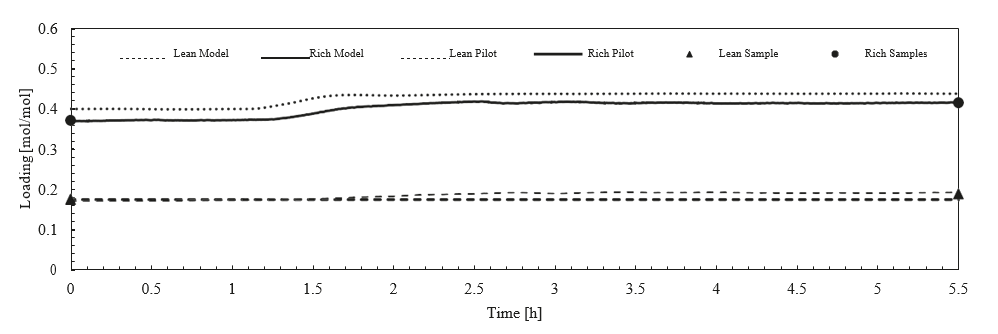
Figure 10. CO2 lean and rich solvent loadings during test 3 (refer to Table 2). Loading pilot curves are based on a correlation for total alkalinity, density and temperature of the solvent, measured at the plant. Lab samples were taken before and after the test.
The task of dynamic process model validation of the post-combustion CO2 absorption with aqueous MEA requires the generation of suitable data sets including both steady-state and transient data. Ideally, the steady-state data should reflect a wide range of operating conditions of the PCC plant. The steady-state data utilized in this work consists of a wide range of operating conditions achieved by changing rich solvent mass flow rate and reboiler heat duty. As shown in Table 1, nine steady-state cases were gathered from the MEA test campaign. The cases include operation of the PCC plant with mass based L/G ratios on the absorber ranging from 1.34 to 0.75, when operating the absorber at 80% capacity and with a capture rate of 85% (cases 1 to 5).
During campaigns at TCM with 30% aqueous MEA, unideal behaviour occurs in the stripper bed and it is handled by addition of anti-foam solution. As shown in literature [13], the addition of anti-foam solution has a significant effect on stripper temperature profile at TCM DA pilot plant for CHP, and especially on specific reboiler duty at low lean amine loadings. Cases 6 and 7 were run before the addition of the anti-foam solution and it has been shown in Figure 4 that the model prediction under estimates lean loading only for these two specific cases. It is advised to check if anti-foam solution was used during the tests, if the data is to be used for process model validation. Anti-foam was introduced in the plant between the transient tests presented in this paper. If required, sufficient time between the tests should be allowed so that steady-state conditions are reached before and after adding anti-foam solution.
The post-combustion TSA process design with solvent recirculation from the stripper to the absorber in a closed- loop makes modeling and validation of the full plant challenging. Modeling errors and inaccuracies in one component of the plant will easily propagate towards other parts of the process. Therefore, a systematic approach is recommended beginning with validation of the separate models of absorber, stripper with reboiler, and heat exchanger sections. In this work, the overall process model is finally developed by joining the different sections and validated with the steady- state and transient pilot data. The intended application of the process model is for transient estimation and plantwide control studies.
Column temperature profiles accurate prediction is of importance since temperature affects phase equilibrium calculation at the gas-liquid interface and liquid phase. In addition, several model parameters and thermophysical properties depend on temperature. These include heat capacity, CO2 solubility, water heat of condensation, heats of reaction and equilibrium constants. The pilot plant absorber and stripper columns temperature profiles are calculated as an averaged value of the temperature measurements from the sensors distributed in the radial plane at the given axial position of the column. The individual temperature measurements are considered reliable, and the resulting temperature profiles are clear and reasonable. Nevertheless, it should be mentioned that some sensors are closer to the wall while some are closer to the center of the packing, thus a small maximum variation (<6 ºC) is observed between the measurements at a given radial position. The variation is different for different operating conditions of the columns and radial planes. The aggregated effect of above-mentioned aspects makes validation of the absorber temperature profiles challenging. Based on the results presented in Figures 2 and 3, it is considered that the dynamic process model is capable of predicting temperature profiles of both absorber and stripper columns with good accuracy for the purpose considered in this work. Tuning of the pre-multiplying factor of the mass transfer enhancement factor has been required (0.2 in absorber and 0.09 in stripper).
Lean and rich CO2 loadings are over-predicted by the dynamic process model. Lean and rich loadings are dependent of each other, and modeling errors will easily propagate. In addition, actual reboiler heat duty has been estimated from steam measurements in the plant as suggested by Thimsen et al. [15]. Nevertheless, that value is not truly representative of regeneration energy due to external factors such as changes in ambient conditions and heat loses through non-insulated pipes and equipment [5]. An under-prediction of lean loading is found on cases 6 and 7. It is believed that this is because the plant was operated before addition of anti-foam solution during these cases as well as due to small deviations on MEA concentration from 30 wt% during that period. The mean percentage error for lean loading is 1.4 % and for rich loading 6.7%. It can be concluded that the process model is capable of predicting the variability in lean and rich loading for the range of operating conditions of the PCC plant. The process model under- predicts CO2 product mass flow rate within <5% for all steady-state cases, being the precision uncertainty of the product CO2 flow measurement 1% (Vortex FT-0010) [13]. This under prediction is illustrated in the transient cases (Figures 6 and 9).
Dynamic process validation with the two tests involving volumetric flue gas flow rate reduction and increase has been presented in this paper. The experiment shows that the system acts as a buffer to load change driven by flue gas volumetric flow rate change at absorber inlet, and long dead times input/output in terms of CO2 product flow are observed (around 20-40 minutes). The results from the model show that the model development has been successful to predict the main process dynamics. This includes CO2 lean and rich loadings and CO2 product flow response to the disturbances.
A dynamic process model of the overall amine-based TSA plant at TCM DA was built for the purpose of model validation with a new set of steady-state and transient plant data. It is concluded that the dynamic process model is capable of estimating the temperature profiles of absorber and stripper columns with good accuracy for the purpose of application. Tuning of the pre-multiplying factor for calibration of the enhancement factor has been required. An over prediction of the model for lean and rich CO2 loadings has been reported, being mean percentage errors <1.5% and <6.7%. The process model is capable of predicting the variability of lean and rich loadings for a wide range of steady-state operating conditions. In addition, an under prediction of CO2 product flow rate has been observed (<5%). The main process dynamics of the pilot plant under flue gas volumetric flow changes is captured by the process model. The validated process model developed in this work will be used to analyze the TCM plant transient performance and expanded to a full-scale plant model to predict transient performance of a natural gas combined cycle power plant integrated with post-combustion CO2 capture.
The authors acknowledge TCM DA and the Department of Energy and Process Engineering at NTNU-Norwegian University of Science and Technology for funding this project. The Faculty of Engineering Science NTNU-Statoil Publication Grant 2016 is also acknowledged. The authors want to acknowledge Espen S. Hamborg from TCM DA for his contribution to establish the ongoing collaboration between TCM DA and the Thermal Energy Research Group at the Department of Energy and Process Engineering at NTNU.
- R.M. Montañés, M. Korpås, L.O. Nord, S. Jaehnert, Identifying Operational Requirements for Flexible CCS Power Plant in Future Energy Systems, Energy Procedia, 86 (2016) 22-31.
- M.E. Boot-Handford, J.C. Abanades, E.J. Anthony, M.J. Blunt, S. Brandani, N. Mac Dowell, J.R. Fernandez, M.-C. Ferrari, R. Gross, J.P. Hallett, R.S. Haszeldine, P. Heptonstall, A. Lyngfelt, Z. Makuch, E. Mangano, R.T.J. Porter, M. Pourkashanian, G.T. Rochelle, N. Shah, J.G. Yao, P.S. Fennell, Carbon capture and storage update, Energy & Environmental Science, 7 (2014) 130-189.
- R. Faber, M. Köpcke, O. Biede, J.N. Knudsen, J. Andersen, Open-loop step responses for the MEA post-combustion capture process: Experimental results from the Esbjerg pilot plant, Energy Procedia, 4 (2011) 1427-1434.
- P. Tait, B. Buschle, I. Ausner, P. Valluri, M. Wehrli, M. Lucquiaud, A pilot-scale study of dynamic response scenarios for the flexible operation of post-combustion CO2 capture, International Journal of Greenhouse Gas Control.
- M. Bui, I. Gunawan, V. Verheyen, P. Feron, E. Meuleman, Flexible operation of CSIRO’s post-combustion CO2 capture pilot plant at the AGL Loy Yang power station, International Journal of Greenhouse Gas Control.
- N. Enaasen Flø, H. Knuutila, H.M. Kvamsdal, M. Hillestad, Dynamic model validation of the post-combustion CO2 absorption process, International Journal of Greenhouse Gas Control, 41 (2015) 127-141.
- H.M. Kvamsdal, J.P. Jakobsen, K.A. Hoff, Dynamic modeling and simulation of a CO2 absorber column for post-combustion CO2 capture, Chemical Engineering and Processing: Process Intensification, 48 (2009) 135-144.
- C. Biliyok, A. Lawal, M. Wang, F. Seibert, Dynamic modelling, validation and analysis of post-combustion chemical absorption CO2 capture plant, International Journal of Greenhouse Gas Control, 9 (2012) 428-445.
- J. Gaspar, A.-M. Cormos, Dynamic modeling and absorption capacity assessment of CO2 capture process, International Journal of Greenhouse Gas Control, 8 (2012) 45-55.
- M. Bui, I. Gunawan, V. Verheyen, P. Feron, E. Meuleman, S. Adeloju, Dynamic modelling and optimisation of flexible operation in post- combustion CO2 capture plants—A review, Computers & Chemical Engineering, 61 (2014) 245-265.
- Modelica Association, https://www.modelica.org/.
- Dassault Systems, Dymola, http://www.3ds.com/products-services/catia/products/dymola.
- N. Brigman, M.I. Shah, O. Falk-Pedersen, T. Cents, V. Smith, T. De Cazenove, A.K. Morken, O.A. Hvidsten, M. Chhaganlal, J.K. Feste, G. Lombardo, O.M. Bade, J. Knudsen, S.C. Subramoney, B.F. Fostås, G. de Koeijer, E.S. Hamborg, Results of Amine Plant Operations from 30 wt% and 40 wt% Aqueous MEA Testing at the CO2 Technology Centre Mongstad, Energy Procedia, 63 (2014) 6012-6022.
- E.S. Hamborg, V. Smith, T. Cents, N. Brigman, O.F. Pedersen, T. De Cazenove, M. Chhaganlal, J.K. Feste, Ø. Ullestad, H. Ulvatn, O. Gorset, I. Askestad, L.K. Gram, B.F. Fostås, M.I. Shah, A. Maxson, D. Thimsen, Results from MEA testing at the CO2 Technology Centre Mongstad. Part II: Verification of baseline results, Energy Procedia, 63 (2014) 5994-6011.
- D. Thimsen, A. Maxson, V. Smith, T. Cents, O. Falk-Pedersen, O. Gorset, E.S. Hamborg, Results from MEA testing at the CO2 Technology Centre Mongstad. Part I: Post-Combustion CO2 capture testing methodology, Energy Procedia, 63 (2014) 5938-5958.
- Modelon, Post-combustion capture with amine solutions. http://www.modelon.com/industries/energy-process/carbon-capture-and- sequestration/.
- K. Prölß, H. Tummescheit, S. Velut, J. Åkesson, Dynamic model of a post-combustion absorption unit for use in a non-linear model predictive control scheme, Energy Procedia, 4 (2011) 2620-2627.
- S.Ó. Garðarsdóttir, F. Normann, K. Andersson, K. Prölß, S. Emilsdóttir, F. Johnsson, Post-combustion CO2 capture applied to a state-of-the- art coal-fired power plant—The influence of dynamic process conditions, International Journal of Greenhouse Gas Control, 33 (2015) 51-62.
- K. Onda, H. Takeuchi, Y. Okumoto, Mass transfer coefficients between gas and liquid phases in packed columns, Journal of Chemical Engineering of Japan, 1 (1968) 56-62.
- W. Böttinger, NMR-spektroskopische Untersuchung der Reaktivabsorption von Kohlendioxid in wässrigen Aminlösungen, VDI-Verlag, 2006.
- Functional Mock-up Interface. https://www.fmi-standard.org/.
- Modelon, FMI-Add-In for Excel. http://www.modelon.com/products/fmi-tools/fmi-add-in-for-excel/.